Evaluation of Hyperparameter-Optimization Approaches in an Industrial Federated Learning System
arxiv(2021)
摘要
Federated Learning (FL) decouples model training from the need for direct access to the data and allows organizations to collaborate with industry partners to reach a satisfying level of performance without sharing vulnerable business information. The performance of a machine learning algorithm is highly sensitive to the choice of its hyperparameters. In an FL setting, hyperparameter optimization poses new challenges. In this work, we investigated the impact of different hyperparameter optimization approaches in an FL system. In an effort to reduce communication costs, a critical bottleneck in FL, we investigated a local hyperparameter optimization approach that -- in contrast to a global hyperparameter optimization approach -- allows every client to have its own hyperparameter configuration. We implemented these approaches based on grid search and Bayesian optimization and evaluated the algorithms on the MNIST data set using an i.i.d. partition and on an Internet of Things (IoT) sensor based industrial data set using a non-i.i.d. partition.
更多查看译文
关键词
federated learning system,hyperparameter-optimization
AI 理解论文
溯源树
样例
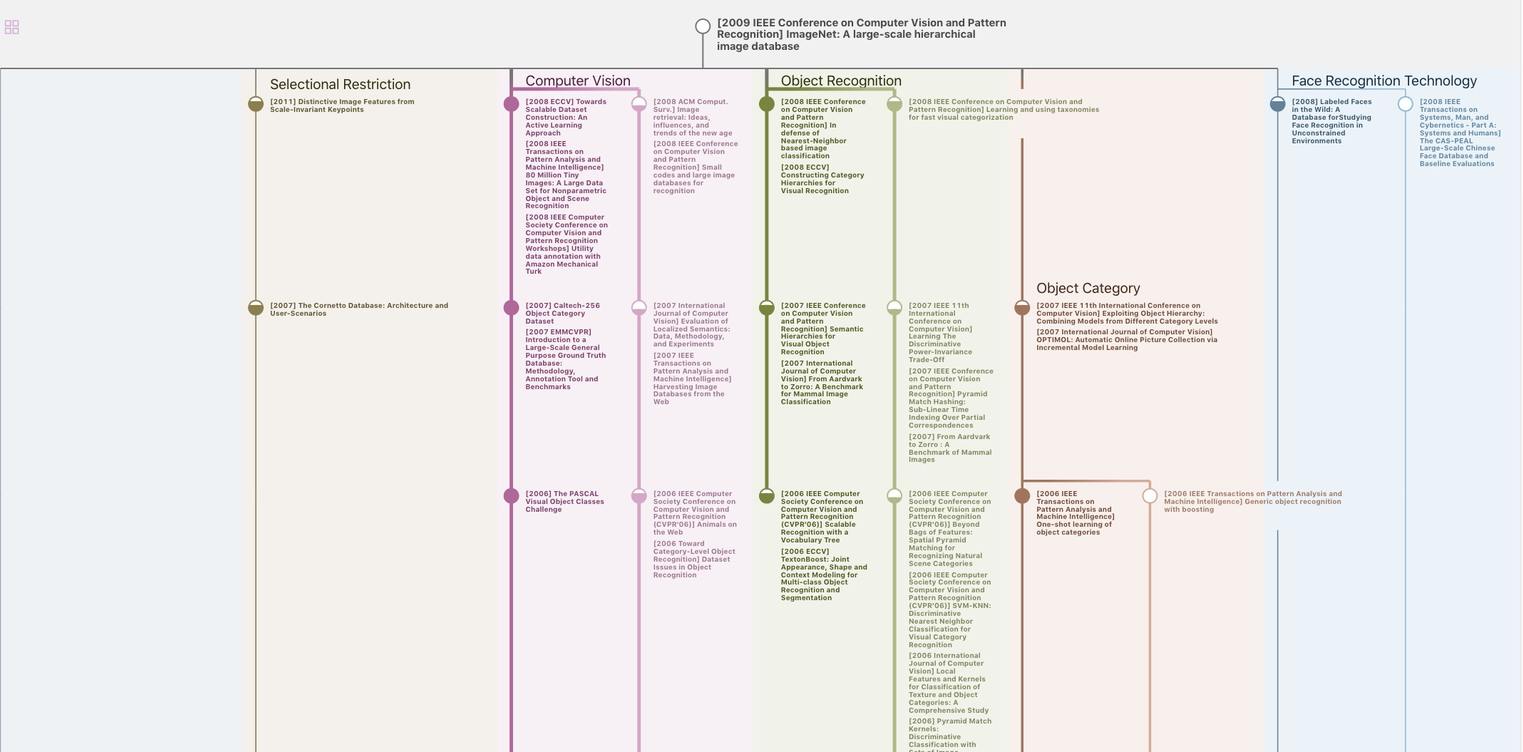
生成溯源树,研究论文发展脉络
Chat Paper
正在生成论文摘要