Focus Your Distribution: Coarse-to-Fine Non-Contrastive Learning for Anomaly Detection and Localization
IEEE International Conference on Multimedia and Expo (ICME)(2022)
摘要
The essence of unsupervised anomaly detection is to learn the compact distribution of normal samples and detect outliers as anomalies in testing. Meanwhile, the anomalies in real-world are usually subtle and fine-grained in a high-resolution image especially for industrial applications. Towards this end, we propose a novel framework for unsupervised anomaly detection and localization. Our method aims at learning dense and compact distribution from normal images with a coarse-to-fine alignment process. The coarse alignment stage standardizes the pixel-wise position of objects in both image and feature levels. The fine alignment stage then densely maximizes the similarity of features among all corresponding locations in a batch. To facilitate the learning with only normal images, we propose a new pretext task called non-contrastive learning for the fine alignment stage. Non-contrastive learning extracts robust and discriminating normal image representations without making assumptions on abnormal samples, and it thus empowers our model to generalize to various anomalous scenarios. Extensive experiments on two typical industrial datasets of MVTec AD and BenTech AD demonstrate that our framework is effective in detecting various real-world defects and achieves a new state-of-the-art in industrial unsupervised anomaly detection.
更多查看译文
关键词
Unsupervised anomaly detection,non-contrastive,coarse-to-fine
AI 理解论文
溯源树
样例
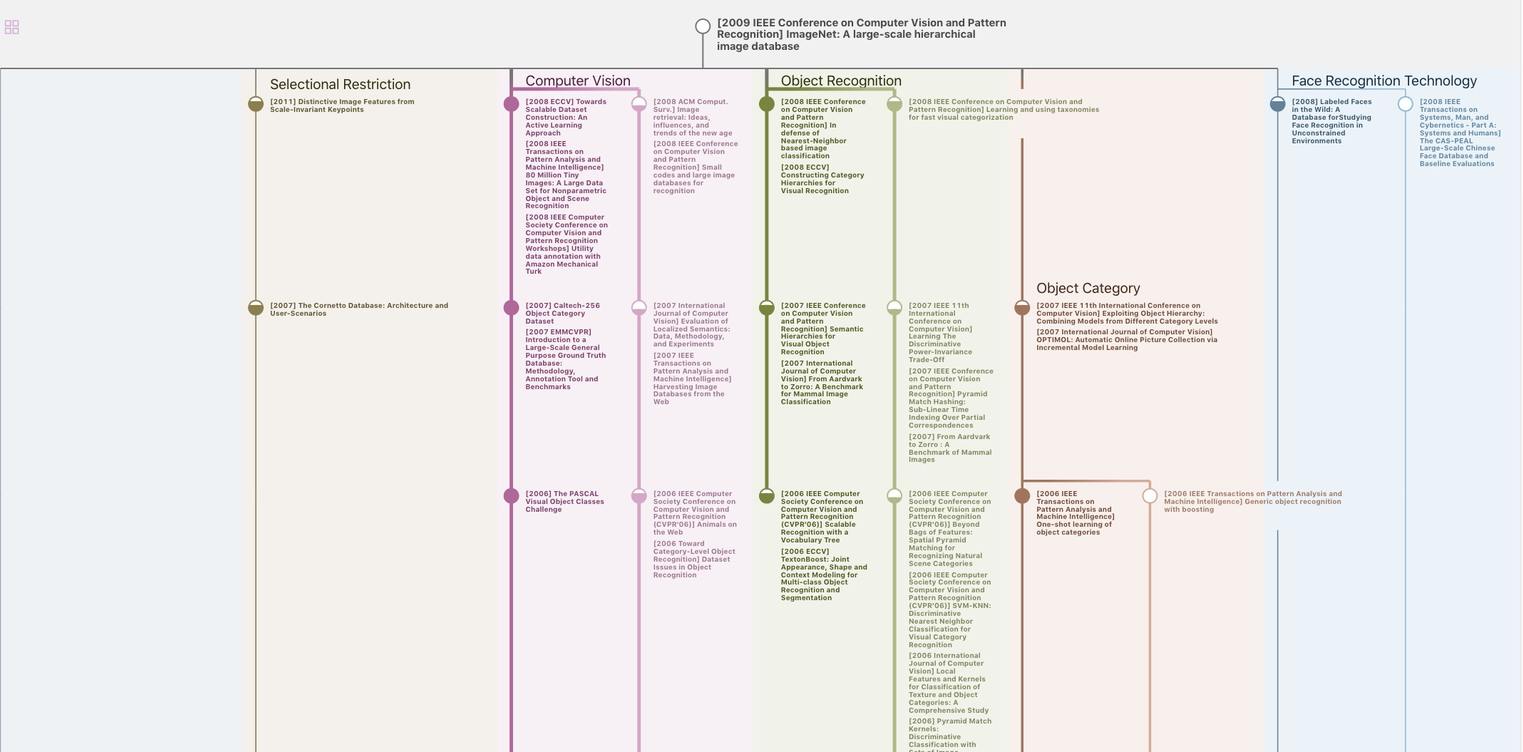
生成溯源树,研究论文发展脉络
Chat Paper
正在生成论文摘要