Automatic Maasailand Boma Mapping with Deep Neural Networks.
IGARSS(2021)
摘要
A prevalent challenge in underdeveloped countries is the mapping and accounting of certain sub-populations for public health matters. This is especially true in sub-Saharan African countries that lack specific resources and technologies. Often, international non-profit groups provide targeted support for populations' health on specific matters, such as clean water initiatives and basic health clinics. This is especially critical in various ethnic populations that are not well integrated into a country's existing public health services. In countries such as Tanzania, one such ethnic population is the Maasai people. In this paper, we demonstrate how machine learning algorithms, combined with remote sensing data, can aid non-profit health delivery organizations to map Maasailand boma homesteads in northern Tanzania. Our study area encompasses over 3900 square kilometers of Massailand, where we have manually identified 635 bomas. We have evaluated four different deep neural network architectures for their ability to recognize boma, ResNet50, Xception, Inception-ResNet-V2, and ProxylessNAS, with cross-validation F1 scores of 95.32%, 95.45%, 96.78%, and 97.28%, respectively.
更多查看译文
关键词
prevalent challenge,sub-populations,public health matters,sub-Saharan African countries,international nonprofit groups,ethnic population,public health services,machine learning algorithms,remote sensing data,nonprofit health delivery organizations,map Maasailand boma homesteads,northern Tanzania,automatic maasailand boma mapping,deep neural network architectures,recognize boma,proxylessNAS,cross-validation,inception-ResNet-V2
AI 理解论文
溯源树
样例
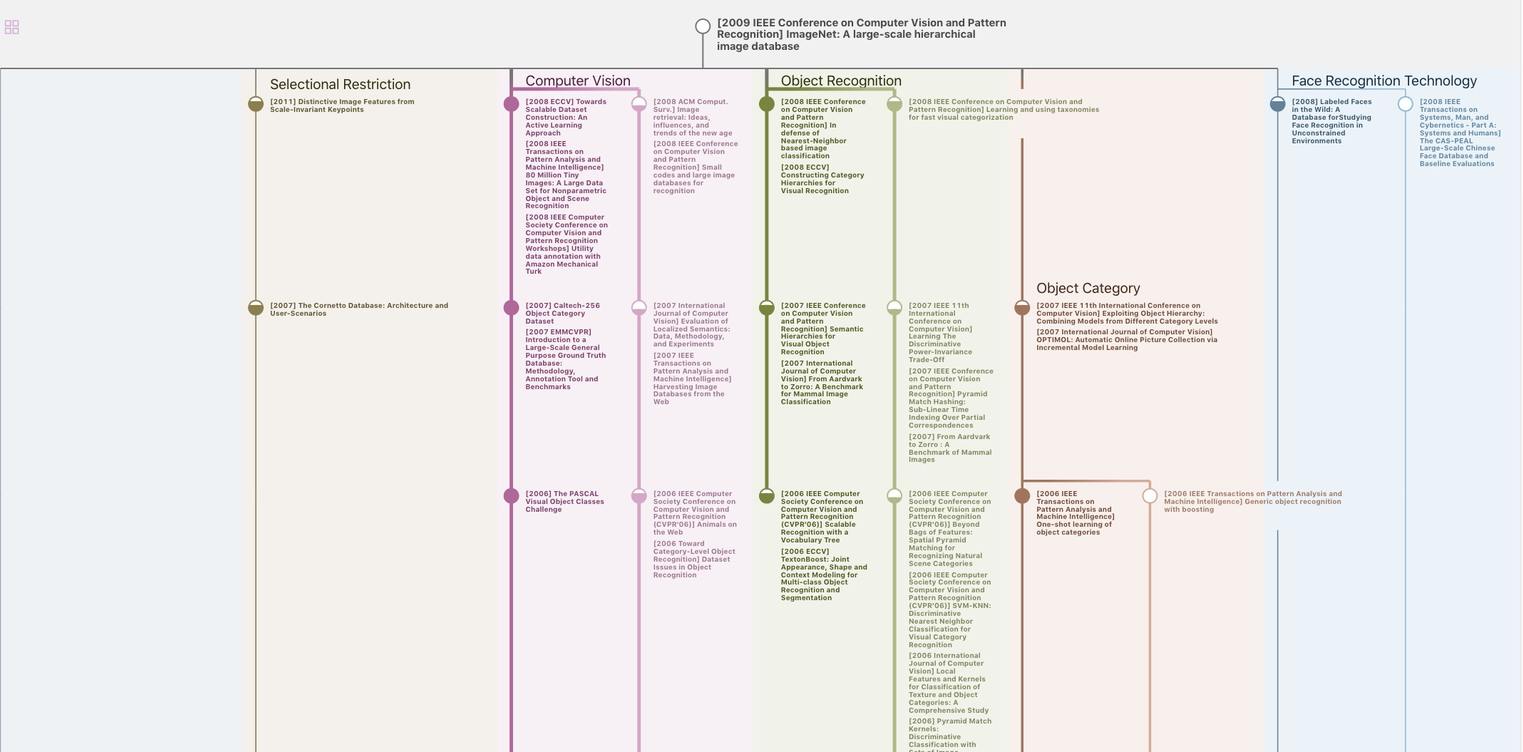
生成溯源树,研究论文发展脉络
Chat Paper
正在生成论文摘要