Adaptive Transformer for Multilingual Neural Machine Translation.
NLPCC(2021)
摘要
Multilingual neural machine translation (MNMT) with a single encoder-decoder model has attracted much interest due to its simple deployment and low training cost. However, the all-shared translation model often yields degraded performance due to the modeling capacity limitations and language diversity. Moreover, it has been revealed in recent studies that the shared parameters lead to negative language interference although they may also facilitate knowledge transfer across languages. In this work, we propose an adaptive architecture for multilingual modeling, which divides the parameters in MNMT sub-layers into shared and language-specific ones. We train the model to learn and balance the shared and unique features with different degrees of parameter sharing. We evaluate our model on one-to-many and many-to-one translation tasks. Experiments on IWSLT dataset show that our proposed model remarkably outperforms the multilingual baseline model and achieves comparable or even better performance compared with the bilingual model.
更多查看译文
关键词
transformer,translation
AI 理解论文
溯源树
样例
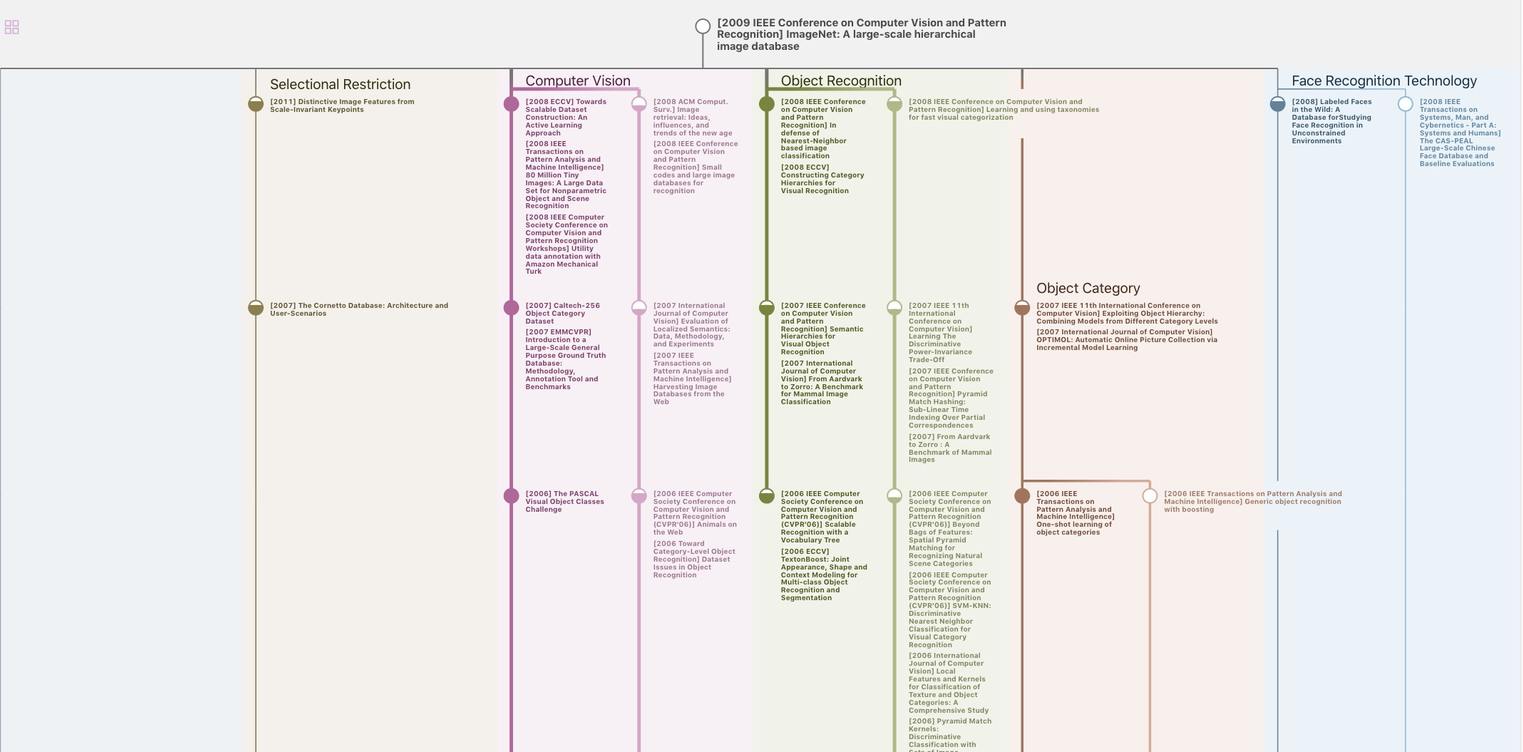
生成溯源树,研究论文发展脉络
Chat Paper
正在生成论文摘要