An active learning approach for improving the performance of equilibrium based chemical simulations
Computational Geosciences(2022)
摘要
In this paper, we propose a novel sequential data-driven method for dealing with equilibrium based chemical simulations, which can be seen as a specific machine learning approach called active learning. The underlying idea of our approach is to consider the function to estimate as a sample of a Gaussian process which allows us to compute the global uncertainty on the function estimation. Thanks to this estimation and with almost no parameter to tune, the proposed method sequentially chooses the most relevant input data at which the function to estimate has to be evaluated to build a surrogate model. Hence, the number of evaluations of the function to estimate is dramatically limited. Our active learning method is validated through numerical experiments and applied to a complex chemical system commonly used in geoscience.
更多查看译文
关键词
Machine learning, Gaussian Process, Chemical simulations
AI 理解论文
溯源树
样例
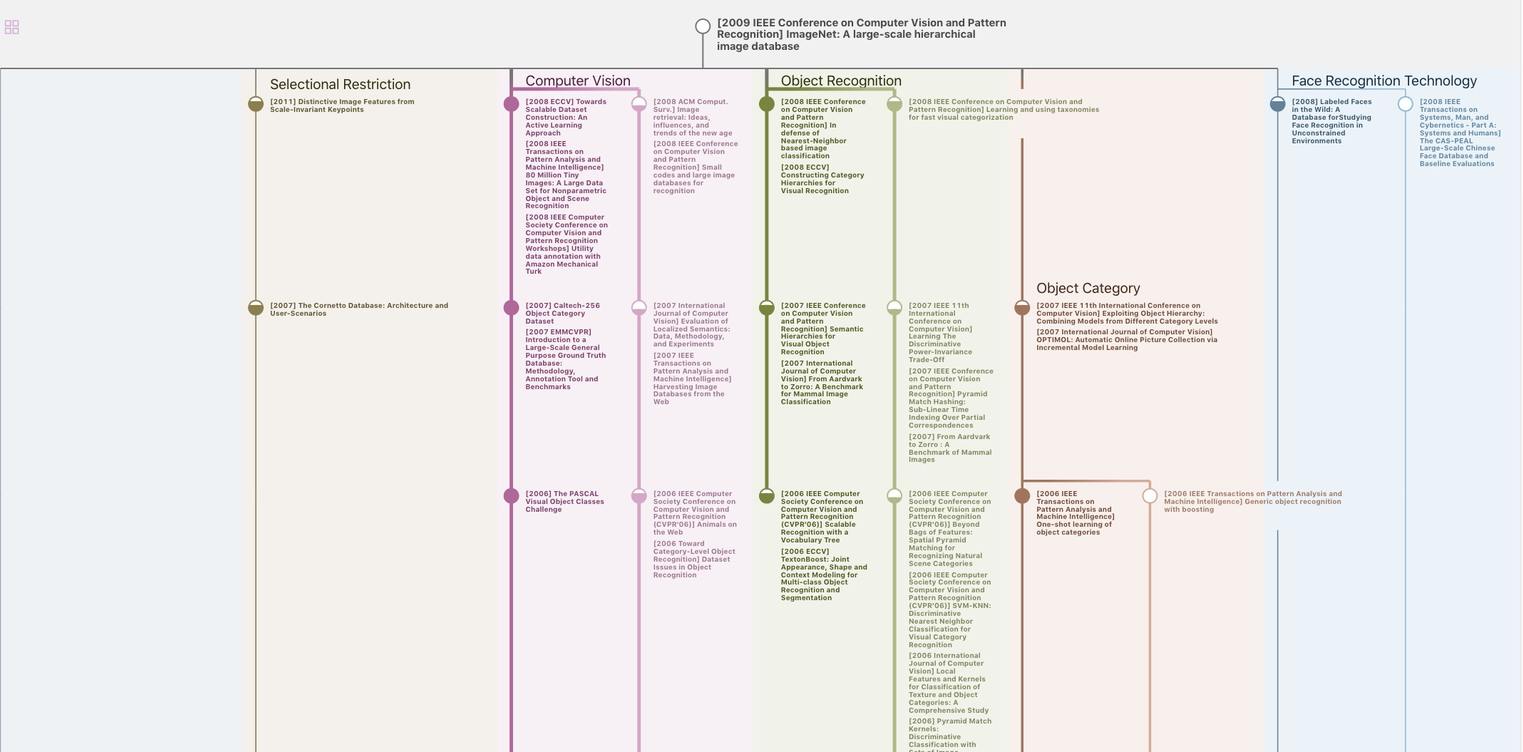
生成溯源树,研究论文发展脉络
Chat Paper
正在生成论文摘要