A Fast Domain Adaptation Network for Image Super-Resolution
IMAGE AND GRAPHICS (ICIG 2021), PT III(2021)
摘要
Most previous super-resolution (SR) methods are based on high-resolution (HR) images and corresponding low-resolution (LR) images obtained artificially through bicubic downsampling. However, in real scenes, LR images are usually obtained with complex degradation functions, which may result in the domain gap between LR images. And we observe that this can sharply weaken the performance of the SR model. In this work, we propose a Fast Domain Adaptation Network for SR to solve this issue. First, we train a domain adaptation module to transform source LR images to the bicubic downsampled LR images. Then, we apply this module on the top of any SR model pretrained on bicubically downsampled images. Abundant experiments demonstrate the effectiveness of our proposed method and show that our network outperforms previous state-of-the-art works in terms of both qualitative and quantitative aspects on real-world dataset and synthetic dataset.
更多查看译文
关键词
Image super-resolution, Domain adaptation, Deep learning
AI 理解论文
溯源树
样例
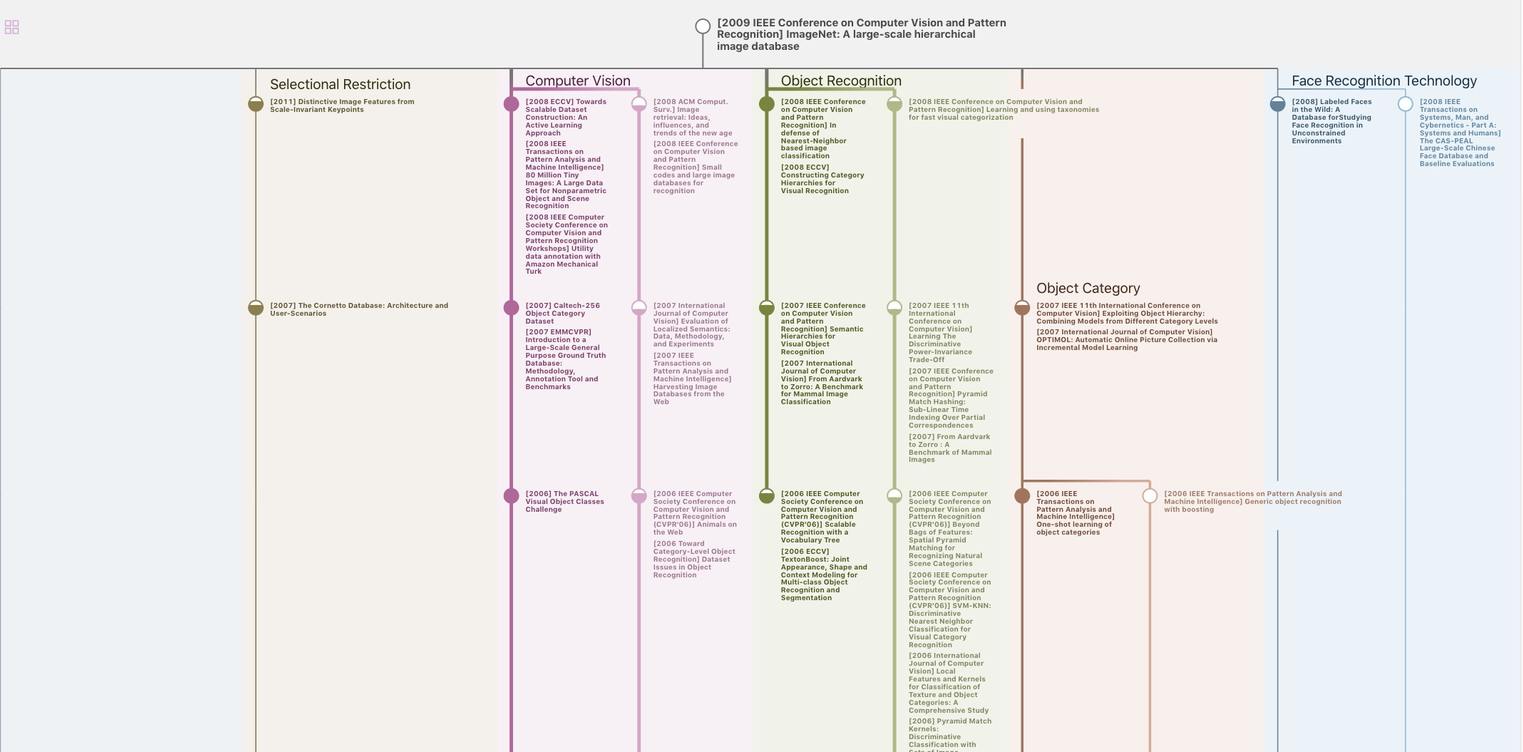
生成溯源树,研究论文发展脉络
Chat Paper
正在生成论文摘要