Federated Learning Over Wireless IoT Networks With Optimized Communication and Resources
IEEE Internet of Things Journal(2022)
摘要
To leverage massive distributed data and computation resources, machine learning in the network edge is considered to be a promising technique, especially for large-scale model training. Federated learning (FL), as a paradigm of collaborative learning techniques, has obtained increasing research attention with the benefits of communication efficiency and improved data privacy. Due to the lossy communication channels and limited communication resources (e.g., bandwidth and power), it is of interest to investigate fast responding and accurate FL schemes over wireless systems. Hence, we investigate the problem of jointly optimized communication efficiency and resources for FL over wireless Internet of Things (IoT) networks. To reduce complexity, we divide the overall optimization problem into two subproblems, i.e., the client scheduling problem and the resource allocation problem. To reduce the communication costs for FL in wireless IoT networks, a new client scheduling policy is proposed by reusing stale local model parameters. To maximize successful information exchange over networks, a Lagrange multiplier method is first leveraged by decoupling variables, including power variables, bandwidth variables, and transmission indicators. Then, a linear-search-based power and bandwidth allocation method is developed. Given appropriate hyperparameters, we show that the proposed communication-efficient FL (CEFL) framework converges at a strong linear rate. Through extensive experiments, it is revealed that the proposed CEFL framework substantially boosts both the communication efficiency and learning performance of both training loss and test accuracy for FL over wireless IoT networks compared to a basic FL approach with uniform resource allocation.
更多查看译文
关键词
Communication efficiency,federated learning (FL),resource allocation,wireless Internet of Things (IoT) networks
AI 理解论文
溯源树
样例
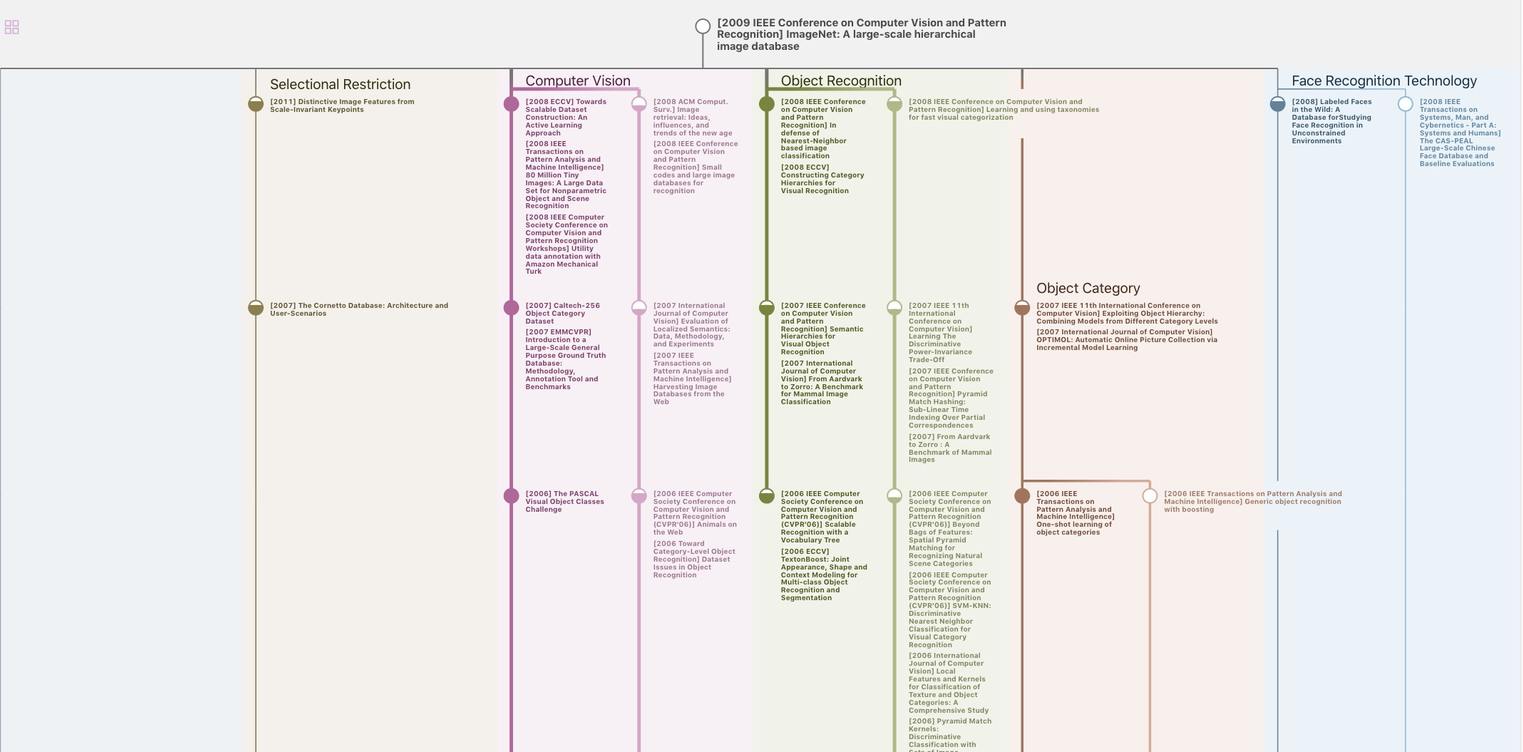
生成溯源树,研究论文发展脉络
Chat Paper
正在生成论文摘要