On the explainability of hospitalization prediction on a large COVID-19 patient dataset.
AMIA ... Annual Symposium proceedings. AMIA Symposium(2021)
摘要
We develop various AI models to predict hospitalization on a large (over 110k) cohort of COVID-19 positive-tested US patients, sourced from March 2020 to February 2021. Models range from Random Forest to Neural Network (NN) and Time Convolutional NN, where combination of the data modalities (tabular and time dependent) are performed at different stages (early vs. model fusion). Despite high data unbalance, the models reach average precision 0.96-0.98 (0.75-0.85), recall 0.96-0.98 (0.74-0.85), and F-score 0.97-0.98 (0.79-0.83) on the non-hospitalized (or hospitalized) class. Performances do not significantly drop even when selected lists of features are removed to study model adaptability to different scenarios. However, a systematic study of the SHAP feature importance values for the developed models in the different scenarios shows a large variability across models and use cases. This calls for even more complete studies on several explainability methods before their adoption in high-stakes scenarios.
更多查看译文
关键词
hospitalization prediction,explainability,patient
AI 理解论文
溯源树
样例
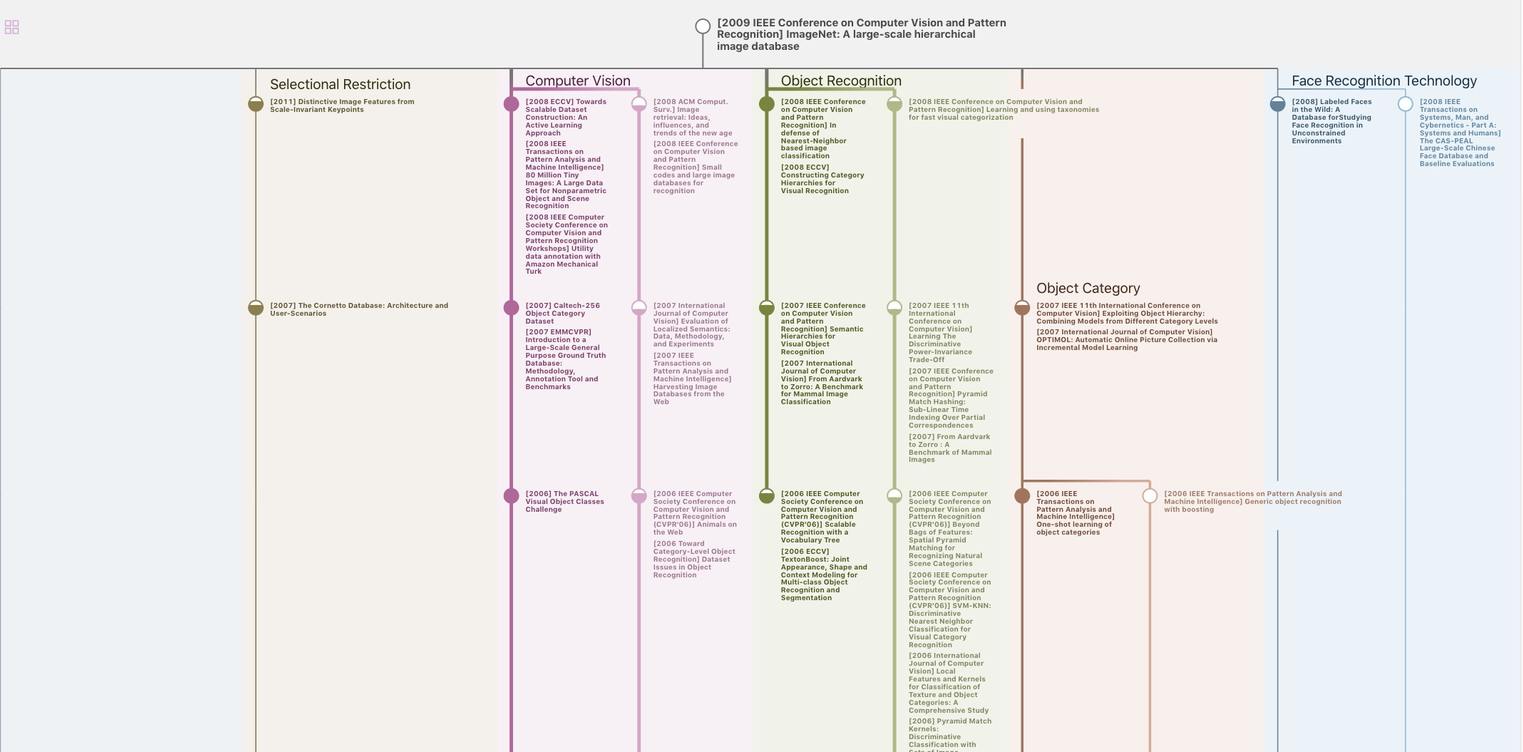
生成溯源树,研究论文发展脉络
Chat Paper
正在生成论文摘要