Beyond Exact Gradients: Convergence of Stochastic Soft-Max Policy Gradient Methods with Entropy Regularization
arxiv(2021)
摘要
Entropy regularization is an efficient technique for encouraging exploration and preventing a premature convergence of (vanilla) policy gradient methods in reinforcement learning (RL). However, the theoretical understanding of entropy regularized RL algorithms has been limited. In this paper, we revisit the classical entropy regularized policy gradient methods with the soft-max policy parametrization, whose convergence has so far only been established assuming access to exact gradient oracles. To go beyond this scenario, we propose the first set of (nearly) unbiased stochastic policy gradient estimators with trajectory-level entropy regularization, with one being an unbiased visitation measure-based estimator and the other one being a nearly unbiased yet more practical trajectory-based estimator. We prove that although the estimators themselves are unbounded in general due to the additional logarithmic policy rewards introduced by the entropy term, the variances are uniformly bounded. This enables the development of the first set of convergence results for stochastic entropy regularized policy gradient methods to both stationary points and globally optimal policies. We also develop some improved sample complexity results under a good initialization.
更多查看译文
关键词
exact gradients,regularization,soft-max
AI 理解论文
溯源树
样例
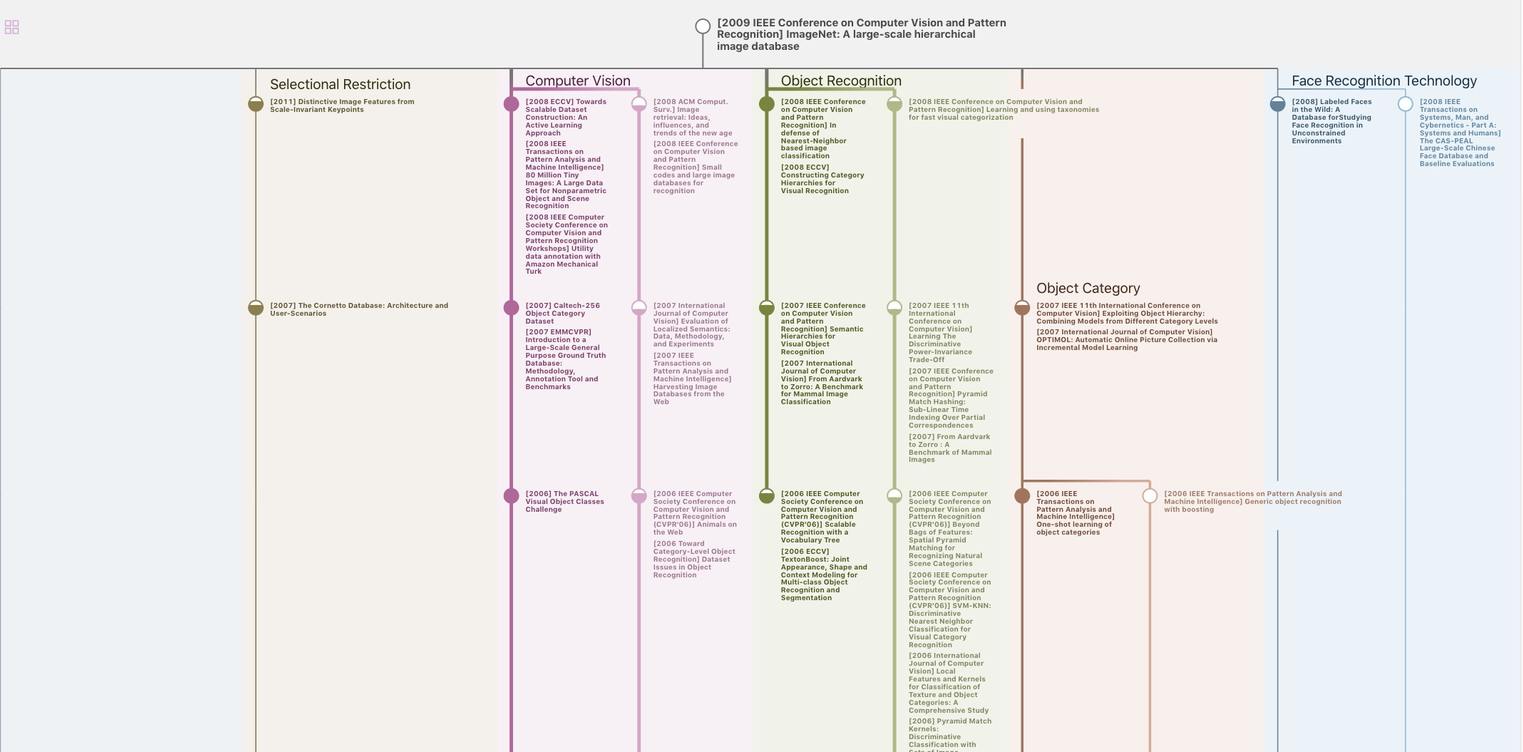
生成溯源树,研究论文发展脉络
Chat Paper
正在生成论文摘要