LF-YOLO: A Lighter and Faster YOLO for Weld Defect Detection of X-Ray Image
arxiv(2023)
摘要
X-ray image plays an important role in manufacturing industry for quality assurance, because it can reflect the internal condition of weld region. However, the shape and scale of different defect types vary greatly, which makes it challenging for model to detect weld defects. In this article, we propose a weld defect detection method based on convolution neural network (CNN), namely, lighter and faster YOLO (LF-YOLO). In particular, a reinforced multiscale feature (RMF) module is designed to implement both parameterbased and parameter-free multiscale information extracting operations. RMF enables the extracted feature map to represent more plentiful information, which is achieved by a superior hierarchical fusion structure. To improve the performance of detection network, we propose an efficient feature extraction (EFE) module. EFE processes input data with extremely low consumption and improves the practicability of whole network in actual industry. Experimental results show that our weld defect detection network achieves satisfactory balance between performance and consumption and reaches 92.9 mean average precision (mAP50) with 61.5 frames/s. To further prove the ability of our method, we test it on the public dataset MS COCO, and the results show that our LF-YOLO has an outstanding versatility detection performance. The code is available at https://github.com/lmomoy/LF-YOLO.
更多查看译文
关键词
Convolutional neural network,lightweight technology,multiscale strategy,weld defect detection,X-ray weld image
AI 理解论文
溯源树
样例
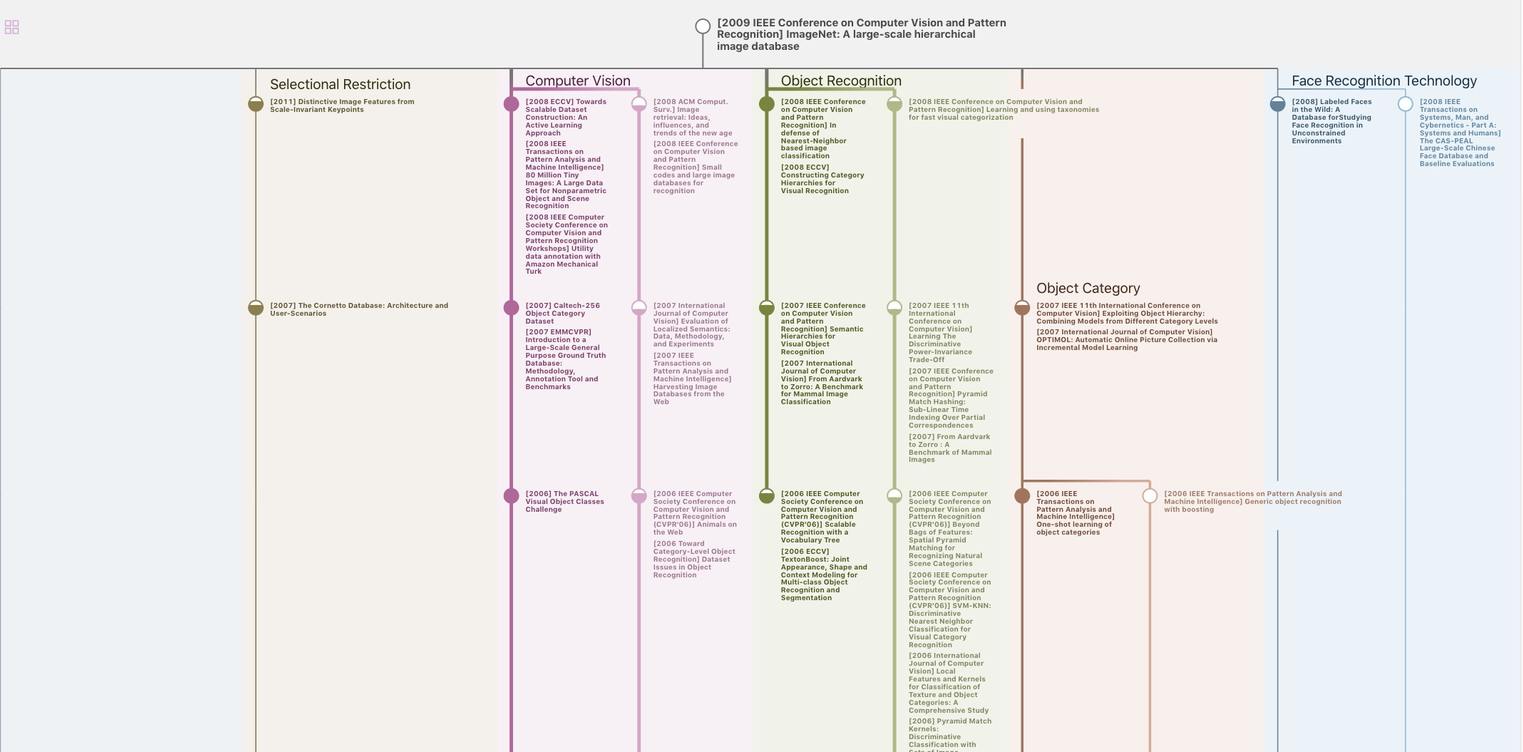
生成溯源树,研究论文发展脉络
Chat Paper
正在生成论文摘要