Unsupervised Belief Representation Learning with Information-Theoretic Variational Graph Auto-Encoders
SIGIR '22: Proceedings of the 45th International ACM SIGIR Conference on Research and Development in Information Retrieval(2022)
摘要
This paper develops a novel unsupervised algorithm for belief representation learning in polarized networks that (i) uncovers the latent dimensions of the underlying belief space and (ii) jointly embeds users and content items (that they interact with) into that space in a manner that facilitates a number of downstream tasks, such as stance detection, stance prediction, and ideology mapping. Inspired by total correlation in information theory, we propose the Information-Theoretic Variational Graph Auto-Encoder (InfoVGAE) that learns to project both users and content items (e.g., posts that represent user views) into an appropriate disentangled latent space. To better disentangle latent variables in that space, we develop a total correlation regularization module, a Proportional-Integral (PI) control module, and adopt rectified Gaussian distribution to ensure the orthogonality. The latent representation of users and content can then be used to quantify their ideological leaning and detect/predict their stances on issues. We evaluate the performance of the proposed InfoVGAE on three real-world datasets, of which two are collected from Twitter and one from U.S. Congress voting records. The evaluation results show that our model outperforms state-of-the-art unsupervised models by reducing 10.5% user clustering errors and achieving 12.1% higher F1 scores for stance separation of content items. In addition, InfoVGAE produces a comparable result with supervised models. We also discuss its performance on stance prediction and user ranking within ideological groups.
更多查看译文
关键词
Unsupervised Representation Learning, Polarized Networks, Ideology Analysis, Variational Graph Auto-Encoders
AI 理解论文
溯源树
样例
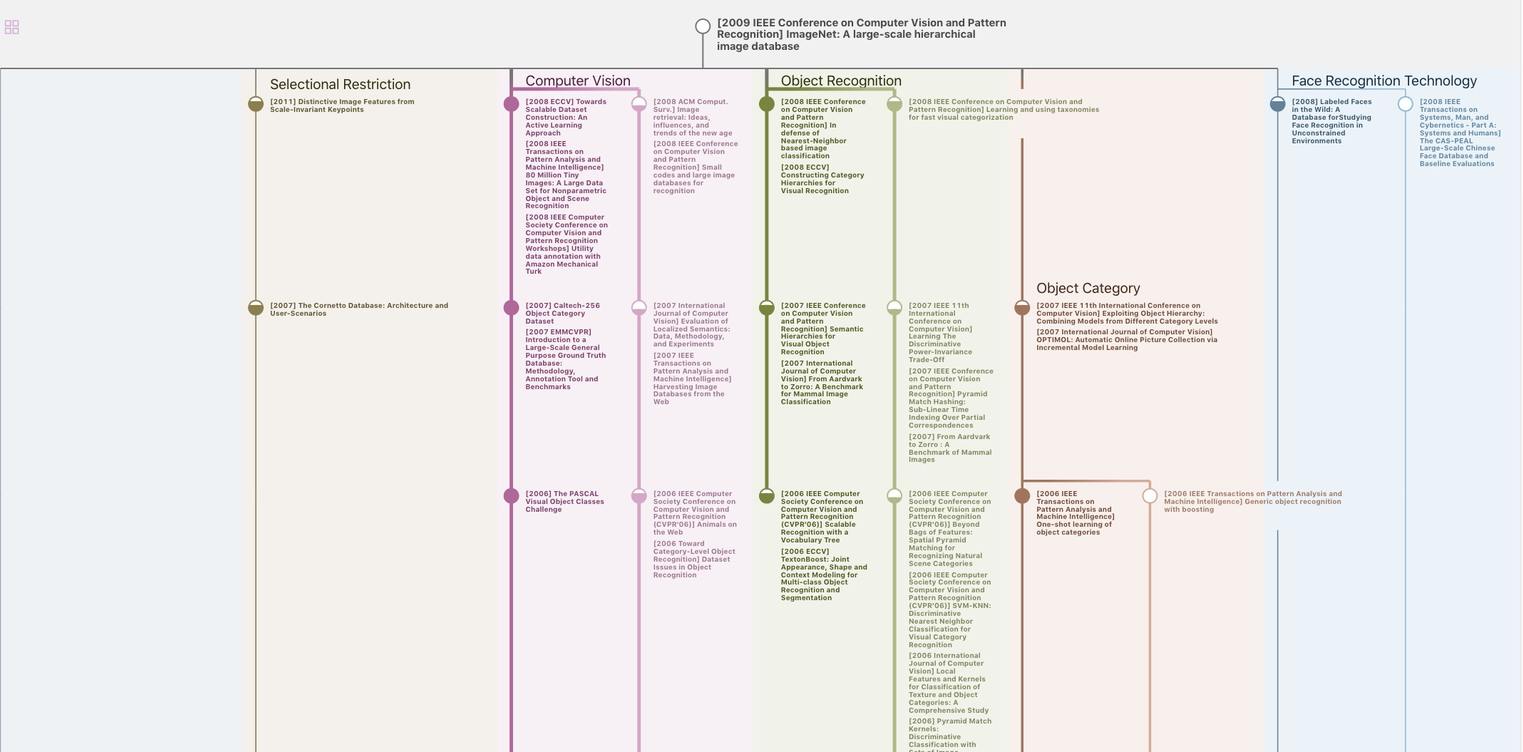
生成溯源树,研究论文发展脉络
Chat Paper
正在生成论文摘要