Temporally stable video segmentation without video annotations
2022 IEEE WINTER CONFERENCE ON APPLICATIONS OF COMPUTER VISION (WACV 2022)(2022)
摘要
Temporally consistent dense video annotations are scarce and hard to collect. In contrast, image segmentation datasets (and pre-trained models) are ubiquitous, and easier to label for any novel task. In this paper, we introduce a method to adapt still image segmentation models to video in an unsupervised manner; by using an optical flow-based consistency measure. To ensure that the inferred segmented videos appear more stable in practice, we verify that the consistency measure is well correlated with human judgement via a user study. Training a new multi-input multi-output decoder using this measure as a loss, together with a technique for refining current image segmentation datasets and a temporal weighted-guided filter; we observe stability improvements in the generated segmented videos with minimal loss of accuracy.
更多查看译文
关键词
Segmentation,Grouping and Shape Deep Learning
AI 理解论文
溯源树
样例
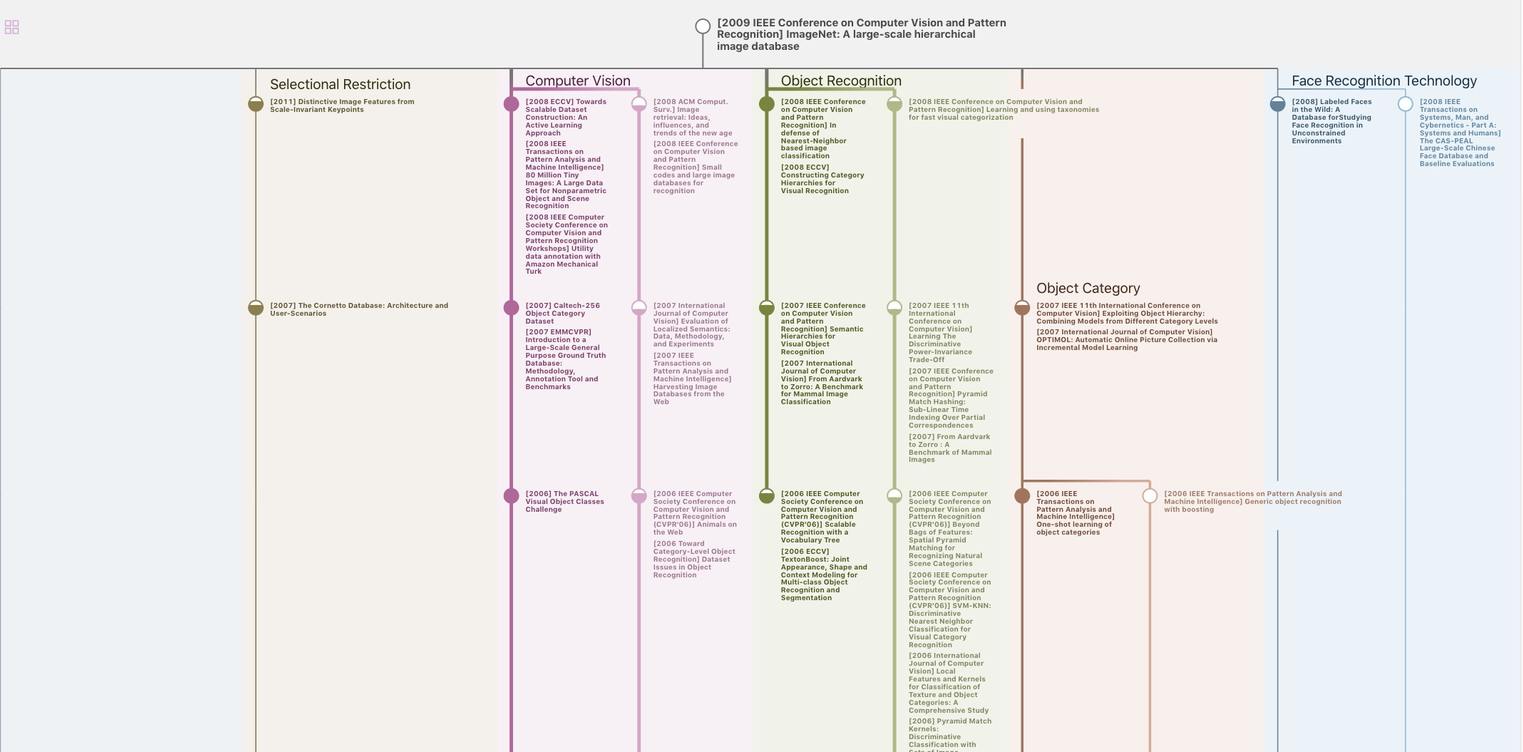
生成溯源树,研究论文发展脉络
Chat Paper
正在生成论文摘要