A Dual Approach to Constrained Markov Decision Processes with Entropy Regularization
INTERNATIONAL CONFERENCE ON ARTIFICIAL INTELLIGENCE AND STATISTICS, VOL 151(2022)
摘要
We study entropy-regularized constrained Markov decision processes (CMDPs) under the soft-max parameterization, in which an agent aims to maximize the entropy-regularized value function while satisfying constraints on the expected total utility. By leveraging the entropy regularization, our theoretical analysis shows that its Lagrangian dual function is smooth and the Lagrangian duality gap can be decomposed into the primal optimality gap and the constraint violation. Furthermore, we propose an accelerated dual-descent method for entropy-regularized CMDPs. We prove that our method achieves the global convergence rate (O) over tilde (1/T) for both the optimality gap and the constraint violation for entropy-regularized CMDPs. A discussion about a linear convergence rate for CMDPs with a single constraint is also provided.
更多查看译文
关键词
constrained markov decision processes,markov decision processes,regularization,dual approach
AI 理解论文
溯源树
样例
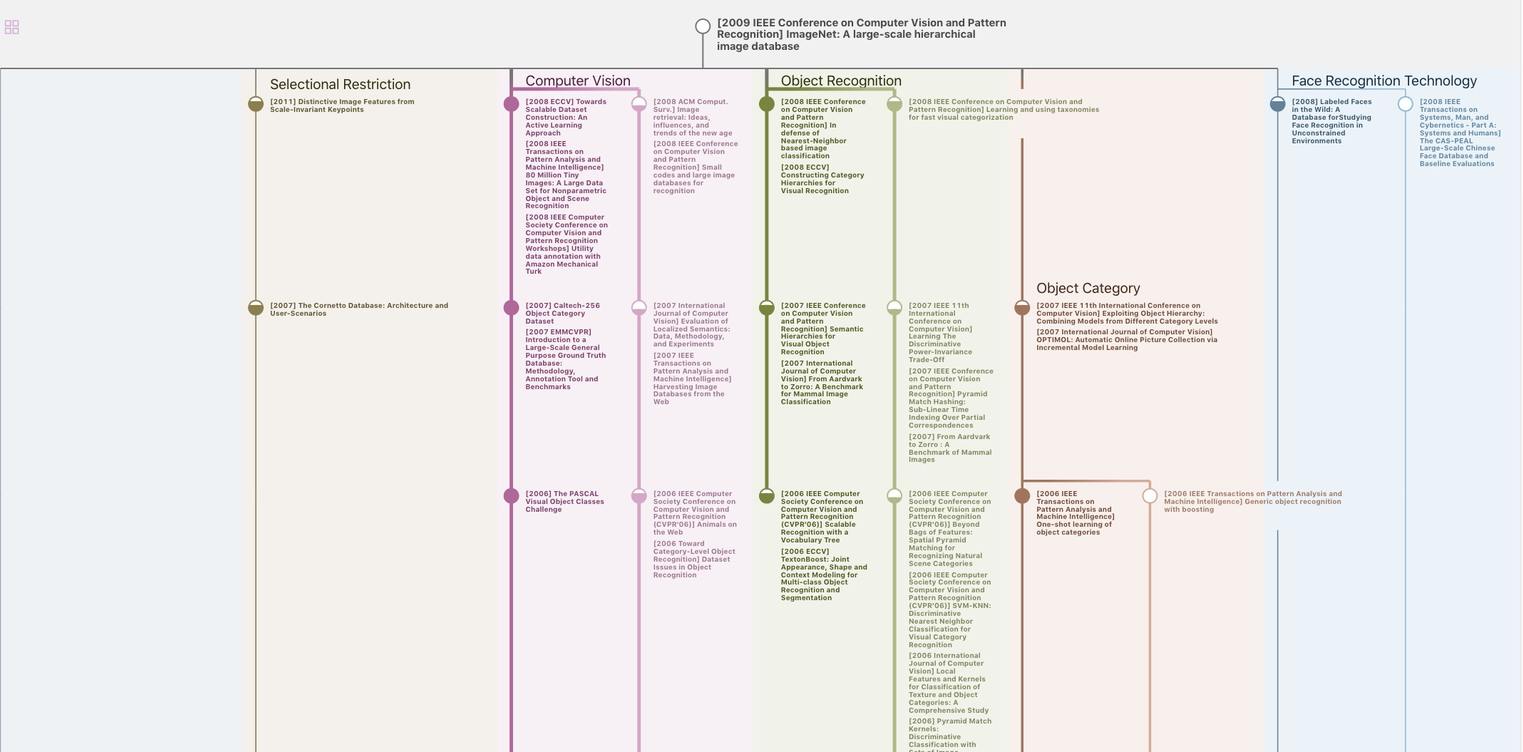
生成溯源树,研究论文发展脉络
Chat Paper
正在生成论文摘要