Multiscale Curvelet Scattering Network.
IEEE transactions on neural networks and learning systems(2021)
摘要
Feature representation has received more and more attention in image classification. Existing methods always directly extract features via convolutional neural networks (CNNs). Recent studies have shown the potential of CNNs when dealing with images' edges and textures, and some methods have been explored to further improve the representation process of CNNs. In this article, we propose a novel classification framework called the multiscale curvelet scattering network (MSCCN). Using the multiscale curvelet-scattering module (CCM), image features can be effectively represented. There are two parts in MSCCN, which are the multiresolution scattering process and the multiscale curvelet module. According to multiscale geometric analysis, curvelet features are utilized to improve the scattering process with more effective multiscale directional information. Specifically, the scattering process and curvelet features are effectively formulated into a unified optimization structure, with features from different scale levels being efficiently aggregated and learned. Furthermore, a one-level CCM, which can essentially improve the quality of feature representation, is constructed to be embedded into other existing networks. Extensive experimental results illustrate that MSCCN achieves better classification accuracy when compared with state-of-the-art techniques. Eventually, the convergence, insight, and adaptability are evaluated by calculating the trend of loss function's values, visualizing some feature maps, and performing generalization analysis.
更多查看译文
关键词
Scattering,Feature extraction,Convolution,Task analysis,Computer architecture,Spatial resolution,Neural networks,Curvelet,feature representation,image classification,multiscale,scattering
AI 理解论文
溯源树
样例
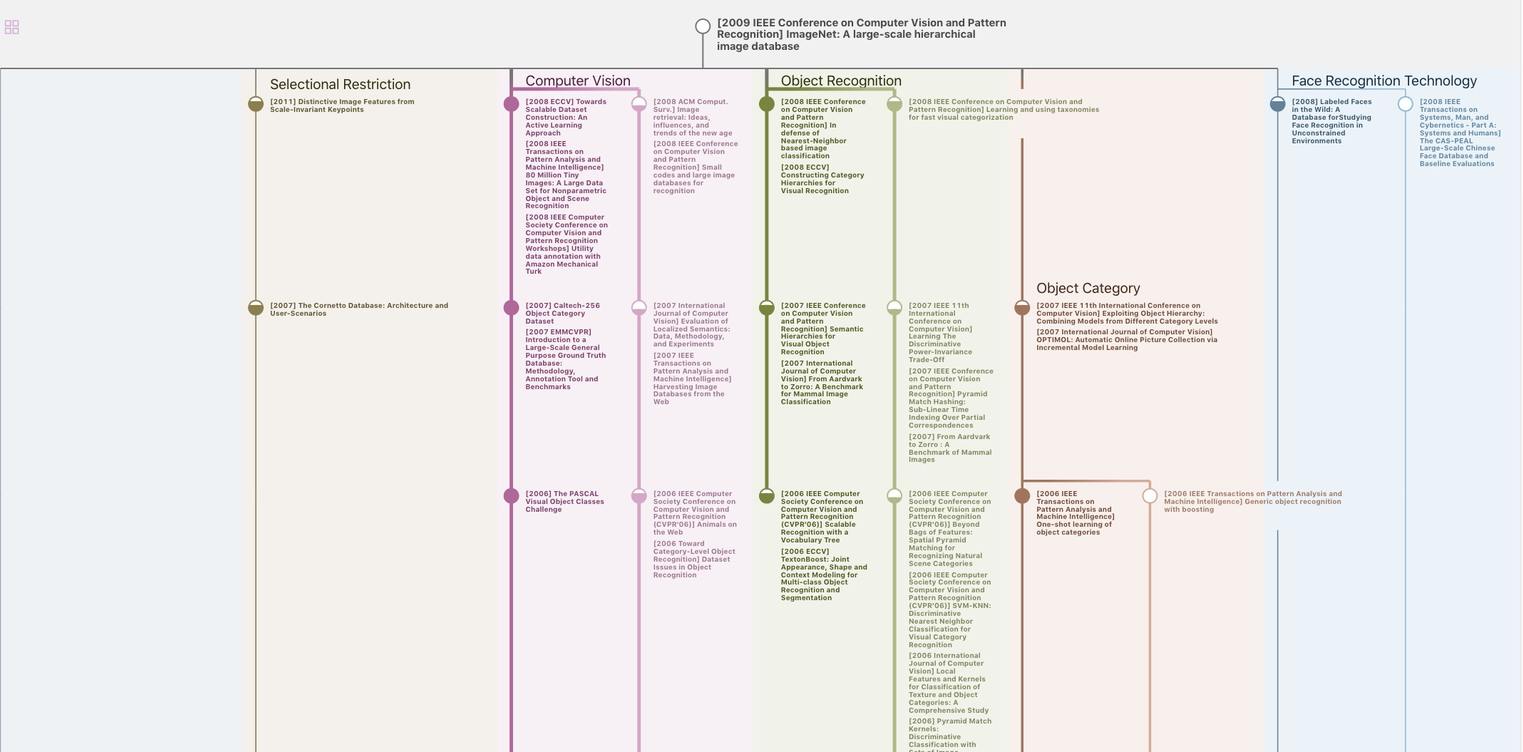
生成溯源树,研究论文发展脉络
Chat Paper
正在生成论文摘要