Hybrid Quantum-Classical Algorithms for Loan-Collection Optimization with Loan-Loss Provisions
arxiv(2023)
摘要
Banks are required to set aside funds in their income statement, known as a loan-loss provision (LLP), to account for potential loan defaults and expenses. By treating the LLP as a global constraint, we propose a hybrid quantum-classical algorithm to solve a specific case of quadratic constrained binary optimization (QCBO) models for loan-collection optimization. The objective is to find a set of optimal loan-collection actions that maximizes the expected net profit presented to the bank as well as the financial welfare in the financial network of loanees, while keeping the LLP at its minimum. Our algorithm consists of three parts: a classical divide-and-conquer algorithm to enable a large-scale optimization, a quantum alternating operator ansatz (QAOA) algorithm to maximize the objective function, and a classical sampling algorithm to handle the LLP. We apply the algorithm to a real-world data set with 600 loanees and five possible collection actions. The QAOA is performed using up to 35 qubits on a classical computer. We show that incorporating the QAOA can enhance the expected net profit by approximately 70% in comparison to scenarios where the QAOA is absent from the hybrid algorithm. Although this improvement does not constitute definitive evidence of quantum advantage, our work illustrates the use of near-term quantum devices to tackle real-world optimization problems.
更多查看译文
关键词
algorithms,quantum-classical,loan-collection,loan-loss
AI 理解论文
溯源树
样例
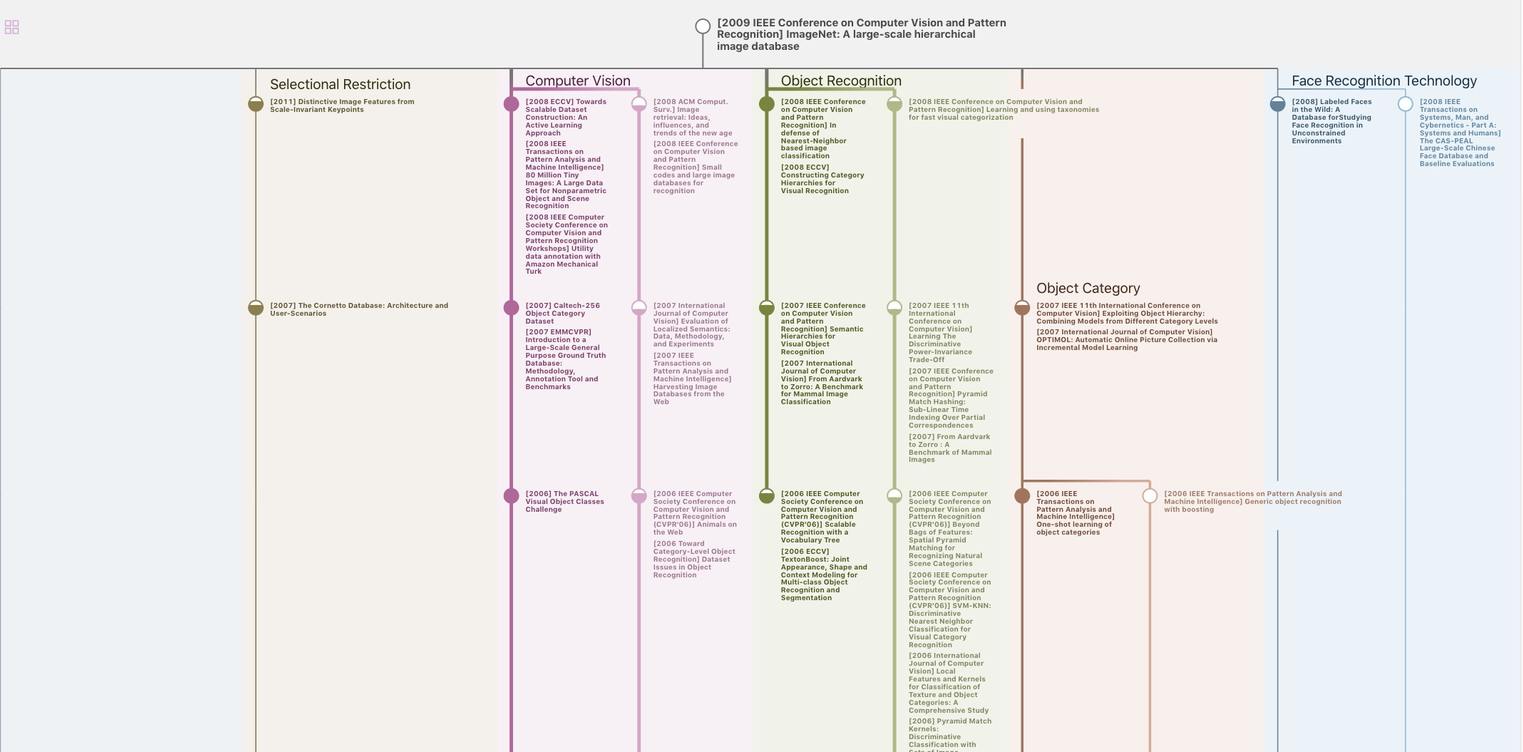
生成溯源树,研究论文发展脉络
Chat Paper
正在生成论文摘要