Self-Supervised Depth Completion for Active Stereo
IEEE ROBOTICS AND AUTOMATION LETTERS(2022)
摘要
Active stereo systems are used in many robotic applications that require 3D information. These depth sensors, however, suffer from stereo artefacts and do not provide dense depth estimates. In this work, we present the first self-supervised depth completion method for active stereo systems that predicts accurate dense depth maps. Our system leverages a feature-based visual inertial SLAM system to produce motion estimates and accurate (but sparse) 3D landmarks. The 3D landmarks are used both as model input and as supervision during training. The motion estimates are used in our novel reconstruction loss that relies on a combination of passive and active stereo frames, resulting in significant improvements in textureless areas that are common in indoor environments. Due to the non-existence of publicly available active stereo datasets, we release a real dataset together with additional information for a publicly available synthetic dataset (TartanAir [30] needed for active depth completion and prediction. Through rigorous evaluations we show that our method outperforms state of the art on both datasets. Additionally we show how our method obtains more complete, and therefore safer, 3D maps when used in a robotic platform.
更多查看译文
关键词
RGB-D perception, data sets for SLAM, sensor fusion, range sensing, visual learning
AI 理解论文
溯源树
样例
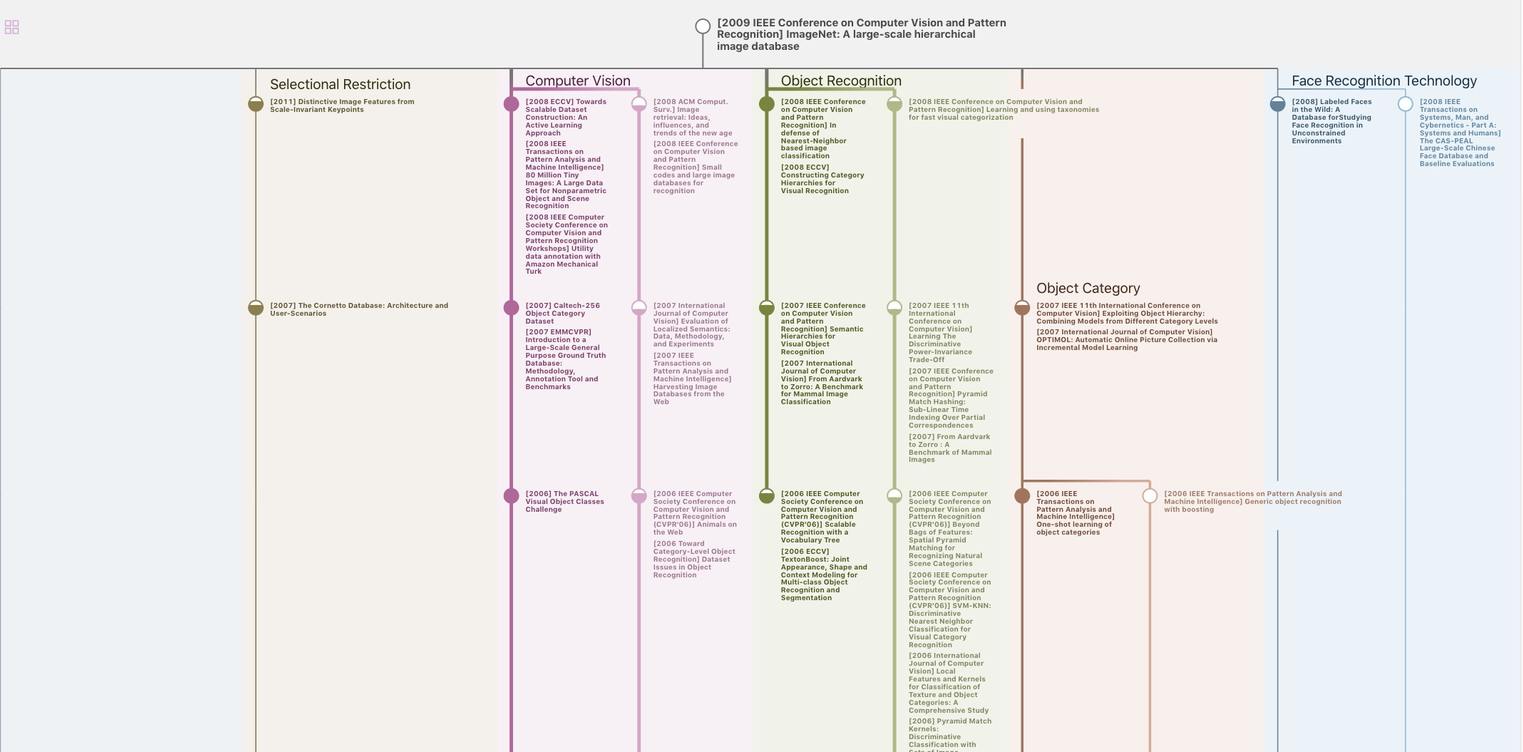
生成溯源树,研究论文发展脉络
Chat Paper
正在生成论文摘要