RC-Struct: A Structure-Based Neural Network Approach for MIMO-OFDM Detection
IEEE Transactions on Wireless Communications(2022)
摘要
In this paper, we introduce a structure-based neural network architecture, namely RC-Struct, for MIMO-OFDM symbol detection. The RC-Struct exploits the temporal structure of the MIMO-OFDM signals through reservoir computing (RC). A binary classifier leverages the repetitive constellation structure in the system to perform multi-class detection. The incorporation of RC allows the RC-Struct to be learned in a purely online fashion with extremely limited pilot symbols in each OFDM subframe. The binary classifier enables the efficient utilization of the precious online training symbols and allows an easy extension to high-order modulations without a substantial increase in complexity. Experiments show that the introduced RC-Struct outperforms both the conventional model-based symbol detection approaches and the state-of-the-art learning-based strategies in terms of bit error rate (BER). The advantages of RC-Struct over existing methods become more significant when rank and link adaptation are adopted. The introduced RC-Struct sheds light on combining communication domain knowledge and learning-based receive processing for 5G/5G-Advanced and Beyond.
更多查看译文
关键词
MIMO-OFDM receive processing,neural networks,structure knowledge,online learning,5G,5G-advanced and QAM constellation
AI 理解论文
溯源树
样例
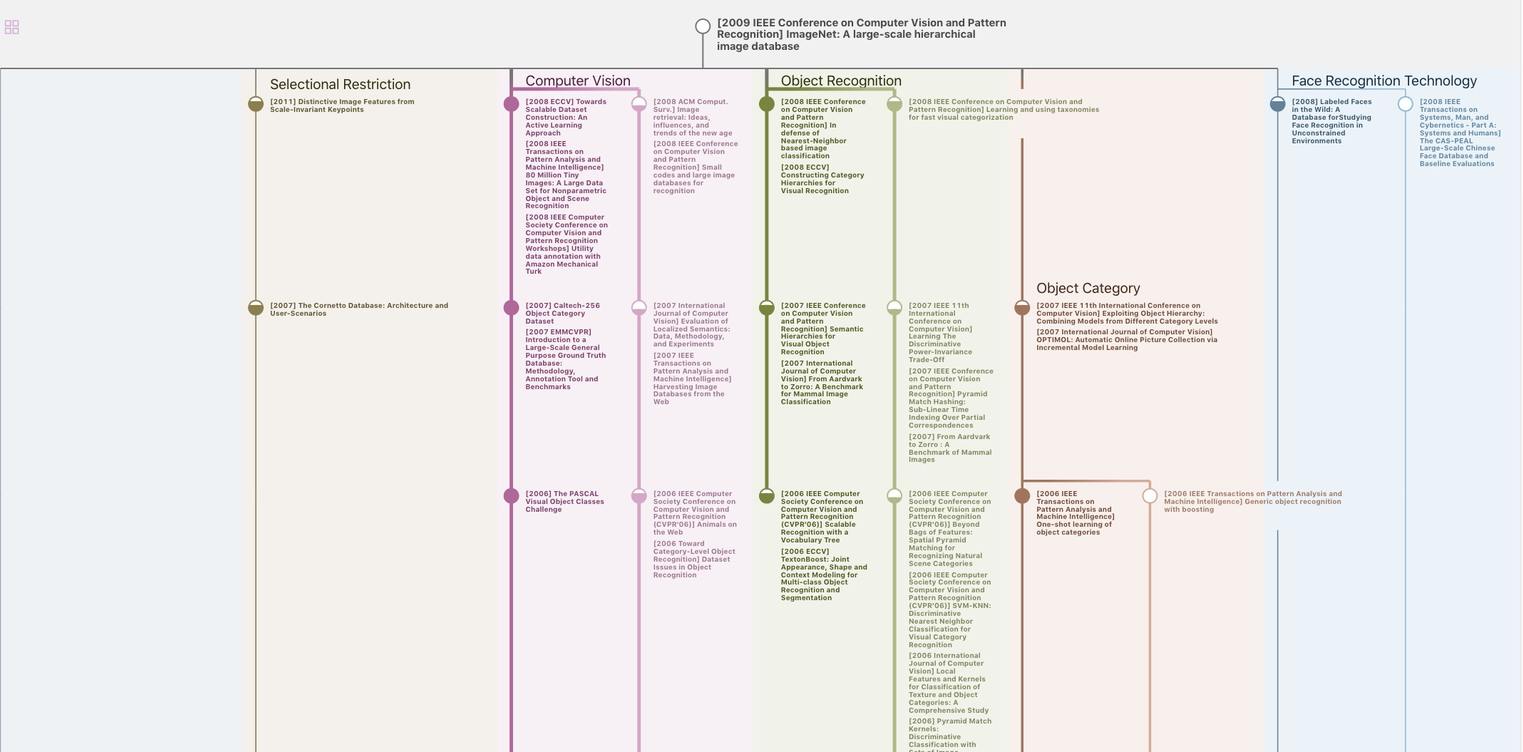
生成溯源树,研究论文发展脉络
Chat Paper
正在生成论文摘要