Learning Grasp Configuration Through Object-Specific Hand Primitives For Posture Planning Of Anthropomorphic Hands
FRONTIERS IN NEUROROBOTICS(2021)
摘要
The proposal of postural synergy theory has provided a new approach to solve the problem of controlling anthropomorphic hands with multiple degrees of freedom. However, generating the grasp configuration for new tasks in this context remains challenging. This study proposes a method to learn grasp configuration according to the shape of the object by using postural synergy theory. By referring to past research, an experimental paradigm is first designed that enables the grasping of 50 typical objects in grasping and operational tasks. The angles of the finger joints of 10 subjects were then recorded when performing these tasks. Following this, four hand primitives were extracted by using principal component analysis, and a low-dimensional synergy subspace was established. The problem of planning the trajectories of the joints was thus transformed into that of determining the synergy input for trajectory planning in low-dimensional space. The average synergy inputs for the trajectories of each task were obtained through the Gaussian mixture regression, and several Gaussian processes were trained to infer the inputs trajectories of a given shape descriptor for similar tasks. Finally, the feasibility of the proposed method was verified by simulations involving the generation of grasp configurations for a prosthetic hand control. The error in the reconstructed posture was compared with those obtained by using postural synergies in past work. The results show that the proposed method can realize movements similar to those of the human hand during grasping actions, and its range of use can be extended from simple grasping tasks to complex operational tasks.
更多查看译文
关键词
grasping, anthropomorphic hand, postural synergy, dimension reduction, Gaussian mixture regression
AI 理解论文
溯源树
样例
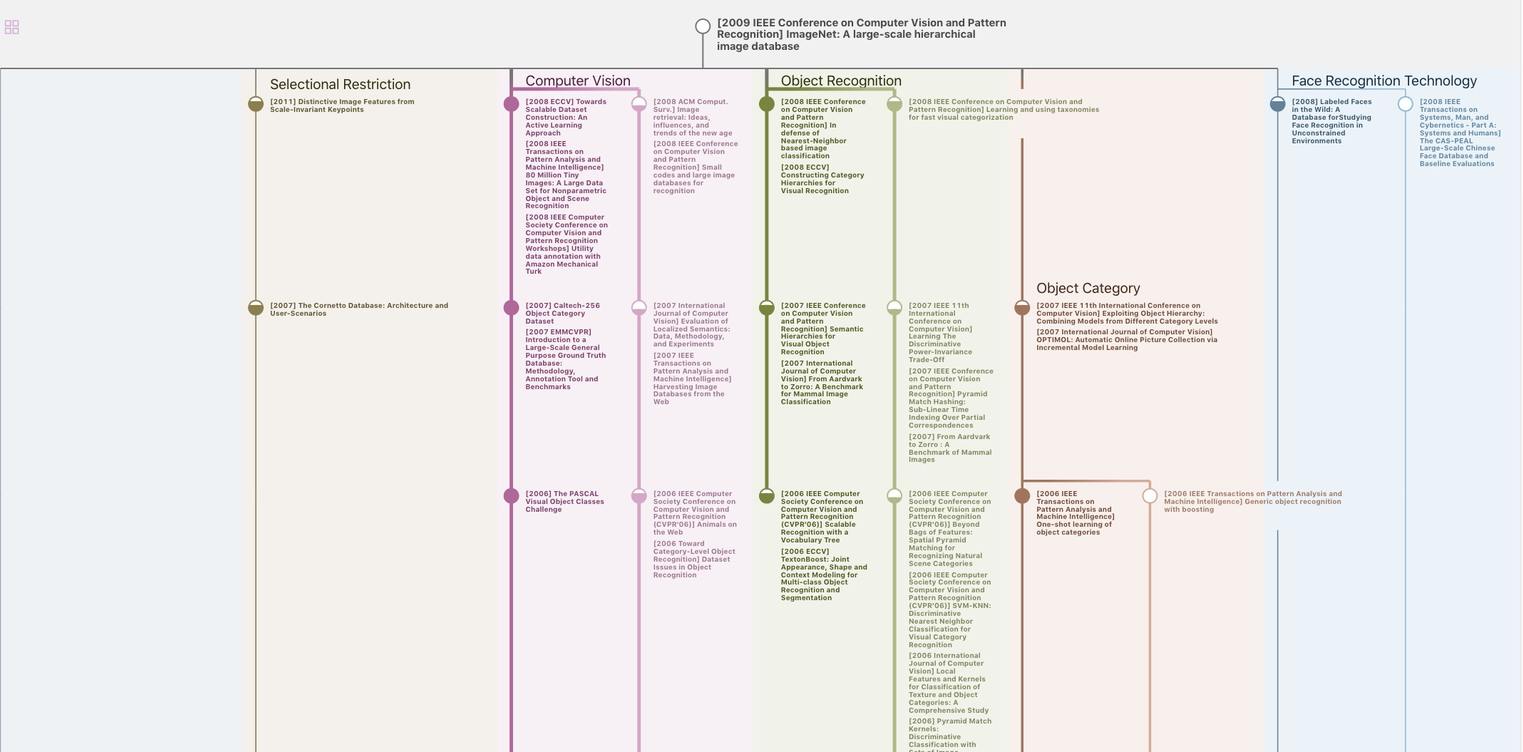
生成溯源树,研究论文发展脉络
Chat Paper
正在生成论文摘要