Learning from Crowdsourced Multi-labeling: A Variational Bayesian Approach
INFORMATION SYSTEMS RESEARCH(2021)
摘要
Microtask crowdsourcing has emerged as a cost-effective approach for obtaining large-scale labeled data. Crowdsourcing platforms, such as MTurk, provide an online marketplace where task requesters can submit a batch of microtasks for a crowd of workers to complete for a small monetary compensation. As the information collected from a crowd can be prone to errors, additional algorithmic techniques are needed to infer the ground truth labels from noisy annotations by workers with heterogeneous quality. Moreover, it would be very beneficial to identify and possibly filter out low-quality workers to foster the creation of a healthy and sustainable crowdsourcing ecosystem. Much of the existing literature on crowd labeling has focused on the single-label setting. However, in many application domains, it is common that each item to be annotated can be assigned to multiple categories simultaneously. In this paper, we present a variety of new approaches for modeling label dependency and worker quality in the context of multi-label crowdsourcing. To capture label dependency, we introduce three methods based on a Bayesian mixture of Bernoulli distributions, its Dirichlet process extension, and a multivariate logitnormal distribution. We also propose two distinct generative models for characterizing shared and hierarchical structures of worker quality. Efficient collapsed and Laplace variational inference algorithms are then developed to jointly infer ground truth labels and worker quality. Extensive simulation and MTurk experiments show that the models based on integrating Bernoulli mixtures and shared structure of worker quality achieve a signifi- cant improvement over other state-of-the-art methods. Our study clearly highlights that joint and effective modeling of label dependency and worker quality is crucial to the design of a multi-label crowdsourcing system. The proposed framework also has great potential to be extended to a broader range of applications, in which different opinions need to be combined to measure multiple perspectives of an object.
更多查看译文
关键词
microtask crowdsourcing, multi-label annotation aggregation, worker quality estimation, hierarchical Bayesian model, variational inference
AI 理解论文
溯源树
样例
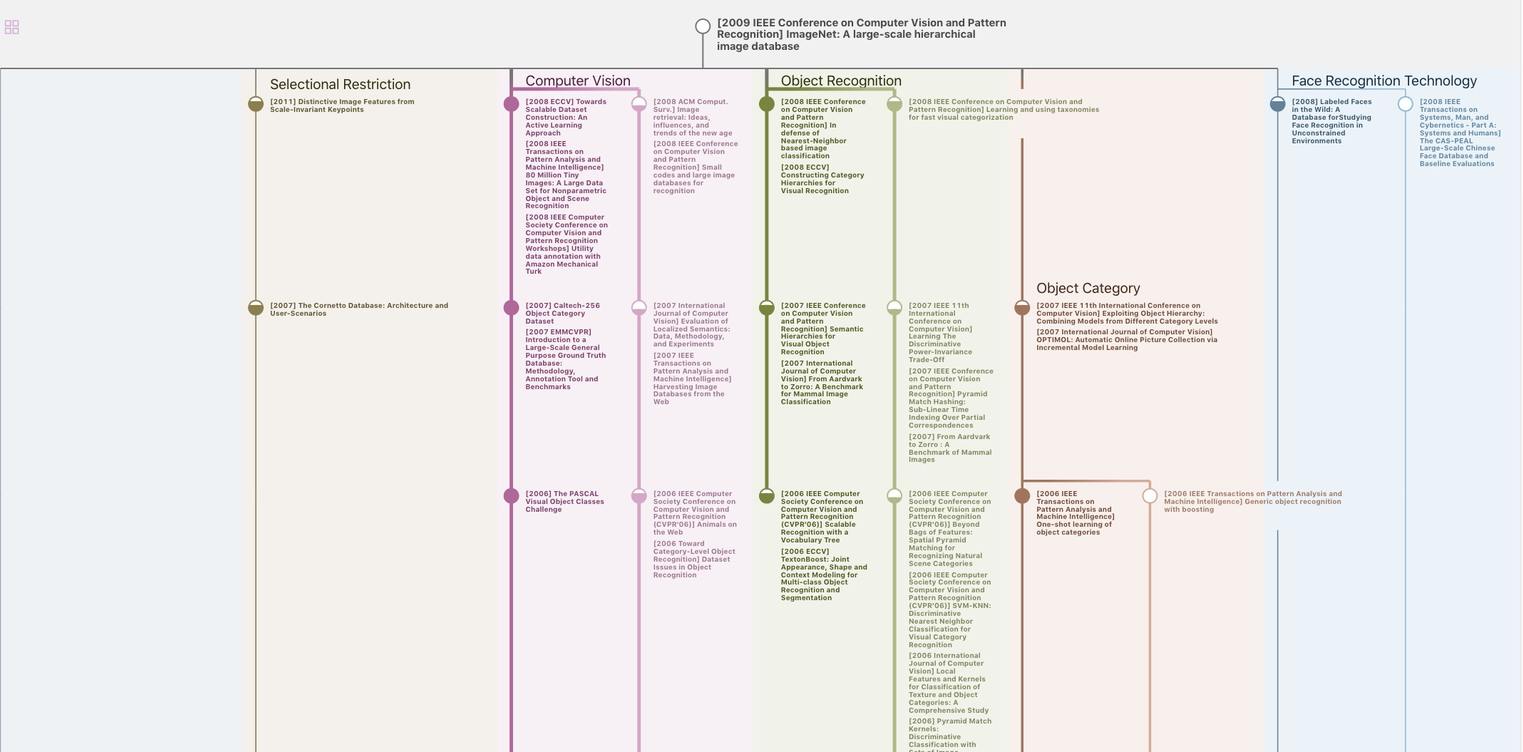
生成溯源树,研究论文发展脉络
Chat Paper
正在生成论文摘要