SHNE: Semantics and Homophily Preserving Network Embedding
IEEE transactions on neural networks and learning systems(2023)
摘要
Graph convolutional networks (GCNs) have achieved great success in many applications and have caught significant attention in both academic and industrial domains. However, repeatedly employing graph convolutional layers would render the node embeddings indistinguishable. For the sake of avoiding oversmoothing, most GCN-based models are restricted in a shallow architecture. Therefore, the expressive power of these models is insufficient since they ignore information beyond local neighborhoods. Furthermore, existing methods either do not consider the semantics from high-order local structures or neglect the node homophily (i.e., node similarity), which severely limits the performance of the model. In this article, we take above problems into consideration and propose a novel Semantics and Homophily preserving Network Embedding (SHNE) model. In particular, SHNE leverages higher order connectivity patterns to capture structural semantics. To exploit node homophily, SHNE utilizes both structural and feature similarity to discover potential correlated neighbors for each node from the whole graph; thus, distant but informative nodes can also contribute to the model. Moreover, with the proposed dual-attention mechanisms, SHNE learns comprehensive embeddings with additional information from various semantic spaces. Furthermore, we also design a semantic regularizer to improve the quality of the combined representation. Extensive experiments demonstrate that SHNE outperforms state-of-the-art methods on benchmark datasets.
更多查看译文
关键词
Semantics,Task analysis,Feature extraction,Aggregates,Learning systems,Training,Social networking (online),Graph convolutional network (GCN),network embedding,node homophily,structural semantics
AI 理解论文
溯源树
样例
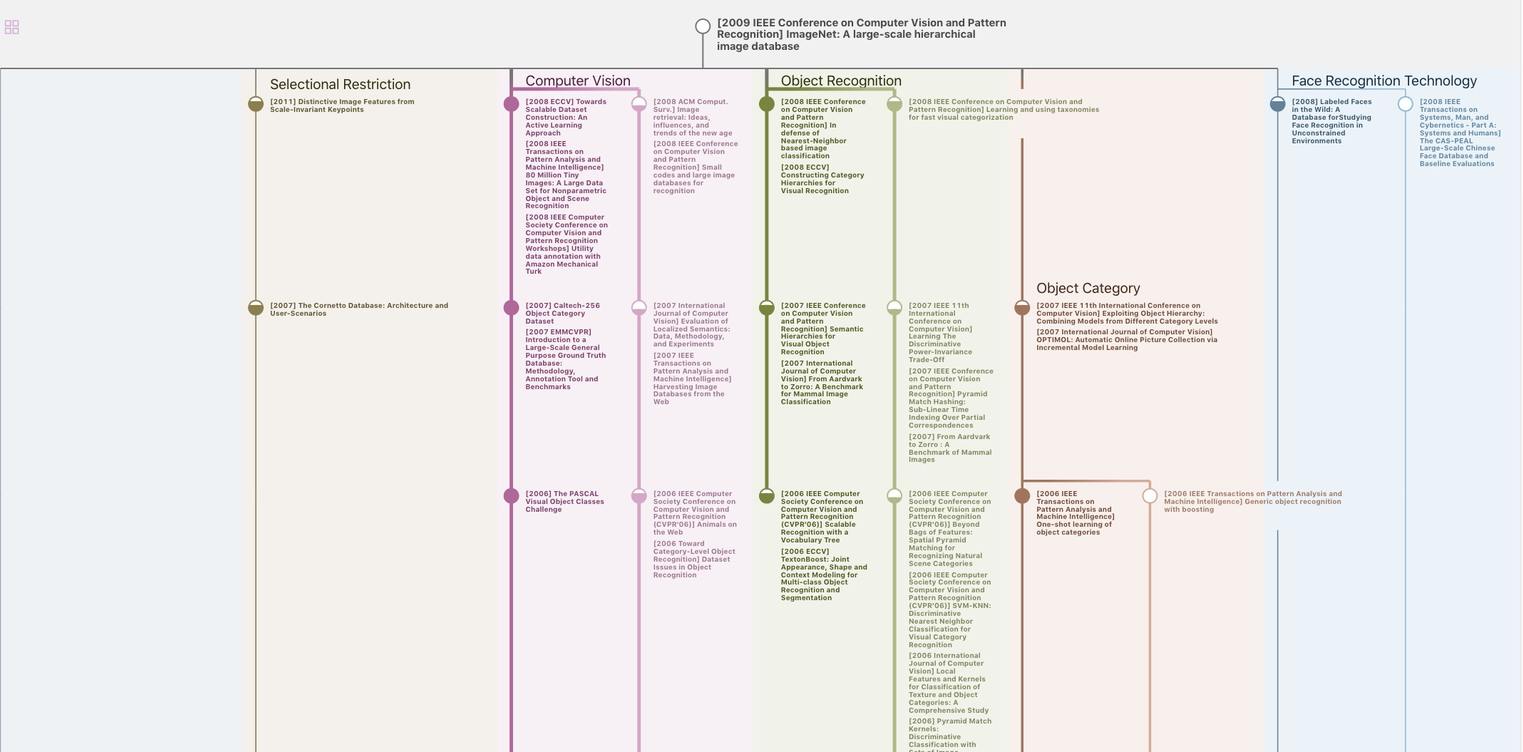
生成溯源树,研究论文发展脉络
Chat Paper
正在生成论文摘要