Reducing Uncertainty Using Placement and Regrasp Planning on a Triangular Corner Fixture
IEEE TRANSACTIONS ON AUTOMATION SCIENCE AND ENGINEERING(2024)
摘要
This paper presented a regrasp planning method to eliminate grasp uncertainty while considering the geometric constraints of a fixture. The method automatically finds the Stable Placement Poses (SPPs) of an object on a Triangular Corner Fixture (TCF), elevates the object from its SPPs to dropping poses and finds the Deterministic Dropping Poses (DDPs), builds regrasp graphs by using the SPP-DDP pairs and their associated grasp configurations, and searches the graph to find regrasp motion sequences for precise assembly. Since the SPPs and their associated regrasps are constrained by the TCF's geometry and have high precision, the final object poses regrasped via it has low uncertainty and can be directly used for assembly by position control. In the experimental section, we study the performance of analytical and learning-based methods for estimating the DDPs of different objects and quantitatively examine the proposed method's ability to suppress uncertainty using assembly tasks like peg-in-hole insertion and sheathing tubes, aligning holes, mounting bearing housings, etc. The results demonstrate the method's robustness and efficacy. Note to Practitioners-In production lines, robots interact with peripheral devices to improve efficiency and reduce uncertainty. In this work, we focus on a particular peripheral device - a Triangular Corner Fixture (TCF) made by three inclined and mutually perpendicular plates. We study using the TCF to improve manipulation precision. The inclined plates of the TCF form a gravity bucket that holds dropped objects in stable states under gravity. In a real scenario, a robot picks up an object and releases it above the TCF. The released object will reach a stable state on the TCF. Then, the robot regrasps and moves the stabilized object to the target pose with reduced uncertainty. Using the method proposed in this paper, a robot can automatically finish the above procedure by finding all the object's stable states in the TCF, planning grasp configurations, invalidating infeasible states and grasps, building regrasp graphs and searching the graph to find a regrasp motion sequence that moves the object to a goal pose with high precision for assembly. In industrial applications, the proposed method has the potential to improve the flexibility of robotic systems for high-precision tasks. In the research fields, it may promote the research on sensorless manipulation and extrinsic manipulation, and push forward the studies in robotic regrasp.
更多查看译文
关键词
Fixture,precise assembly,regrasp planning
AI 理解论文
溯源树
样例
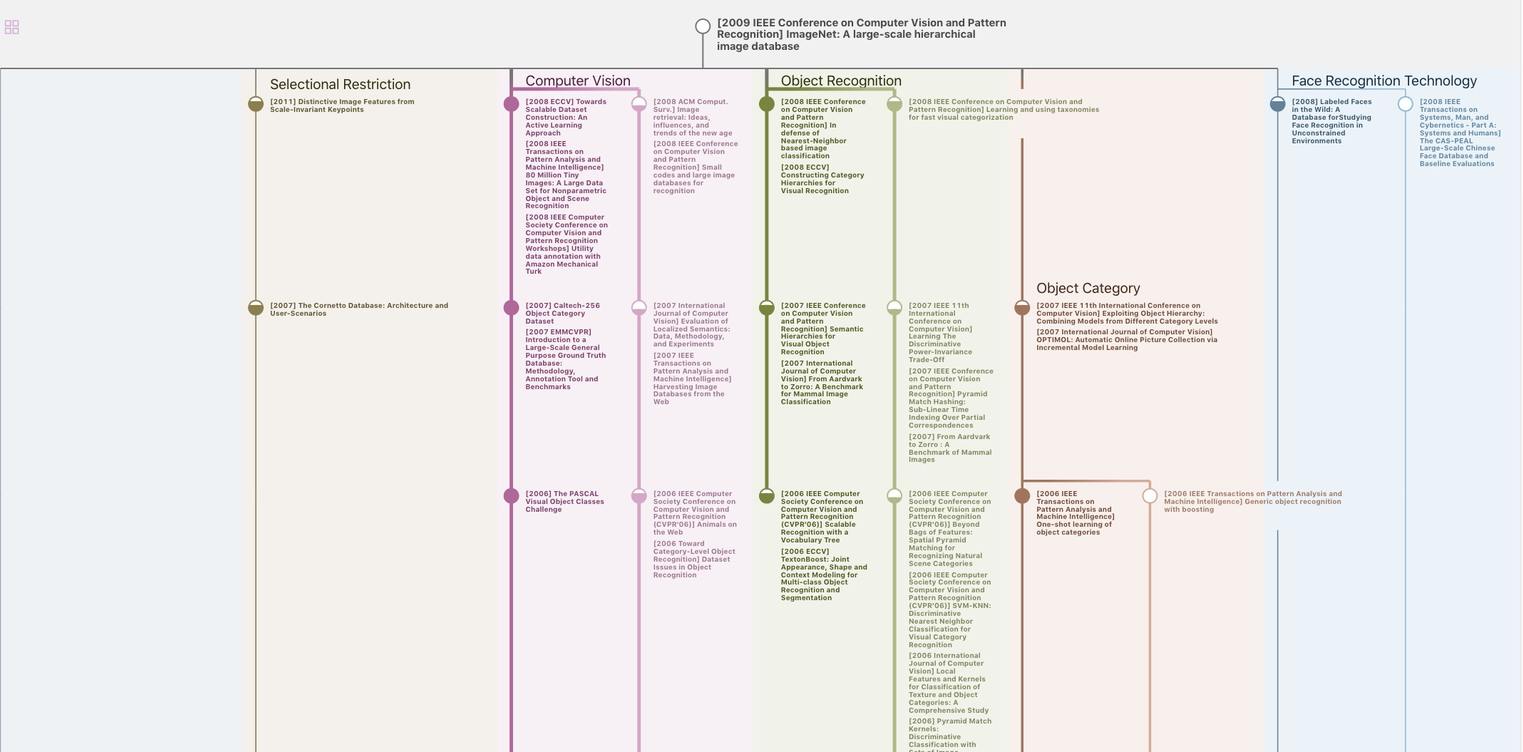
生成溯源树,研究论文发展脉络
Chat Paper
正在生成论文摘要