Learning Visual Shape Control of Novel 3D Deformable Objects from Partial-View Point Clouds
IEEE International Conference on Robotics and Automation(2022)
摘要
If robots could reliably manipulate the shape of 3D deformable objects, they could find applications in fields ranging from home care to warehouse fulfillment to surgical assistance. Analytic models of elastic, 3D deformable objects require numerous parameters to describe the potentially infinite degrees of freedom present in determining the object's shape. Previous attempts at performing 3D shape control rely on hand-crafted features to represent the object shape and require training of object-specific control models. We overcome these issues through the use of our novel DeformerNet neural network architecture, which operates on a partial-view point cloud of the object being manipulated and a point cloud of the goal shape to learn a low-dimensional representation of the object shape. This shape embedding enables the robot to learn to define a visual servo controller that provides Cartesian pose changes to the robot end-effector causing the object to deform towards its target shape. Crucially, we demonstrate both in simulation and on a physical robot that DeformerNet reliably generalizes to object shapes and material stiffness not seen during training and outperforms comparison methods for both the generic shape control and the surgical task of retraction.
更多查看译文
关键词
visual shape control,novel 3D deformable objects,partial-view point cloud,object shape,object-specific control models,goal shape,shape embedding,visual servo controller,target shape,generic shape control
AI 理解论文
溯源树
样例
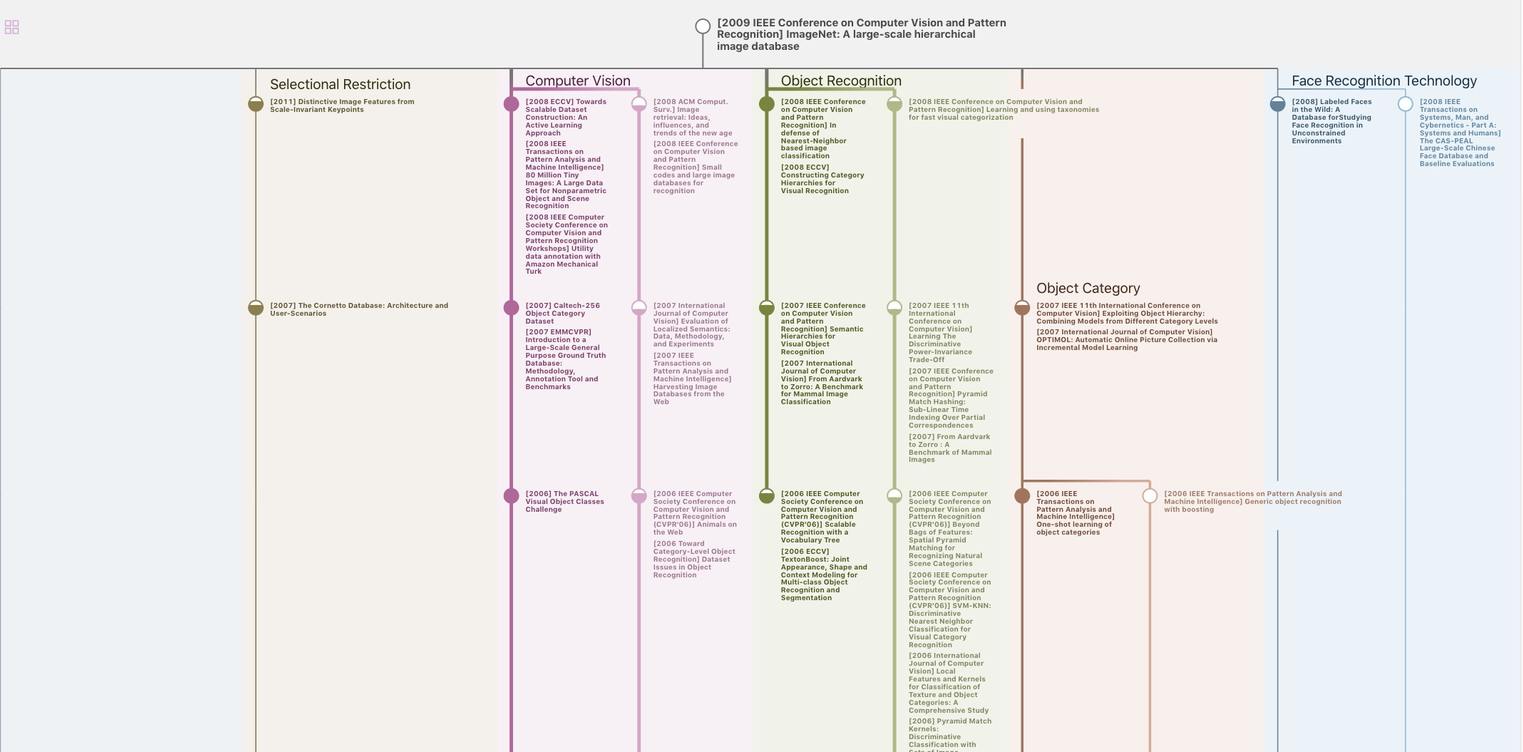
生成溯源树,研究论文发展脉络
Chat Paper
正在生成论文摘要