Foolish Crowds Support Benign Overfitting
arxiv(2022)
摘要
We prove a lower bound on the excess risk of sparse interpolating procedures for linear regression with Gaussian data in the overparameterized regime. We apply this result to obtain a lower bound for basis pursuit (the minimum l(1)-norm interpolant) that implies that its excess risk can converge at an exponentially slower rate than OLS (the minimum l(2)-norm interpolant), even when the ground truth is sparse. Our analysis exposes the benefit of an effect analogous to the "wisdom of the crowd", except here the harm arising from fitting the noise is ameliorated by spreading it among many directions-the variance reduction arises from a foolish crowd.
更多查看译文
关键词
overfitting,benign
AI 理解论文
溯源树
样例
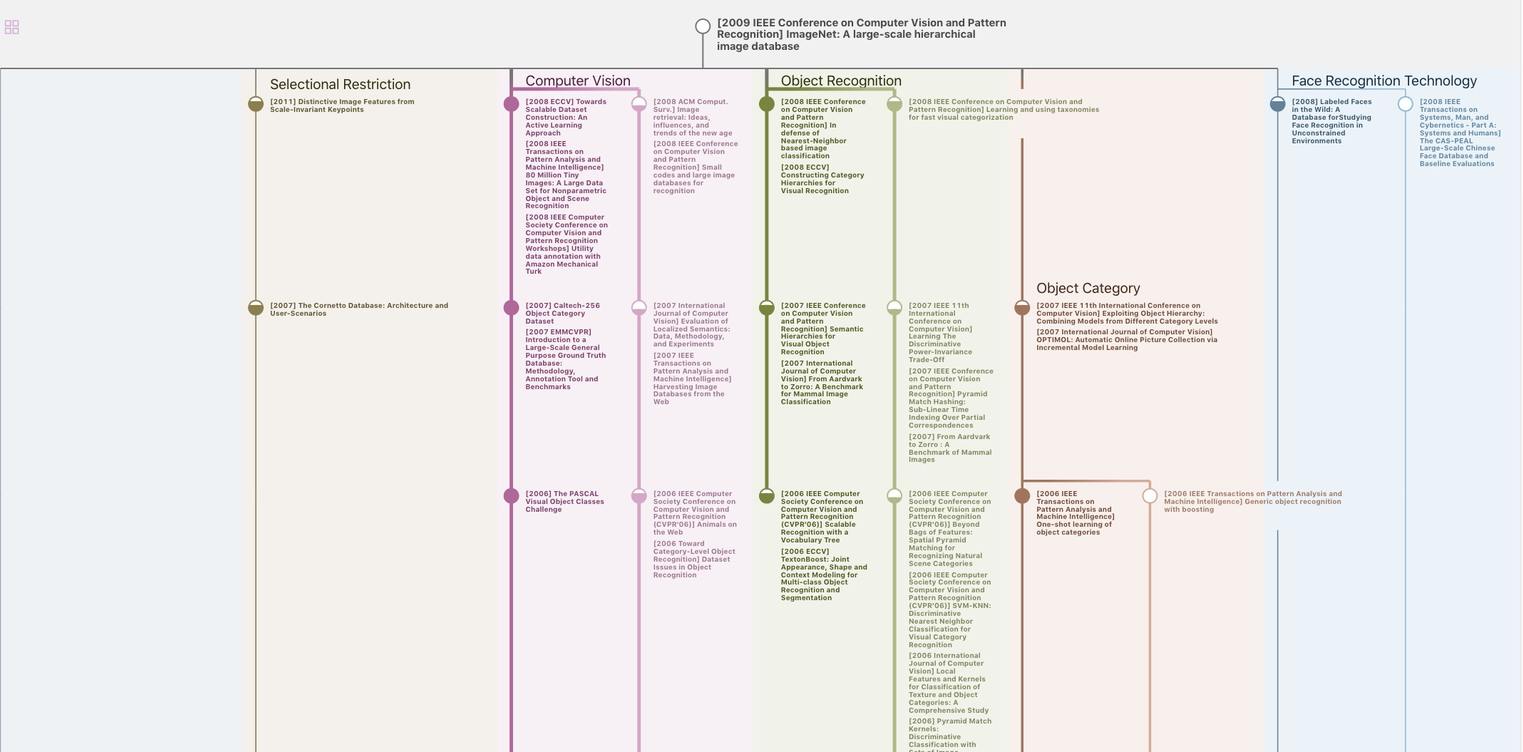
生成溯源树,研究论文发展脉络
Chat Paper
正在生成论文摘要