On the Sample Complexity of Decentralized Linear Quadratic Regulator With Partially Nested Information Structure
arxiv(2023)
摘要
In this article, we study the problem of control policy design for decentralized state-feedback linear quadratic control with a partially nested information structure, when the system model is unknown. We propose a model-based learning solution, which consists of two steps. First, we estimate the unknown system model from a single system trajectory of finite length, using least squares estimation. Next, based on the estimated system model, we design a decentralized control policy that satisfies the desired information structure. We show that the suboptimality gap between our control policy and the optimal decentralized control policy (designed using accurate knowledge of the system model) scales linearly with the estimation error of the system model. Using this result, we provide an end-to-end sample complexity result for learning decentralized controllers for a linear quadratic control problem with a partially nested information structure.
更多查看译文
关键词
decentralized linear quadratic regulator,sample complexity,information
AI 理解论文
溯源树
样例
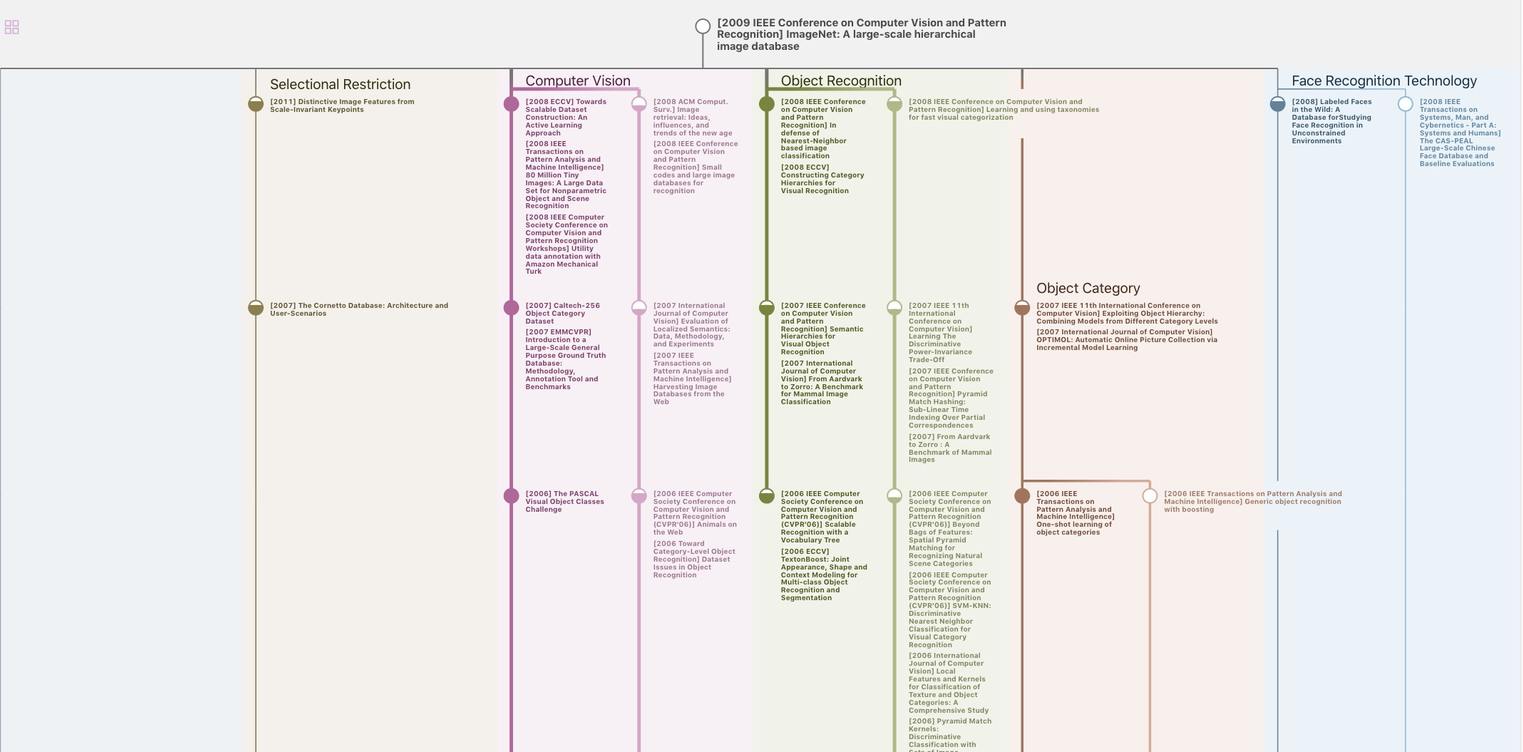
生成溯源树,研究论文发展脉络
Chat Paper
正在生成论文摘要