Genecgan: A Conditional Generative Adversarial Network Based On Genetic Tree For Point Cloud Reconstruction
NEUROCOMPUTING(2021)
摘要
In this paper, we propose an end-to-end conditional generative adversarial model for 3D point clouds reconstruction, called GeneCGAN. Recently, utilizing deep neural networks to generate 3D data has attracted increasing attention in the research community. Generative models of point clouds are used extensively for many tasks ranging from upsampling to shape completion or reconstruction. Our work addresses 3D reconstruction from a latent representation, generating a particular output corresponding to the ground truth point cloud. It is well known that graph convolutional networks (GCNs) require a tremendous amount of computational complexity to compute the graph connectivity dynamically. To reduce this, a simulated genetic (SG) layer is introduced in the generator of the GeneCGAN from a heredity perspective. It performs within a hierarchical rooted tree by leveraging ancestor information and neighbor relationships. Moreover, with a prior fusing operation, global features regarded as the conditional information are added to the root node in a hierarchical rooted tree for learning a conditional probability distribution of the input datasets. It leads to a controllable point cloud output. Experimental results reveal the important fact that the proposed method can achieve a better performance than other existing methods listed in the literature. (c) 2021 Elsevier B.V. All rights reserved.
更多查看译文
关键词
Point clouds, Conditional Generative adversarial nets, (CGANs), Reconstruction, Neural network
AI 理解论文
溯源树
样例
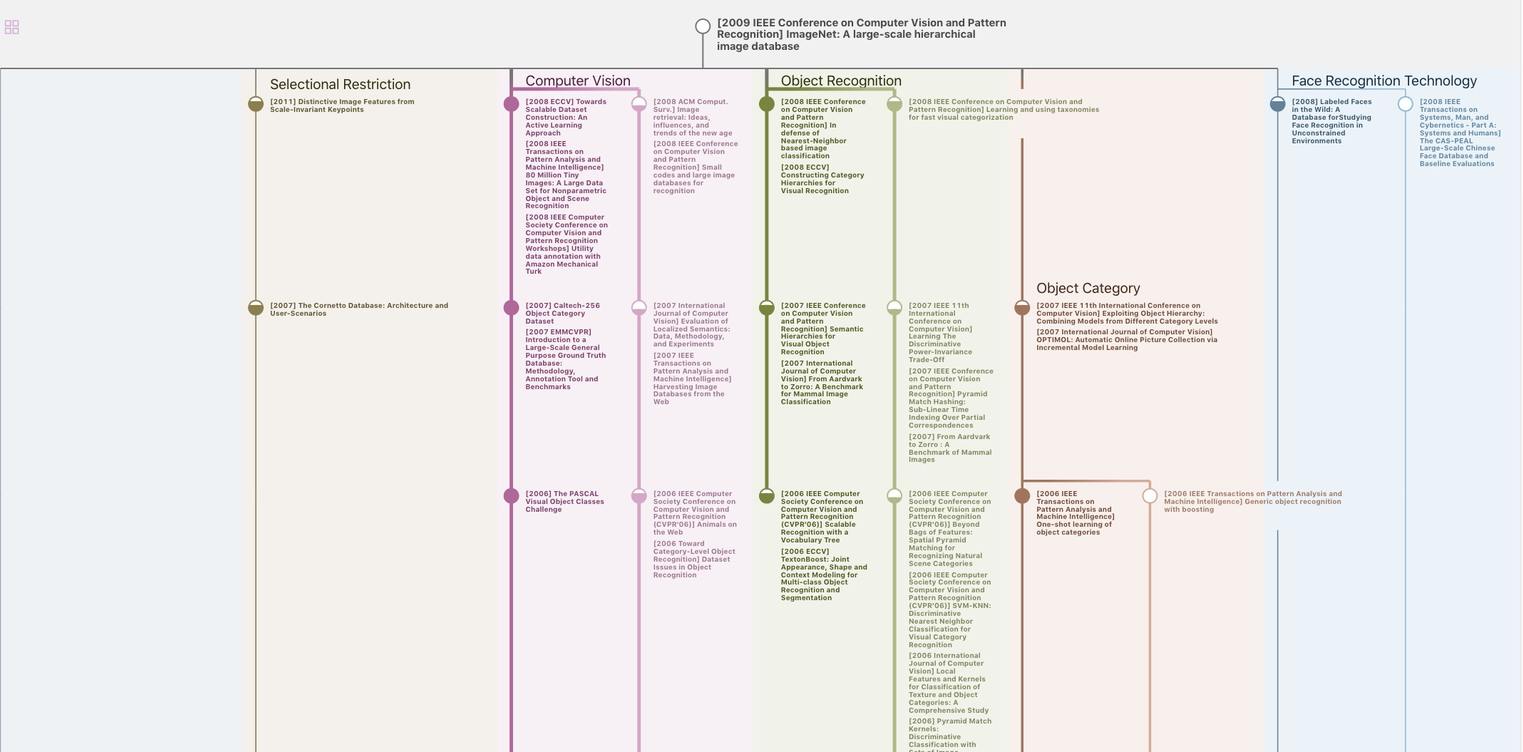
生成溯源树,研究论文发展脉络
Chat Paper
正在生成论文摘要