An Adversarial sample defense method based on multi-scale GAN
INTERNATIONAL JOURNAL OF MACHINE LEARNING AND CYBERNETICS(2021)
摘要
In recent years, the development of deep neural networks is in full gear in the fields of computer vision, natural language processing, and others. However, the existence of adversarial examples brings risks to the completion of these tasks, which is also a huge obstacle to implement deep learning applications in the real world. In order to solve the aforementioned problems and improve the robustness of neural networks, a novel defense network based on generative adversarial networks (GANs) is proposed. First, we use generators to eliminate disturbances of adversarial samples and utilize multi-scale discriminators to classify images of different scales to better assist the generator to produce high-quality images. Then, we utilize salient feature extraction model to extract salient maps of both clean examples and adversarial samples, thus improving the denoising effect of the generator by reducing the difference between salient images. The proposed method can guide the generation networks to accurately remove the invisible disturbance and to restore the adversarial samples to clean samples, which not only improves the success rate of classification, but also achieves satisfactory defense effect. Extensive experiments are conducted to compare the defense effect of our proposed method with other defense methods against various attacks. Results show that our method has strong defensive capabilities against the tested attack methods.
更多查看译文
关键词
Adversarial example, Defense, Deep neural networks, Generative adversarial networks, Multi-scale discriminator
AI 理解论文
溯源树
样例
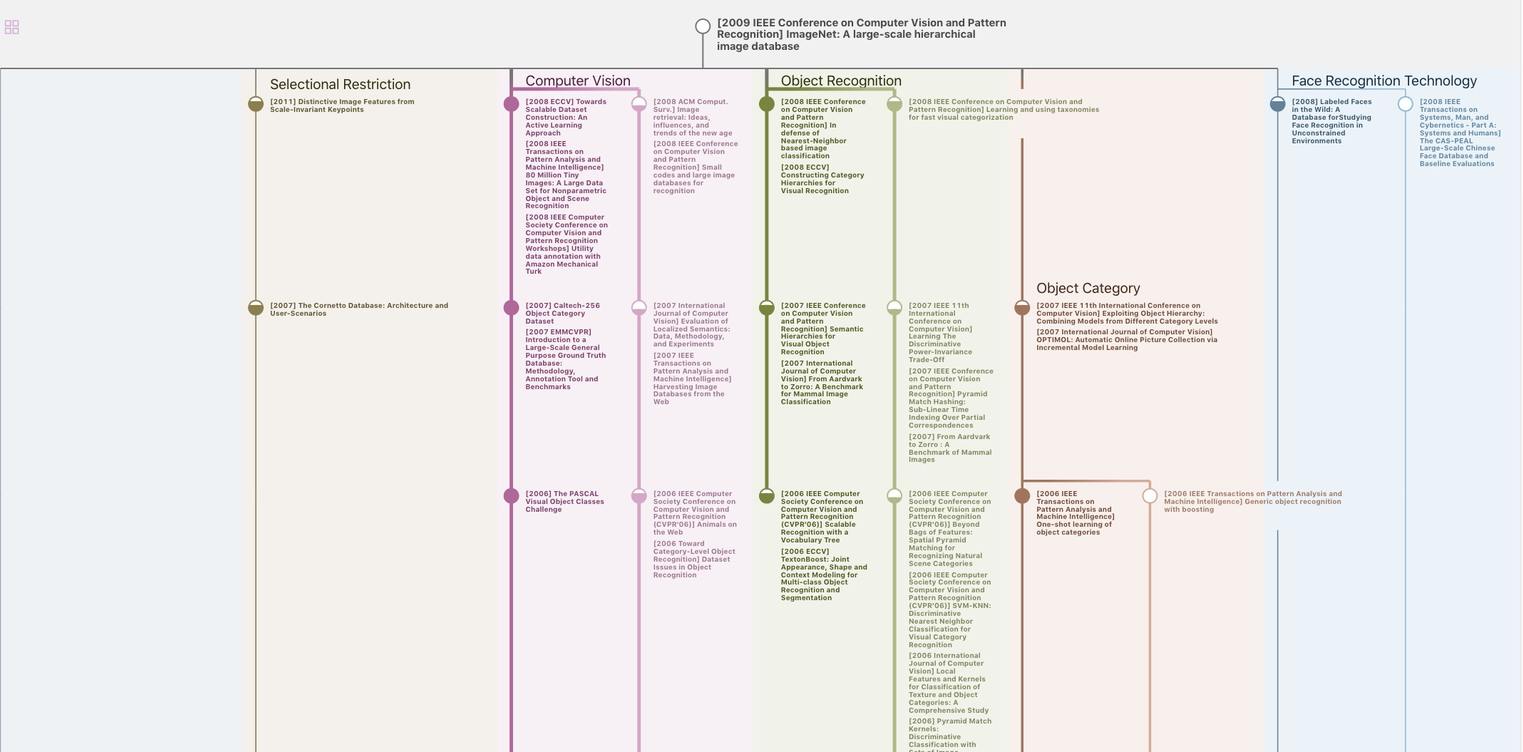
生成溯源树,研究论文发展脉络
Chat Paper
正在生成论文摘要