Permutation Invariance of Deep Neural Networks with ReLUs
NASA Formal Methods (NFM)(2022)
摘要
Consider a deep neural network (DNN) that is being used to suggest the direction in which an aircraft must turn to avoid a possible collision with an intruder aircraft. Informally, such a network is well-behaved if it asks the own ship to turn right (left) when an intruder approaches from the left (right). Consider another network that takes four inputs -- the cards dealt to the players in a game of contract bridge -- and decides which team can bid game. Loosely speaking, if you exchange the hands of partners (north and south, or east and west), the decision would not change. However, it will change if, say, you exchange north's hand with east. This permutation invariance property, for certain permutations at input and output layers, is central to the correctness and robustness of these networks. This paper proposes a sound, abstraction-based technique to establish permutation invariance in DNNs with ReLU as the activation function. The technique computes an over-approximation of the reachable states, and an under-approximation of the safe states, and propagates this information across the layers, both forward and backward. The novelty of our approach lies in a useful tie-class analysis, that we introduce for forward propagation, and a scalable 2-polytope under-approximation method that escapes the exponential blow-up in the number of regions during backward propagation. An experimental comparison shows the efficiency of our algorithm over that of verifying permutation invariance as a two-safety property (using FFNN verification over two copies of the network).
更多查看译文
关键词
deep neural networks,neural networks,invariance
AI 理解论文
溯源树
样例
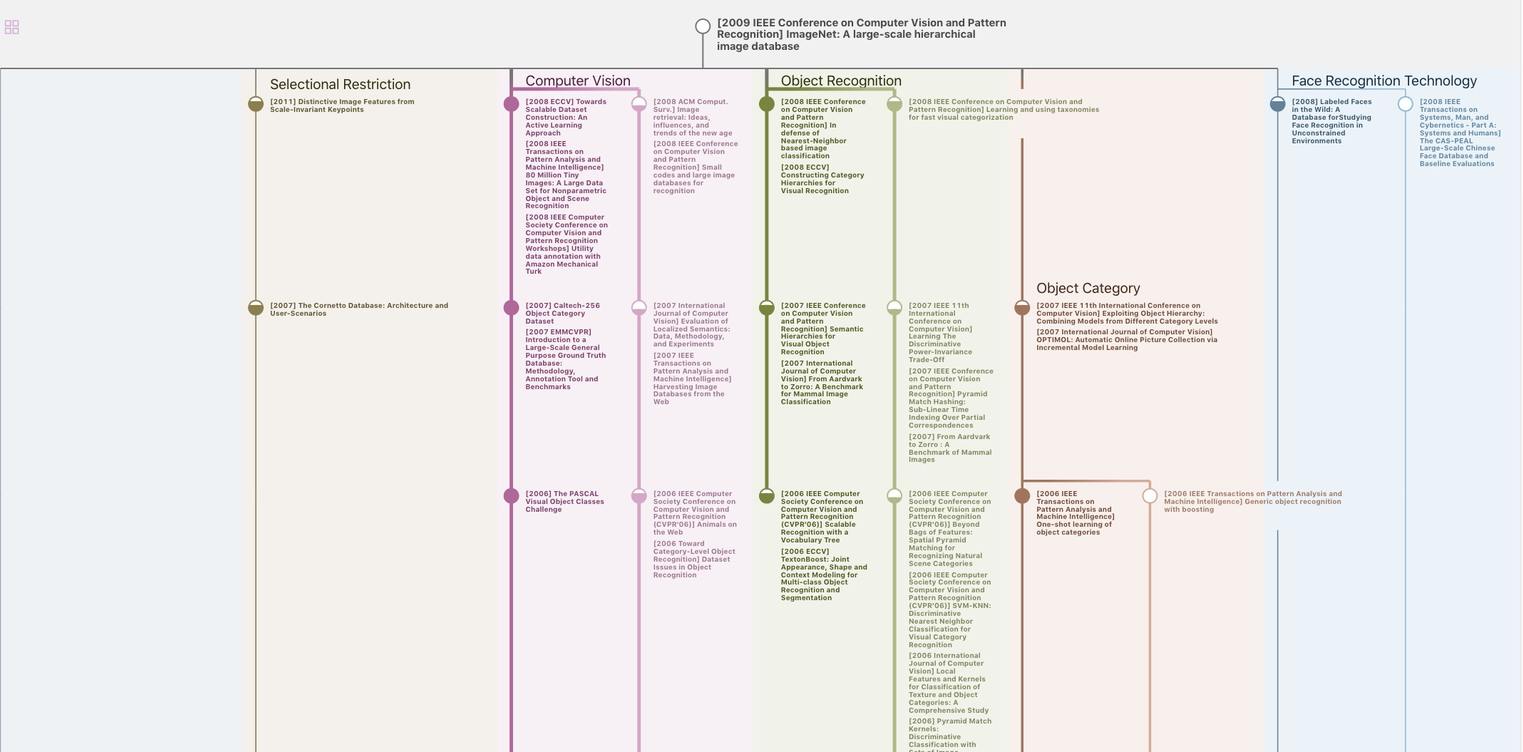
生成溯源树,研究论文发展脉络
Chat Paper
正在生成论文摘要