Image Search with Text Feedback by Deep Hierarchical Attention Mutual Information Maximization
International Multimedia Conference(2021)
摘要
ABSTRACTImage retrieval with text feedback is an emerging research topic with the objective of integrating inputs from multiple modalities as queries. In this paper, queries contain a reference image plus text feedback that describes modifications between this image and the desired image. The existing work for this task mainly focuses on designing a new fusion network to compose the image and text. Still, little research pays attention to the modality gap caused by the inconsistent distribution of features from different modalities, which dramatically influences the feature fusion and similarity learning between queries and the desired image. We propose a Distribution-Aligned Text-based Image Retrieval (DATIR) model, which consists of attention mutual information maximization and hierarchical mutual information maximization, to bridge this gap by increasing non-linear statistic dependencies between representations of different modalities. More specifically, attention mutual information maximization narrows the modality gap between different input modalities by maximizing mutual information between the text representation and its semantically consistent representation captured from the reference image and the desired image by the difference transformer. For hierarchical mutual information maximization, it aligns distributions of features from the image modality and the fusion modality by estimating mutual information between a single-layer representation in the fusion network and the multi-level representations in the desired image encoder. Extensive experiments on three large-scale benchmark datasets demonstrate that we can bridge the modality gap between different modalities and achieve state-of-the-art retrieval performance.
更多查看译文
AI 理解论文
溯源树
样例
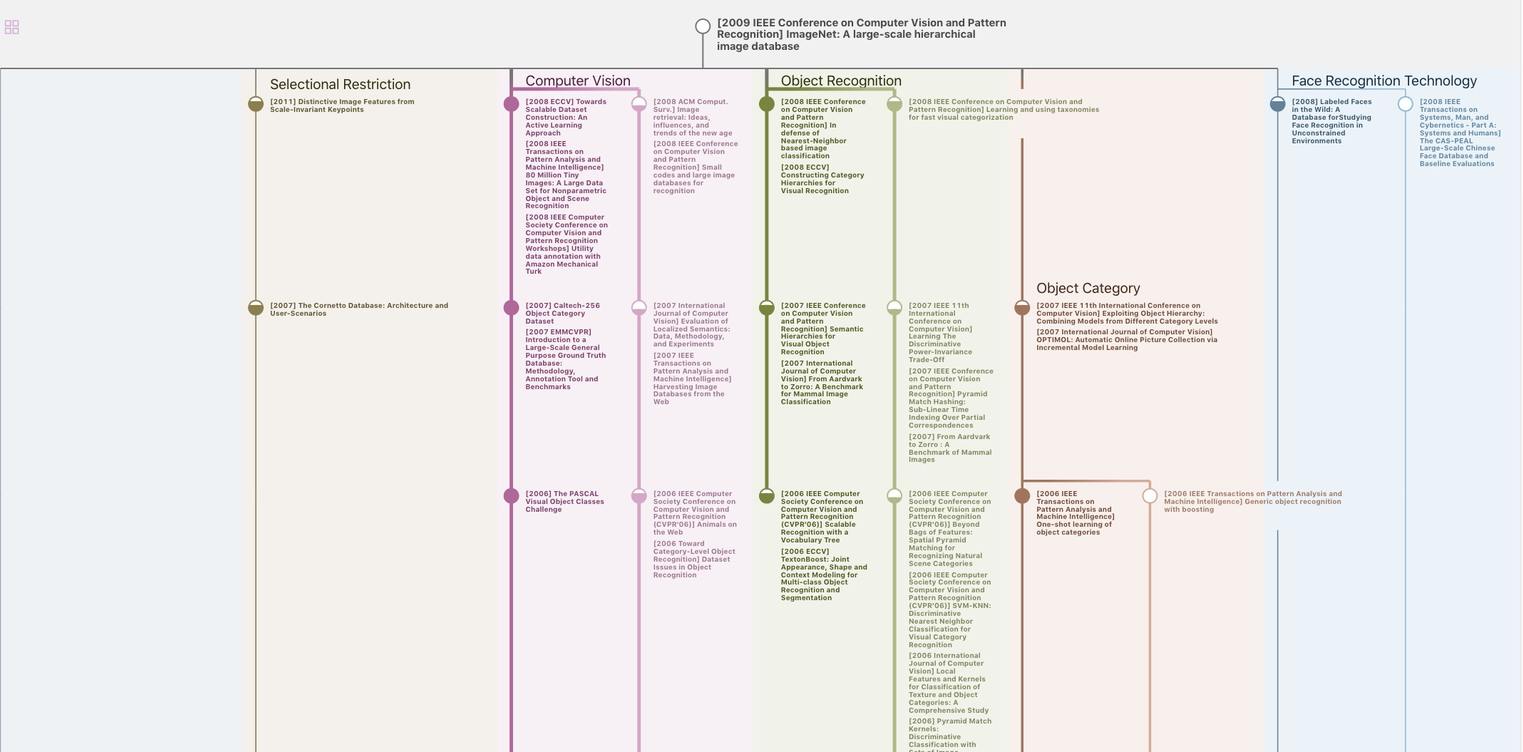
生成溯源树,研究论文发展脉络
Chat Paper
正在生成论文摘要