Learning Tactile Models for Factor Graph-based Estimation
2021 IEEE INTERNATIONAL CONFERENCE ON ROBOTICS AND AUTOMATION (ICRA 2021)(2021)
摘要
We're interested in the problem of estimating object states from touch during manipulation under occlusions. In this work, we address the problem of estimating object poses from touch during planar pushing. Vision-based tactile sensors provide rich, local image measurements at the point of contact. A single such measurement, however, contains limited information and multiple measurements are needed to infer latent object state. We solve this inference problem using a factor graph. In order to incorporate tactile measurements in the graph, we need local observation models that can map high-dimensional tactile images onto a low-dimensional state space. Prior work has used low-dimensional force measurements or engineered functions to interpret tactile measurements. These methods, however, can be brittle and difficult to scale across objects and sensors. Our key insight is to directly learn tactile observation models that predict the relative pose of the sensor given a pair of tactile images. These relative poses can then be incorporated as factors within a factor graph. We propose a two-stage approach: first we learn local tactile observation models supervised with ground truth data, and then integrate these models along with physics and geometric factors within a factor graph optimizer. We demonstrate reliable object tracking using only tactile feedback for similar to 150 real-world planar pushing sequences with varying trajectories across three object shapes.
更多查看译文
关键词
tactile models,factor graph-based estimation,vision-based tactile sensors,image measurements,latent object state,inference problem,tactile measurements,local observation models,map highdimensional tactile images,low-dimensional state space,low-dimensional force measurements,local tactile observation models,geometric factors,factor graph optimizer,reliable object tracking,tactile feedback,object shapes
AI 理解论文
溯源树
样例
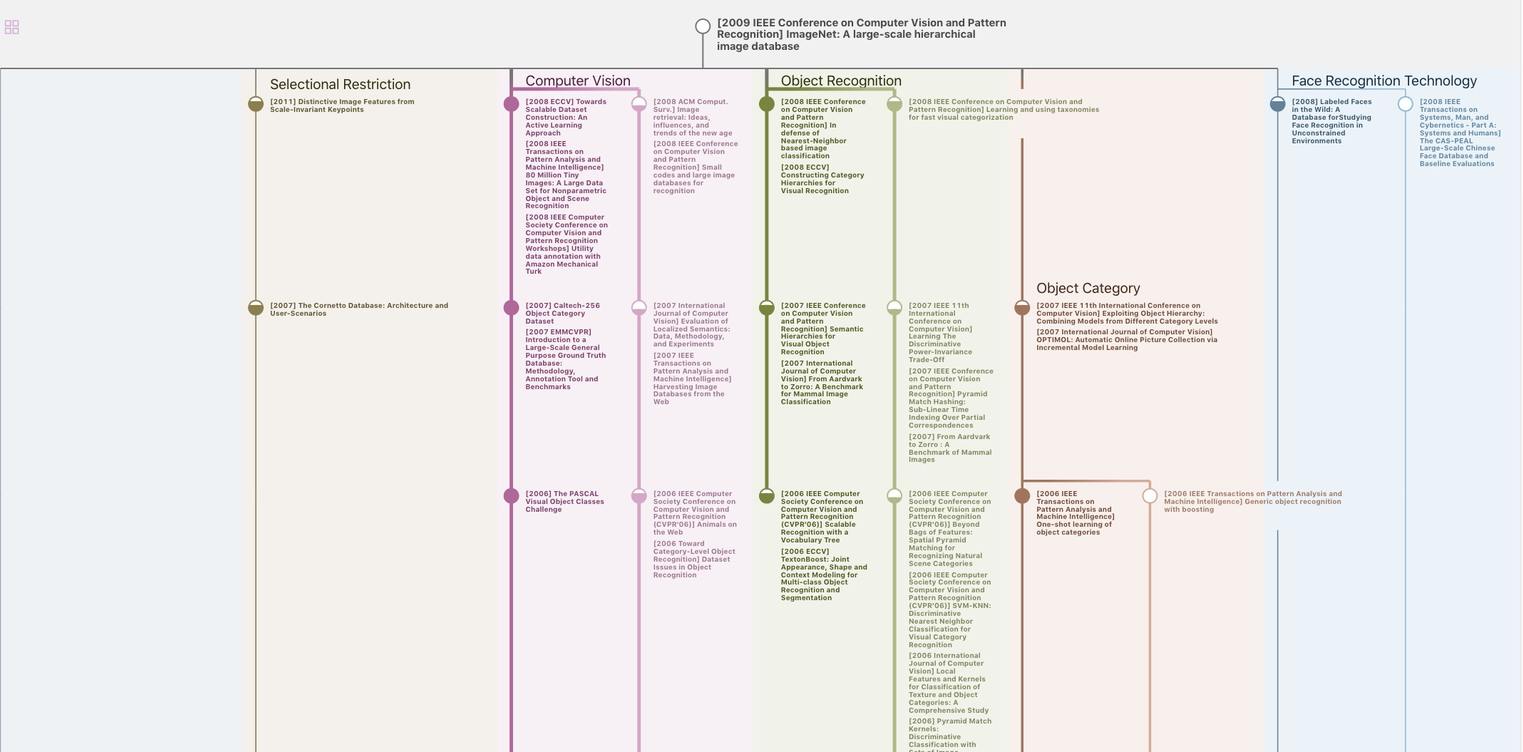
生成溯源树,研究论文发展脉络
Chat Paper
正在生成论文摘要