Variational Predictive Routing with Nested Subjective Timescales.
International Conference on Learning Representations (ICLR)(2022)
摘要
Discovery and learning of an underlying spatiotemporal hierarchy in sequential data is an important topic for machine learning. Despite this, little work has been done to explore hierarchical generative models that can flexibly adapt their layerwise representations in response to datasets with different temporal dynamics. Here, we present Variational Predictive Routing (VPR) - a neural probabilistic inference system that organizes latent representations of video features in a temporal hierarchy, based on their rates of change, thus modeling continuous data as a hierarchical renewal process. By employing an event detection mechanism that relies solely on the system's latent representations (without the need of a separate model), VPR is able to dynamically adjust its internal state following changes in the observed features, promoting an optimal organisation of representations across the levels of the model's latent hierarchy. Using several video datasets, we show that VPR is able to detect event boundaries, disentangle spatiotemporal features across its hierarchy, adapt to the dynamics of the data, and produce accurate time-agnostic rollouts of the future. Our approach integrates insights from neuroscience and introduces a framework with high potential for applications in model-based reinforcement learning, where flexible and informative state-space rollouts are of particular interest.
更多查看译文
关键词
Hierarchical temporal abstraction,event discovery,hierarchical generative models,variational inference
AI 理解论文
溯源树
样例
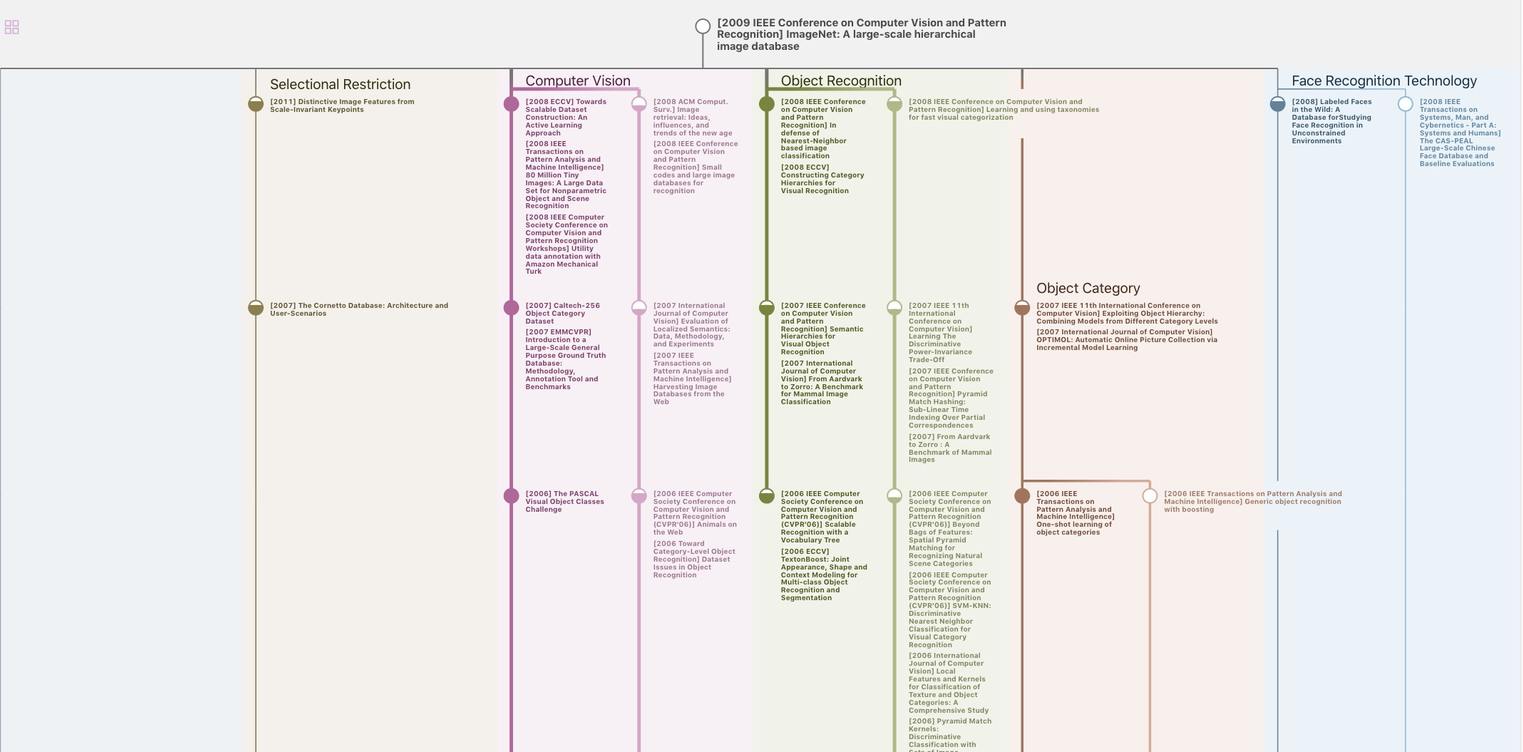
生成溯源树,研究论文发展脉络
Chat Paper
正在生成论文摘要