Jumping Particle Swarm Optimization
PROCEEDINGS OF SIXTH INTERNATIONAL CONGRESS ON INFORMATION AND COMMUNICATION TECHNOLOGY (ICICT 2021), VOL 2(2022)
摘要
Classical Particle Swarm Optimization (PSO) has limitations of slow convergence rate and getting trapped in a local optimum solution, when the data dimensions are high. It is therefore important to propose an algorithm that has an ability to overcome the limitations of classical PSO. Keeping in view the above mentioned limitations, this paper proposes a variant of classical PSO that has an ability to overcome the problem of slow convergence and skipping the local optimum solution. The proposed algorithm is based on a jumping strategy which triggers the particles to jump whenever they are found stuck in a local optimum solution. The proposed jumping strategy in PSO not only enables the algorithm to skip the local optima but also enables it to converge at a faster rate. The effectiveness of the proposed jumping strategy is demonstrated by performing experiments on a benchmark dataset that contains both the unimodal and the multimodal test functions.
更多查看译文
关键词
Global optimization, Large scale optimization, Metaheuristics, Particle swarm optimization, Unimodal and multimodal functions
AI 理解论文
溯源树
样例
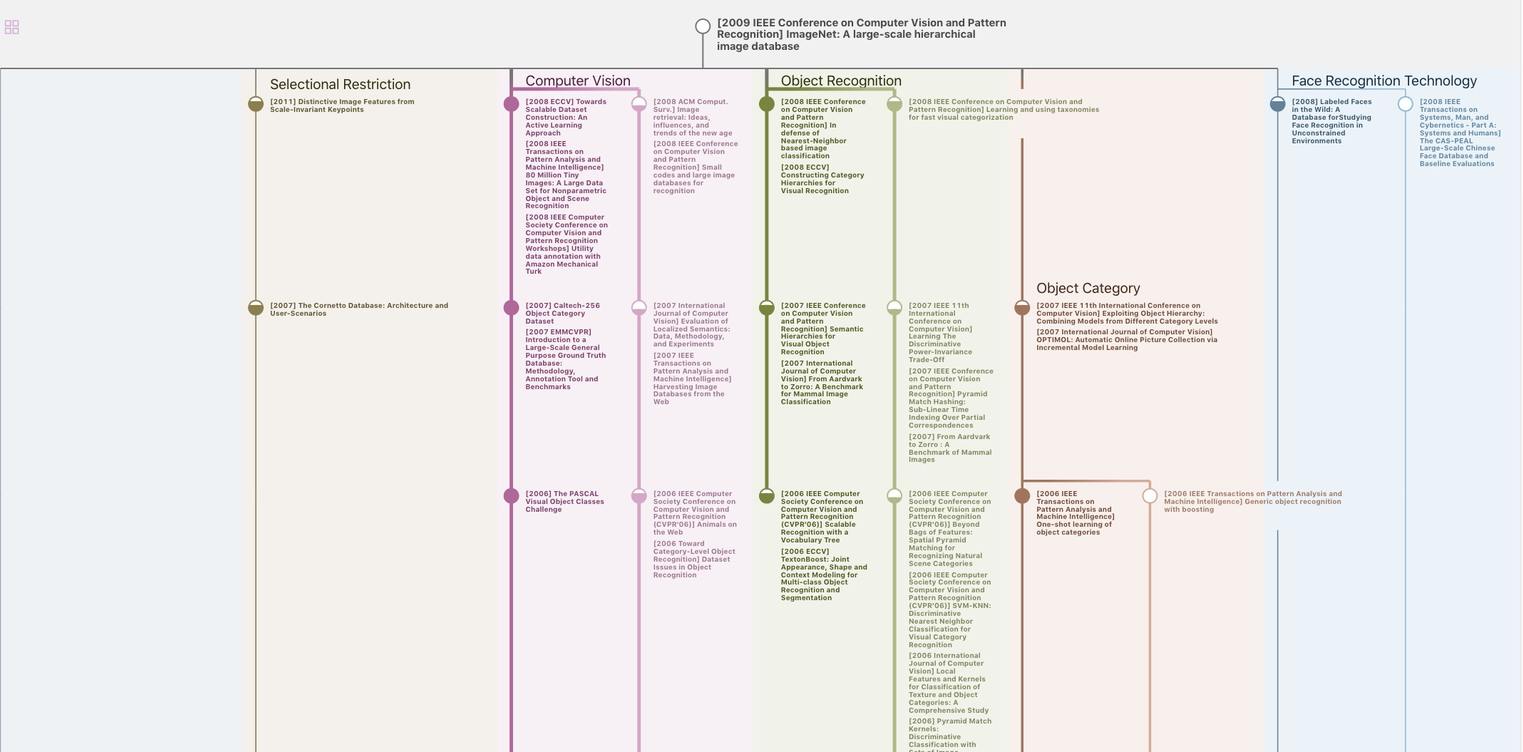
生成溯源树,研究论文发展脉络
Chat Paper
正在生成论文摘要