Path Signature Area-Based Causal Discovery in Coupled Time Series.
Causal Analysis Workshop Series (CAWS)(2021)
摘要
Coupled dynamical systems are frequently observed in nature, but often not well understood in terms of their causal structure without additional domain knowledge about the system. Especially when analyzing observational time series data of dynamical systems where it is not possible to conduct controlled experiments, for example time series of climate variables, it can be challenging to determine how features causally influence each other. There are many techniques available to recover causal relationships from data, such as Granger causality, convergent cross mapping, and causal graph structure learning approaches such as PCMCI. Path signatures and their associated signed areas provide a new way to approach the analysis of causally linked dynamical systems, particularly in informing a model-free, data-driven approach to algorithmic causal discovery. With this paper, we explore the use of path signatures in causal discovery and propose the application of confidence sequences to analyze the significance of the magnitude of the signed area between two variables. These confidence sequence regions converge with greater sampling length, and in conjunction with analyzing pairwise signed areas across time-shifted versions of the time series, can help identify the presence of lag/lead causal relationships. This approach provides a new way to define the confidence of a causal link existing between two time series, and ultimately may provide a framework for hypothesis testing to define whether one time series causes another
更多查看译文
关键词
causal discovery,coupled time series,time series,area-based
AI 理解论文
溯源树
样例
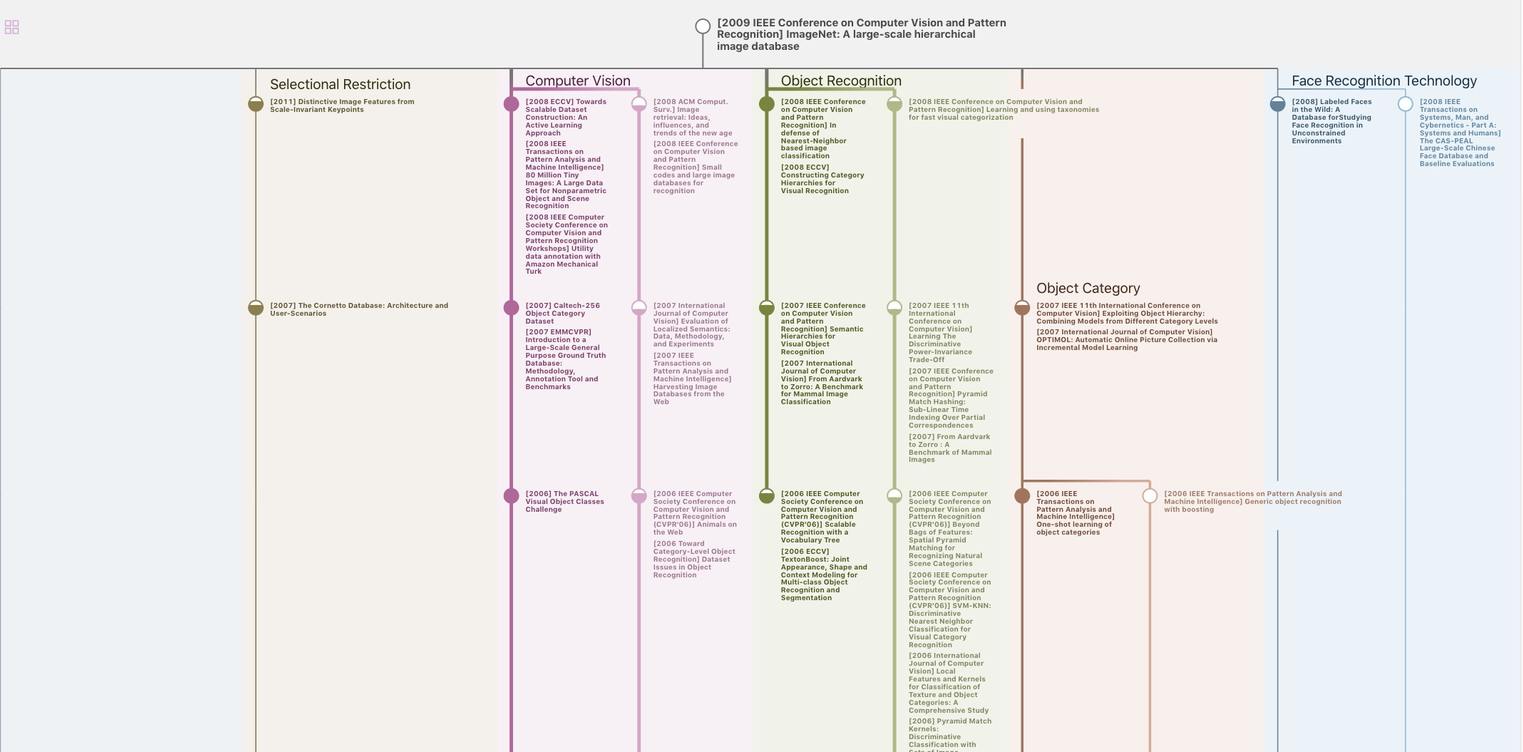
生成溯源树,研究论文发展脉络
Chat Paper
正在生成论文摘要