Prediction of Carbon Nanostructure Mechanical Properties and Role of Defects Using Machine Learning
arxiv(2021)
摘要
Carbon fiber and graphene-based nanostructures such as carbon nanotubes (CNTs) and defective structures have extraordinary potential as strong and lightweight materials. A longstanding bottleneck has been lack of understanding and implementation of atomic-scale engineering to harness the theoretical limits of modulus and tensile strength, of which only a fraction is routinely reached today. Here we demonstrate accurate and fast predictions of mechanical properties for CNTs and arbitrary 3D graphitic assemblies based on a training set of over 1000 stress-strain curves from cutting-edge reactive MD simulation and machine learning (ML). Several ML methods are compared and show that our newly proposed hierarchically structured graph neural networks with spatial information (HS-GNNs) achieve predictions in modulus and strength for any 3D nanostructure with only 5-10% error across a wide range of possible values. The reliability is sufficient for practical applications and a great improvement over off-the shelf ML methods with up to 50% deviation, as well as over earlier models for specific chemistry with 20% deviation. The algorithms allow more than 100 times faster mechanical property predictions than traditional molecular dynamics simulations, the identification of the role of defects and random 3D morphology, and high-throughput screening of 3D structures for enhanced mechanical properties. The algorithms can be scaled to morphologies up to 100 nm in size, expanded for chemically similar compounds, and trained to predict a broader range of properties.
更多查看译文
关键词
carbon nanostructure mechanical properties,machine learning,mechanical properties,prediction
AI 理解论文
溯源树
样例
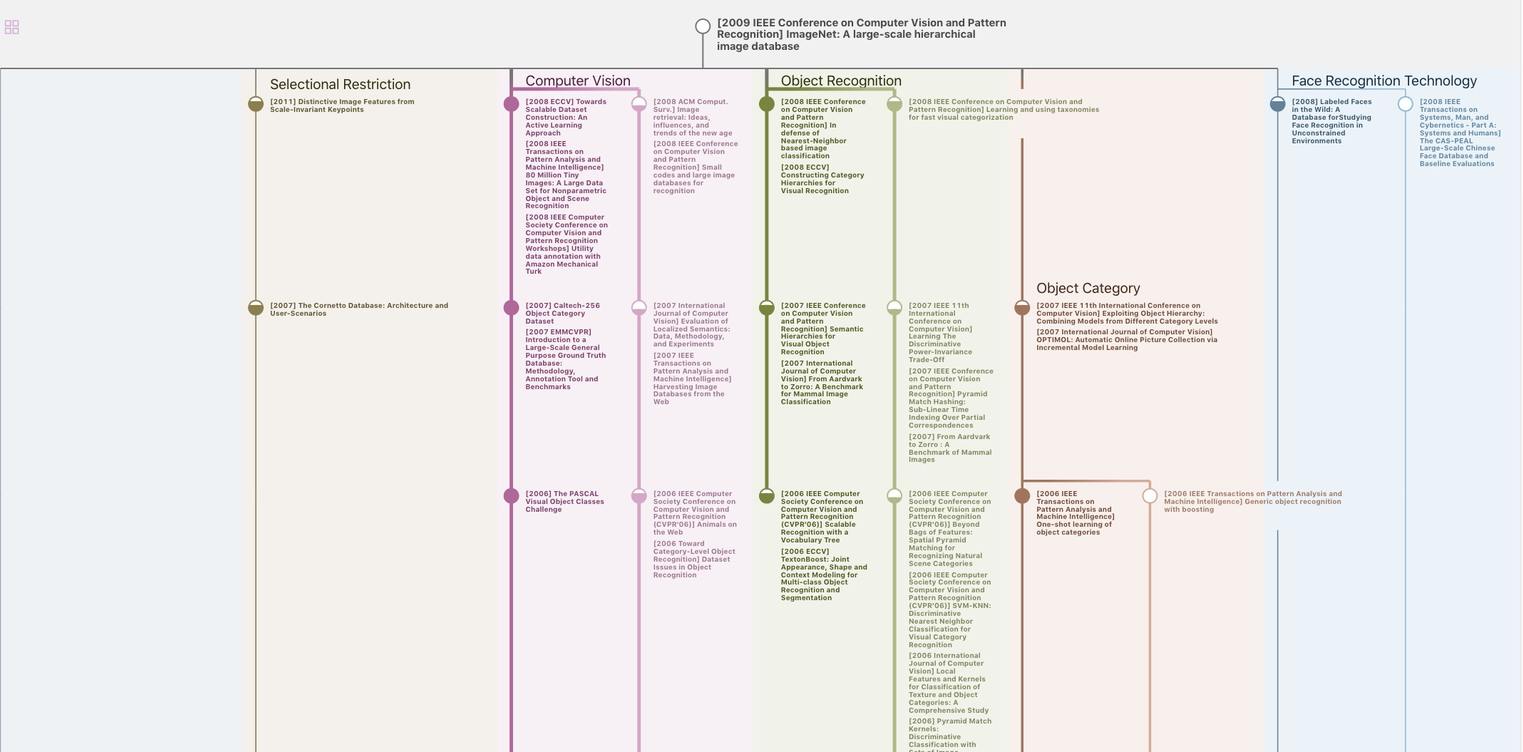
生成溯源树,研究论文发展脉络
Chat Paper
正在生成论文摘要