Sensitivity Of Deep Learning Applied To Spatial Image Steganalysis
PEERJ COMPUTER SCIENCE(2021)
摘要
In recent years, the traditional approach to spatial image steganalysis has shifted to deep learning (DL) techniques, which have improved the detection accuracy while combining feature extraction and classification in a single model, usually a convolutional neural network (CNN). The main contribution from researchers in this area is new architectures that further improve detection accuracy. Nevertheless, the preprocessing and partition of the database influence the overall performance of the CNN. This paper presents the results achieved by novel steganalysis networks (Xu-Net, Ye-Net, Yedroudj-Net, SR-Net, Zhu-Net, and GBRAS-Net) using different combinations of image and filter normalization ranges, various database splits, different activation functions for the preprocessing stage, as well as an analysis on the activation maps and how to report accuracy. These results demonstrate how sensible steganalysis systems are to changes in any stage of the process, and how important it is for researchers in this field to register and report their work thoroughly. We also propose a set of recommendations for the design of experiments in steganalysis with DL.
更多查看译文
关键词
Convolutional neural network, Deep learning, Steganalysis, Steganography, Sensitivity
AI 理解论文
溯源树
样例
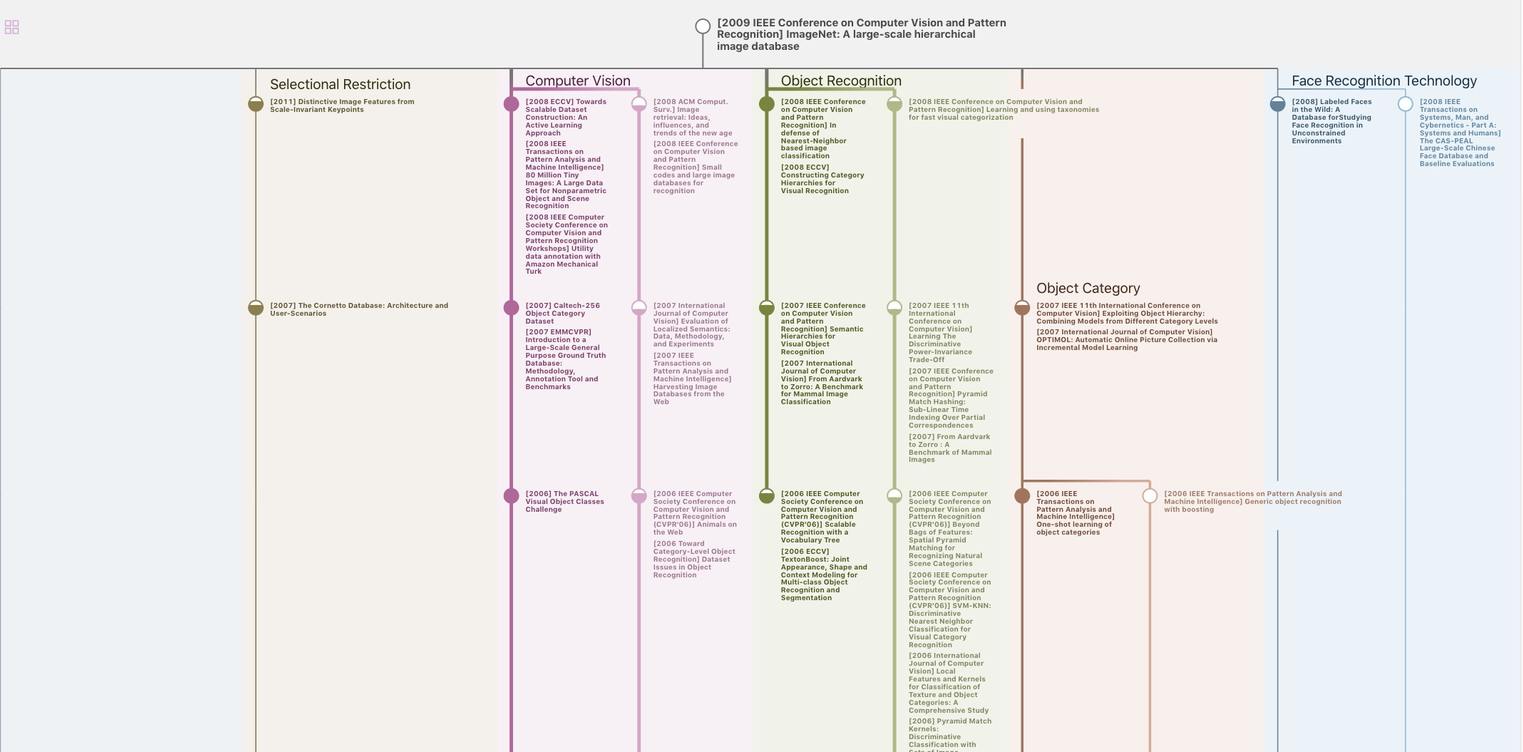
生成溯源树,研究论文发展脉络
Chat Paper
正在生成论文摘要