Fingerprinting Multi-exit Deep Neural Network Models via Inference Time
arxiv(2021)
摘要
Transforming large deep neural network (DNN) models into the multi-exit architectures can overcome the overthinking issue and distribute a large DNN model on resource-constrained scenarios (e.g. IoT frontend devices and backend servers) for inference and transmission efficiency. Nevertheless, intellectual property (IP) protection for the multi-exit models in the wild is still an unsolved challenge. Previous efforts to verify DNN model ownership mainly rely on querying the model with specific samples and checking the responses, e.g., DNN watermarking and fingerprinting. However, they are vulnerable to adversarial settings such as adversarial training and are not suitable for the IP verification for multi-exit DNN models. In this paper, we propose a novel approach to fingerprint multi-exit models via inference time rather than inference predictions. Specifically, we design an effective method to generate a set of fingerprint samples to craft the inference process with a unique and robust inference time cost as the evidence for model ownership. We conduct extensive experiments to prove the uniqueness and robustness of our method on three structures (ResNet-56, VGG-16, and MobileNet) and three datasets (CIFAR-10, CIFAR-100, and Tiny-ImageNet) under comprehensive adversarial settings.
更多查看译文
AI 理解论文
溯源树
样例
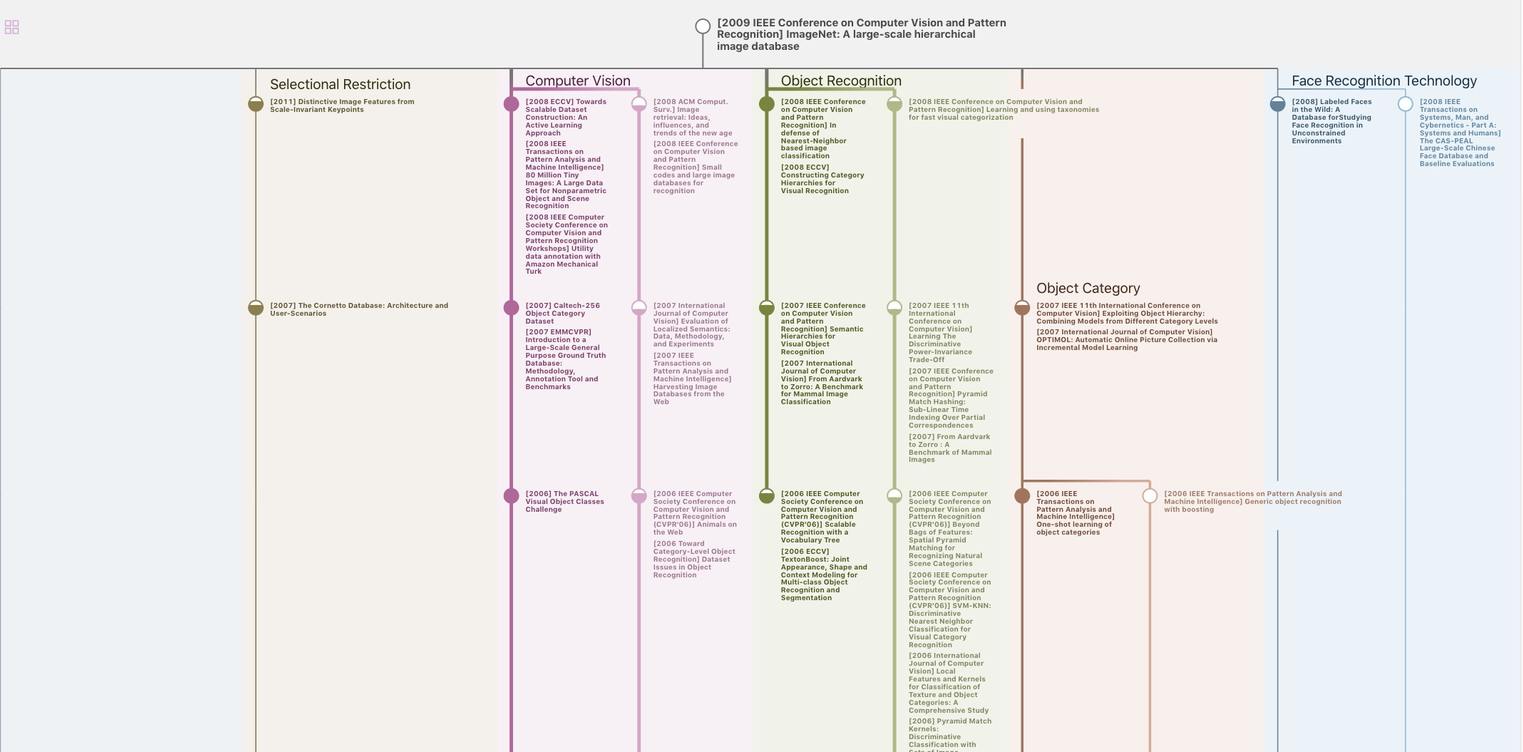
生成溯源树,研究论文发展脉络
Chat Paper
正在生成论文摘要