A general framework for formulating structured variable selection
arxiv(2021)
摘要
In variable selection, a selection rule that prescribes the permissible sets
of selected variables (called a "selection dictionary") is desirable due to the
inherent structural constraints among the candidate variables. Such selection
rules can be complex in real-world data analyses, and failing to incorporate
such restrictions could not only compromise the interpretability of the model
but also lead to decreased prediction accuracy. However, no general framework
has been proposed to formalize selection rules and their applications, which
poses a significant challenge for practitioners seeking to integrate these
rules into their analyses. In this work, we establish a framework for
structured variable selection that can incorporate universal structural
constraints. We develop a mathematical language for constructing arbitrary
selection rules, where the selection dictionary is formally defined. We
demonstrate that all selection rules can be expressed as combinations of
operations on constructs, facilitating the identification of the corresponding
selection dictionary. Once this selection dictionary is derived, practitioners
can apply their own user-defined criteria to select the optimal model.
Additionally, our framework enhances existing penalized regression methods for
variable selection by providing guidance on how to appropriately group
variables to achieve the desired selection rule. Furthermore, our innovative
framework opens the door to establishing new l0 norm-based penalized regression
techniques that can be tailored to respect arbitrary selection rules, thereby
expanding the possibilities for more robust and tailored model development.
更多查看译文
关键词
permissible variable subsets
AI 理解论文
溯源树
样例
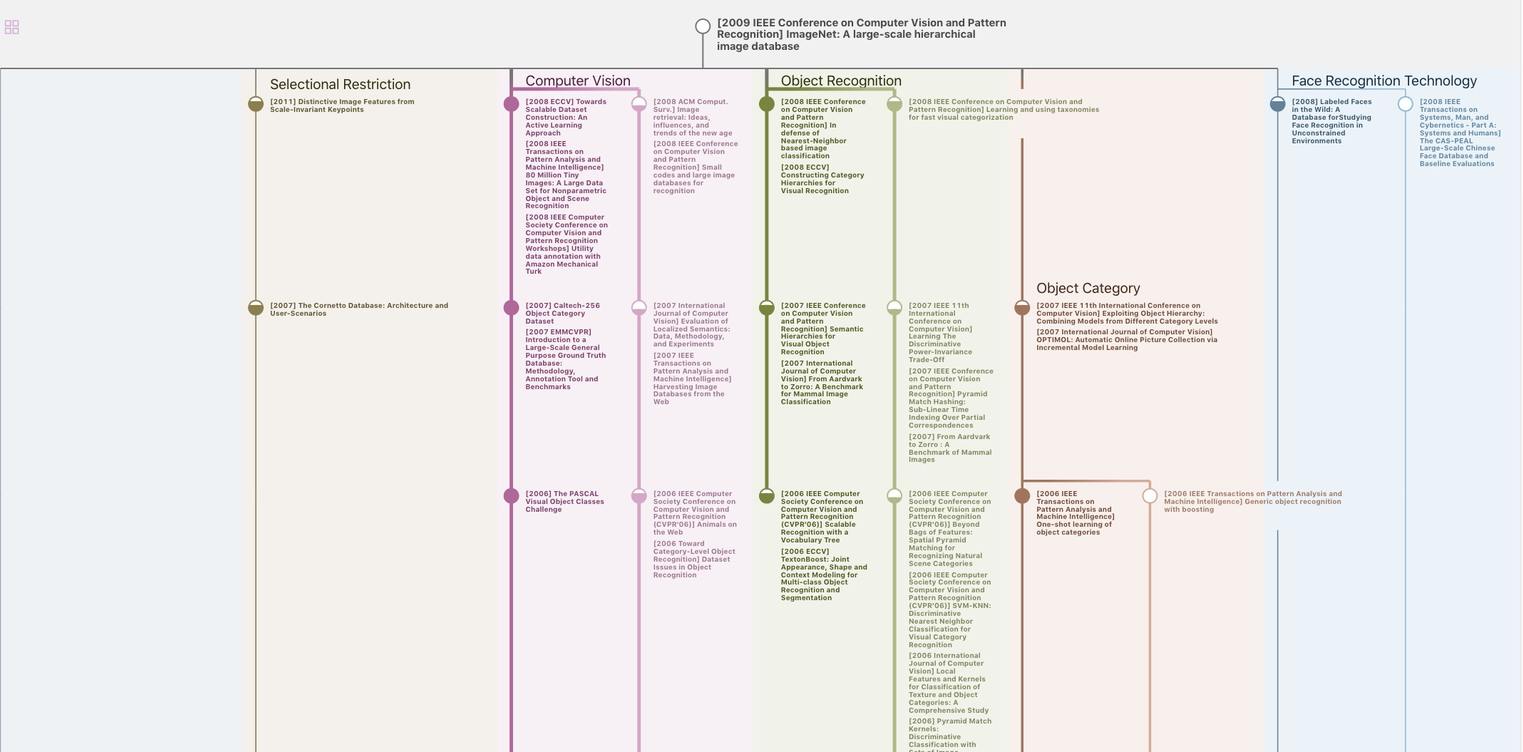
生成溯源树,研究论文发展脉络
Chat Paper
正在生成论文摘要