Revisiting the Performance of iALS on Item Recommendation Benchmarks
ACM Conference On Recommender Systems(2022)
摘要
BSTRACT Matrix factorization learned by implicit alternating least squares (iALS) is a popular baseline in recommender system research publications. iALS is known to be one of the most computationally efficient and scalable collaborative filtering methods. However, recent studies suggest that its prediction quality is not competitive with the current state of the art, in particular autoencoders and other item-based collaborative filtering methods. In this work, we revisit four well-studied benchmarks where iALS was reported to perform poorly and show that with proper tuning, iALS is highly competitive and outperforms any method on at least half of the comparisons. We hope that these high quality results together with iALS’s known scalability spark new interest in applying and further improving this decade old technique.
更多查看译文
关键词
item recommendation benchmarks,ials,performance
AI 理解论文
溯源树
样例
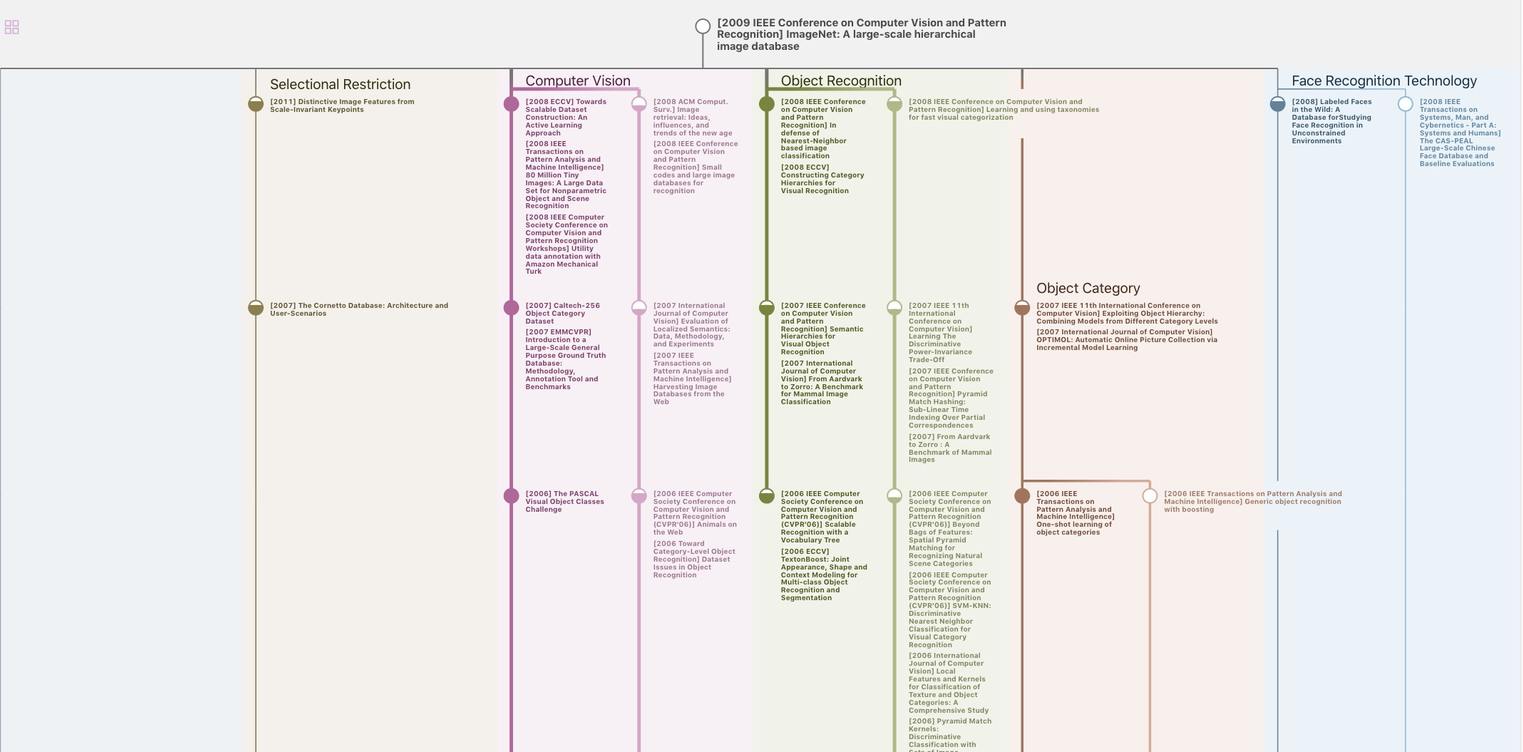
生成溯源树,研究论文发展脉络
Chat Paper
正在生成论文摘要