Dropout Q-Functions for Doubly Efficient Reinforcement Learning
International Conference on Learning Representations (ICLR)(2022)
摘要
Randomized ensemble double Q-learning (REDQ) has recently achieved state-of-the-art sample efficiency on continuous-action reinforcement learning benchmarks. This superior sample efficiency is possible by using a large Q-function ensemble. However, REDQ is much less computationally efficient than non-ensemble counterparts such as Soft Actor-Critic (SAC). To make REDQ more computationally efficient, we propose a method of improving computational efficiency called Dr.Q, which is a variant of REDQ that uses a small ensemble of dropout Q-functions. Our dropout Q-functions are simple Q-functions equipped with dropout connection and layer normalization. Despite its simplicity of implementation, our experimental results indicate that Dr.Q is doubly (sample and computationally) efficient. It achieved comparable sample efficiency with REDQ and much better computational efficiency than REDQ and comparable computational efficiency with that of SAC.
更多查看译文
关键词
Reinforcement learning
AI 理解论文
溯源树
样例
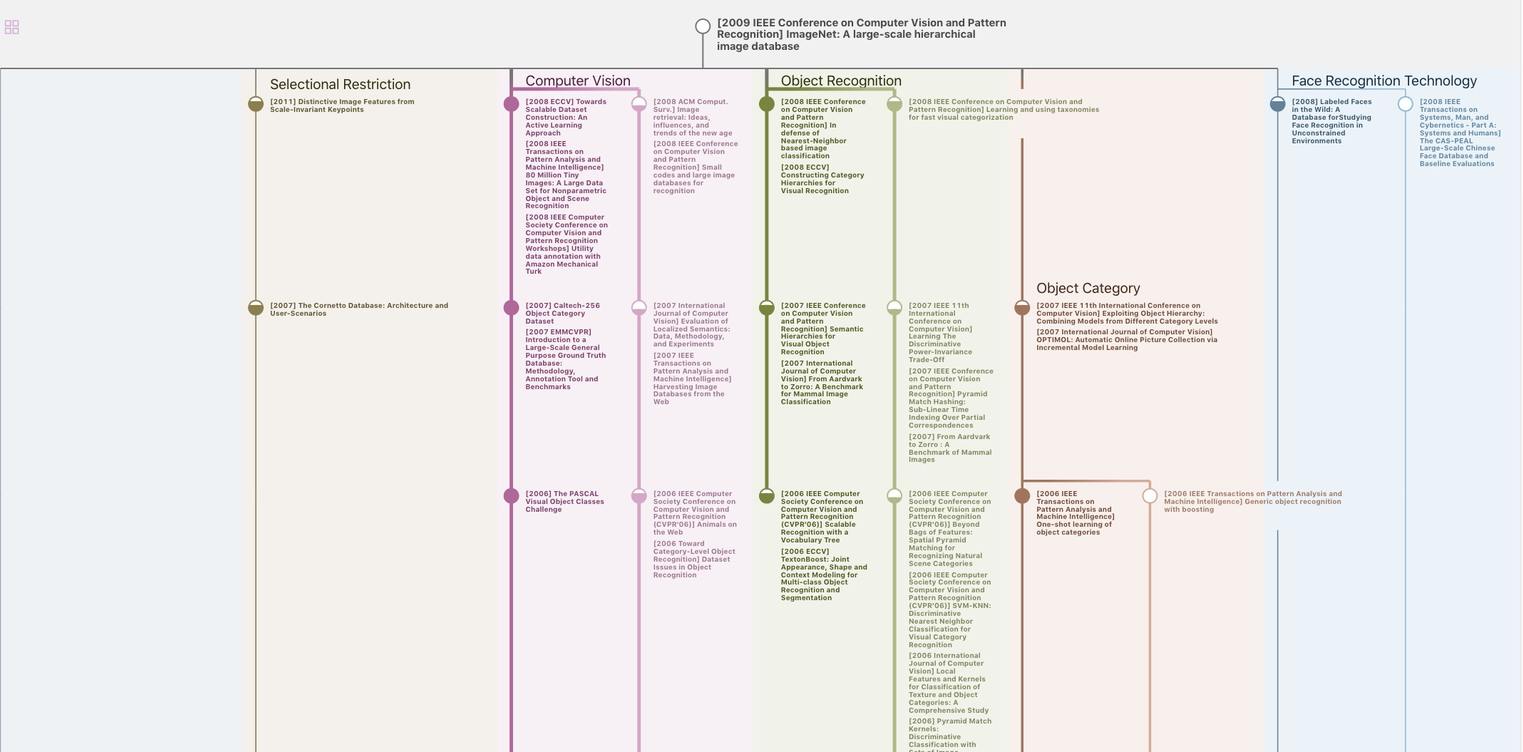
生成溯源树,研究论文发展脉络
Chat Paper
正在生成论文摘要