Differentiable Equilibrium Computation with Decision Diagrams for Stackelberg Models of Combinatorial Congestion Games.
Annual Conference on Neural Information Processing Systems(2021)
摘要
We address Stackelberg models of combinatorial congestion games (CCGs); we aim to optimize the parameters of CCGs so that the selfish behavior of non-atomic players attains desirable equilibria. This model is essential for designing such social infrastructures as traffic and communication networks. Nevertheless, computational approaches to the model have not been thoroughly studied due to two difficulties: (I) bilevel-programming structures and (II) the combinatorial nature of CCGs. We tackle them by carefully combining (I) the idea of \textit{differentiable} optimization and (II) data structures called \textit{zero-suppressed binary decision diagrams} (ZDDs), which can compactly represent sets of combinatorial strategies. Our algorithm numerically approximates the equilibria of CCGs, which we can differentiate with respect to parameters of CCGs by automatic differentiation. With the resulting derivatives, we can apply gradient-based methods to Stackelberg models of CCGs. Our method is tailored to induce Nesterov's acceleration and can fully utilize the empirical compactness of ZDDs. These technical advantages enable us to deal with CCGs with a vast number of combinatorial strategies. Experiments on real-world network design instances demonstrate the practicality of our method.
更多查看译文
关键词
combinatorial congestion games,stackelberg models,differentiable equilibrium computation,decision diagrams
AI 理解论文
溯源树
样例
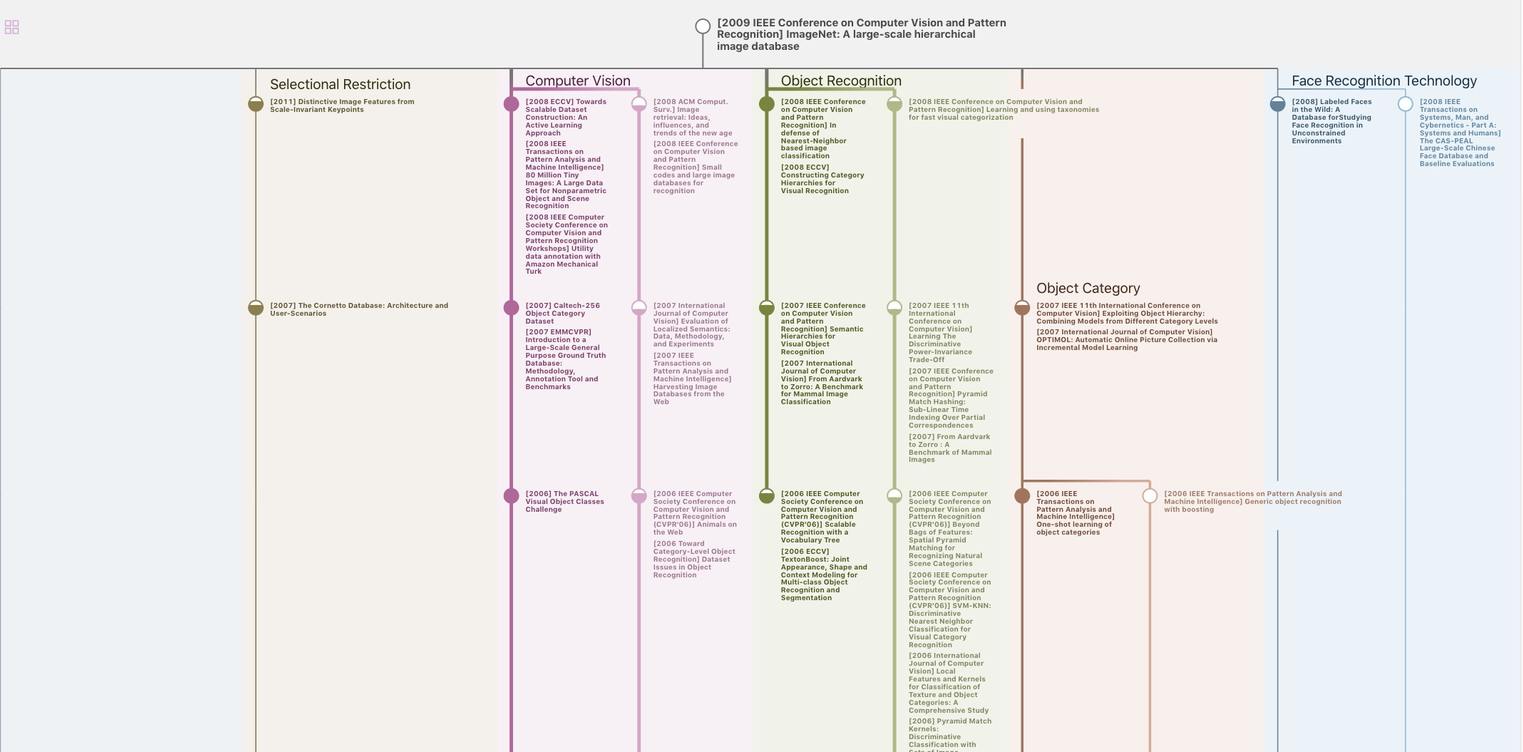
生成溯源树,研究论文发展脉络
Chat Paper
正在生成论文摘要