Large Scale Substitution-based Word Sense Induction
PROCEEDINGS OF THE 60TH ANNUAL MEETING OF THE ASSOCIATION FOR COMPUTATIONAL LINGUISTICS (ACL 2022), VOL 1: (LONG PAPERS)(2022)
摘要
We present a word-sense induction method based on pre-trained masked language models (MLMs), which can cheaply scale to large vocabularies and large corpora. The result is a corpus which is sense-tagged according to a corpus-derived sense inventory and where each sense is associated with indicative words. Evaluation on English Wikipedia that was sense-tagged using our method shows that both the induced senses, and the per-instance sense assignment, are of high quality even compared to WSD methods, such as Babelfy. Furthermore, by training a static word embeddings algorithm on the sense-tagged corpus, we obtain high-quality static senseful embeddings. These outperform existing senseful embeddings methods on the WiC dataset and on a new outlier detection dataset we developed. The data driven nature of the algorithm allows to induce corpora-specific senses, which may not appear in standard sense inventories, as we demonstrate using a case study on the scientific domain.
更多查看译文
关键词
induction,word,sense,substitution-based
AI 理解论文
溯源树
样例
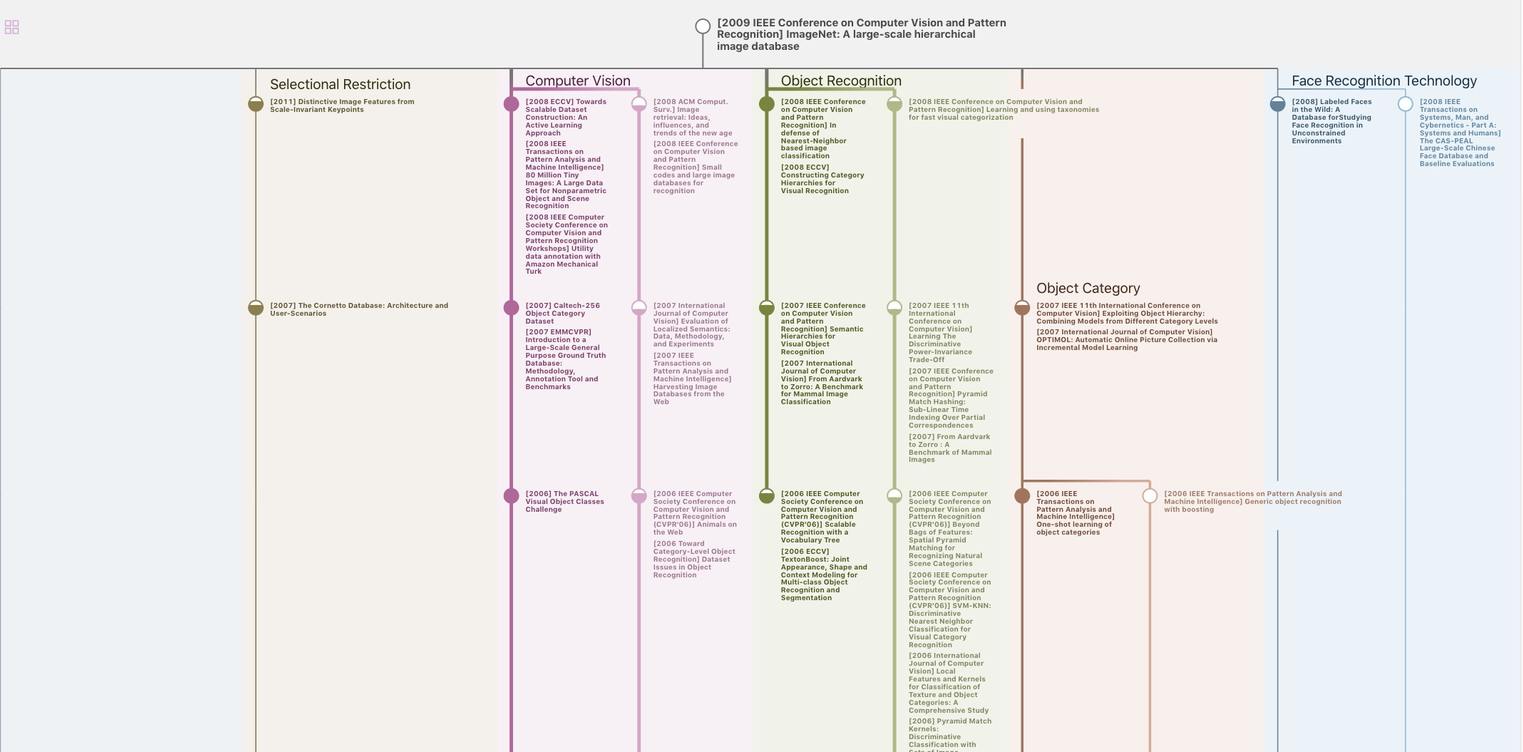
生成溯源树,研究论文发展脉络
Chat Paper
正在生成论文摘要