Keypoint-Based Bimanual Shaping of Deformable Linear Objects under Environmental Constraints using Hierarchical Action Planning
arxiv(2021)
摘要
This paper addresses the problem of contact-based manipulation of deformable linear objects (DLOs) towards desired shapes with a dual-arm robotic system. To alleviate the burden of high-dimensional continuous state-action spaces, we model the DLO as a kinematic multibody system via our proposed keypoint detection network. This new perception network is trained on a synthetic labeled image dataset and transferred to real manipulation scenarios without conducting any manual annotations. Our goal-conditioned policy can efficiently learn to rearrange the configuration of the DLO based on the detected keypoints. The proposed hierarchical action framework tackles the manipulation problem in a coarse-to-fine manner (with high-level task planning and low-level motion control) by leveraging on two action primitives. The identification of deformation properties is avoided since the algorithm replans its motion after each bimanual execution. The conducted experimental results reveal that our method achieves high performance in state representation of the DLO, and is robust to uncertain environmental constraints.
更多查看译文
关键词
hierarchical action planning,deformable linear objects,bimanual shaping,constraints,keypoint-based
AI 理解论文
溯源树
样例
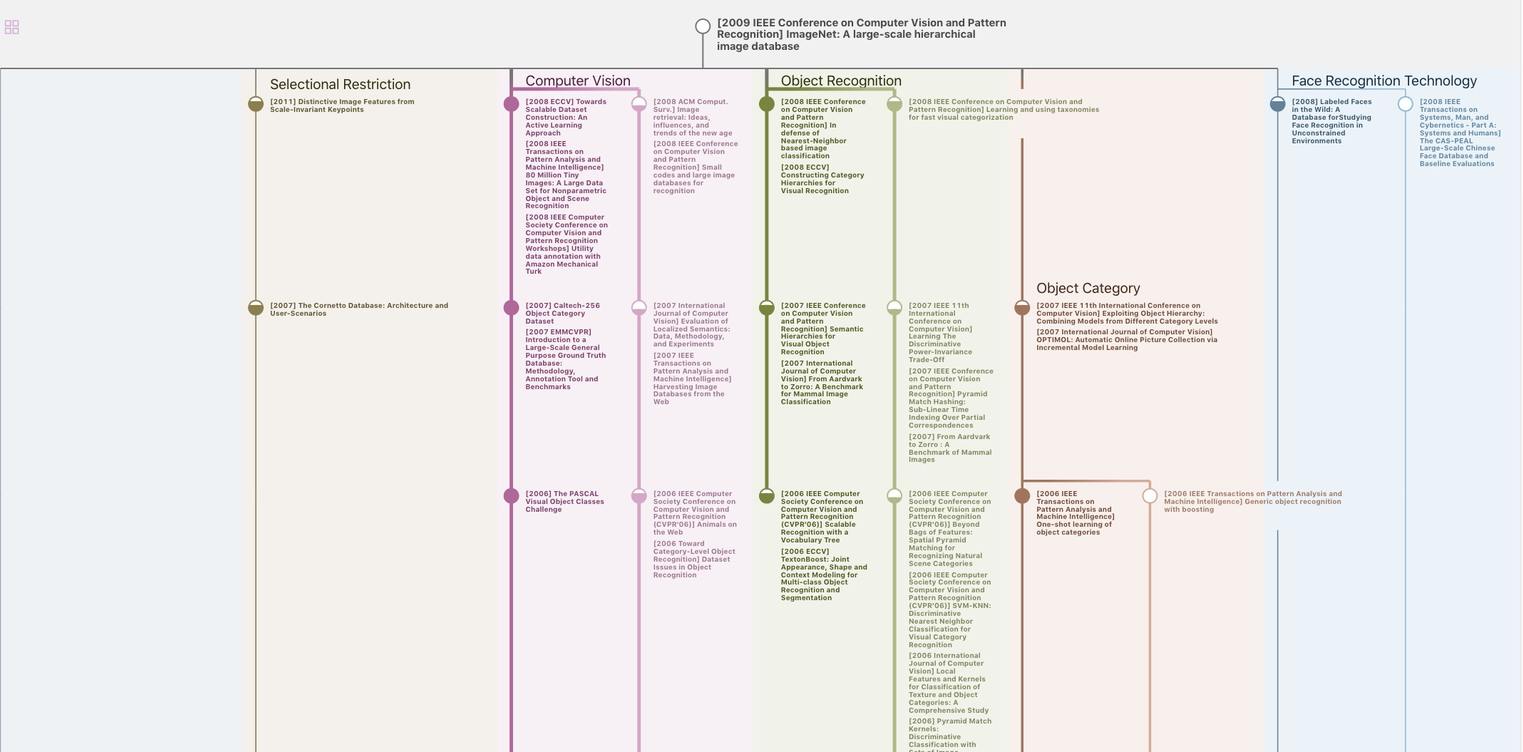
生成溯源树,研究论文发展脉络
Chat Paper
正在生成论文摘要