Active Learning of Markov Decision Processes using Baum-Welch algorithm.
ICMLA(2021)
摘要
Cyber-physical systems (CPSs) are naturally modelled as reactive systems with nondeterministic and probabilistic dynamics. Model-based verification techniques have proved effective in the deployment of safety-critical CPSs. Central for a successful application of such techniques is the construction of an accurate formal model for the system. Manual construction can be a resource-demanding and error-prone process, thus motivating the design of automata learning algorithms to synthesise a system model from observed system behaviours. This paper revisits and adapts the classic Baum-Welch algorithm for learning Markov decision processes and Markov chains. For the case of MDPs, which typically demand more observations, we present a model-based active learning sampling strategy that choses examples which are most informative w.r.t. the current model hypothesis. We empirically compare our approach with state-of-the-art tools and demonstrate that the proposed active learning procedure can significantly reduce the number of observations required to obtain accurate models.
更多查看译文
关键词
Baum-Welch algorithm,Markov decision processes,active learning
AI 理解论文
溯源树
样例
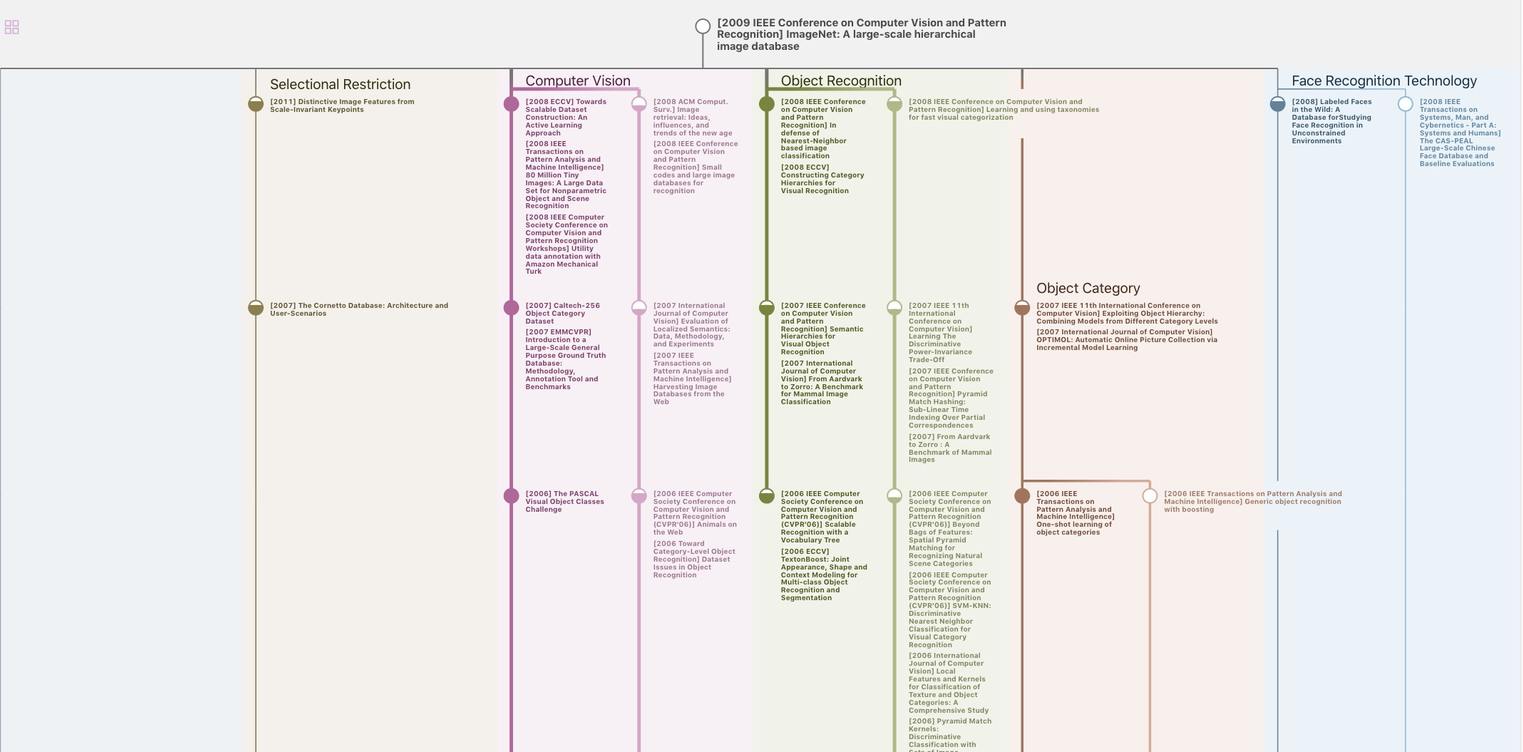
生成溯源树,研究论文发展脉络
Chat Paper
正在生成论文摘要