Counterbalancing Learning and Strategic Incentives in Allocation Markets
arxiv(2021)
摘要
This paper considers the problem of offering a scarce object with a common
unobserved quality to strategic agents in a priority queue. Each agent has a
private signal over the quality of the object and observes the decisions made
by other agents. We first show that, under the widely-used
first-come-first-served sequential offering mechanism, herding behavior
emerges: initial rejections create an information cascade resulting in
inefficient waste. To address this issue, we then introduce a class of batching
mechanisms. Agents in each batch report whether they would be willing to accept
or reject the object based on their private signals and prior information. If
the majority opts to accept, the object is randomly allocated within that
batch. We prove that suitable batching mechanisms are incentive-compatible and
improve efficiency. A key property of the mechanism is the gradual increase of
the batch size after each failed allocation; the size is chosen so that it
elicits as much information as possible without distorting the incentives of
agents to report truthfully. Additionally, from a healthcare policy
perspective, our results can shed light on the large wastage in organ
allocation. In particular, wastage that arises due to herding may be reduced by
applying adaptive simultaneous offering mechanisms.
更多查看译文
AI 理解论文
溯源树
样例
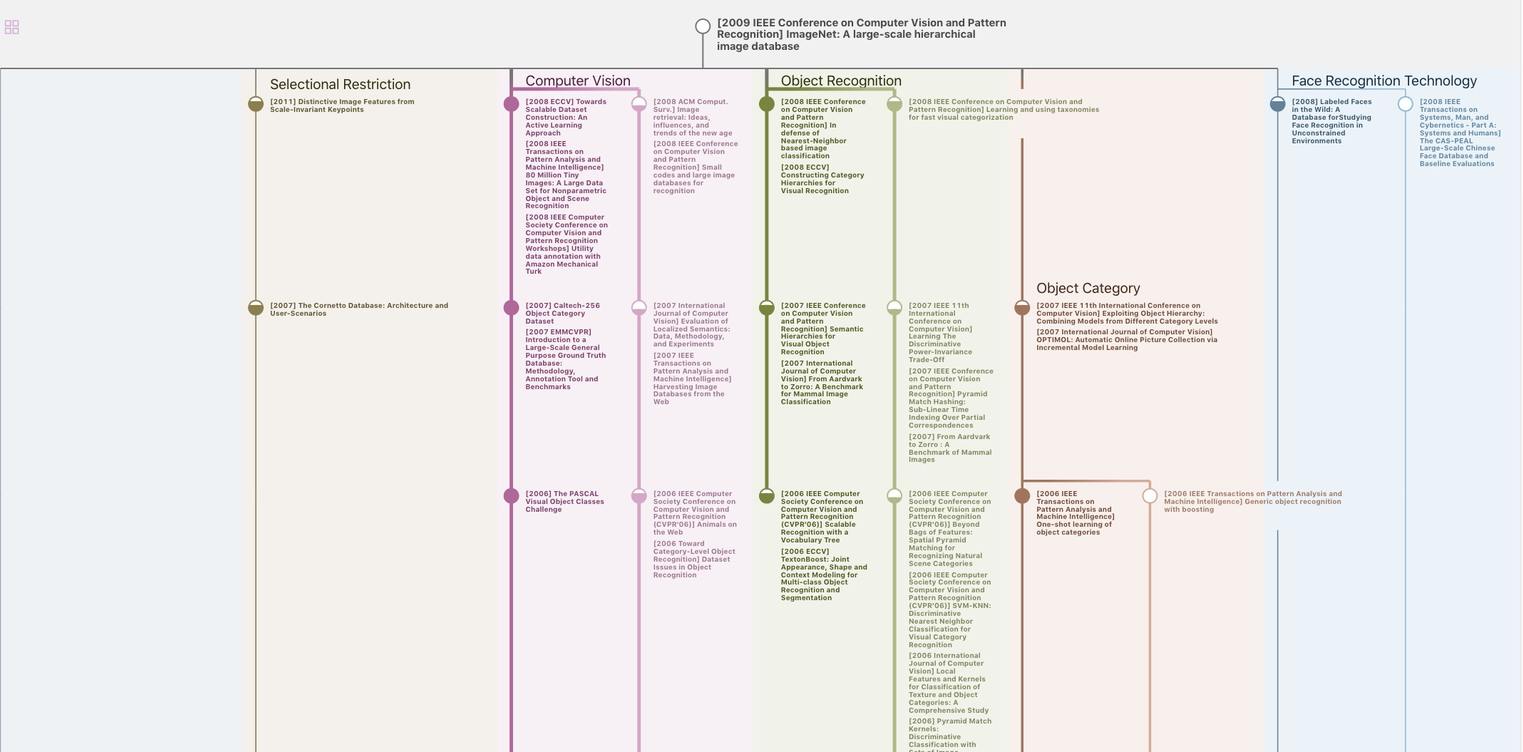
生成溯源树,研究论文发展脉络
Chat Paper
正在生成论文摘要