Joint Distribution Adaptation via Wasserstein Adversarial Training
2021 INTERNATIONAL JOINT CONFERENCE ON NEURAL NETWORKS (IJCNN)(2021)
摘要
This paper considers the unsupervised domain adaptation problem, in which we want to find a good prediction function on the unlabeled target domain, by utilizing the information provided in the labeled source domain. A common approach to the domain adaptation problem is to learn a representation space where the distributional discrepancy of the source and target domains is small. Existing methods generally tend to match the marginal distributions of the two domains, while the label information in the source domain is not fully exploited. In this paper, we propose a representation learning approach for domain adaptation, which is addressed as JODAWAT. We aim to adapt the joint distributions of the feature-label pairs in the shared representation space for both domains. In particular, we minimize the Wasserstein distance between the source and target domains, while the prediction performance on the source domain is also guaranteed. The proposed approach results in a minimax adversarial training procedure that incorporates a novel split gradient penalty term. A generalization bound on the target domain is provided to reveal the efficacy of representation learning for joint distribution adaptation. We conduct extensive evaluations on JODAWAT, and test its classification accuracy on multiple synthetic and real datasets. The experimental results justify that our proposed method is able to achieve superior performance compared with various domain adaptation methods.
更多查看译文
关键词
domain adaptation, transfer learning, adversarial training, Wasserstein distance, generalization error bound
AI 理解论文
溯源树
样例
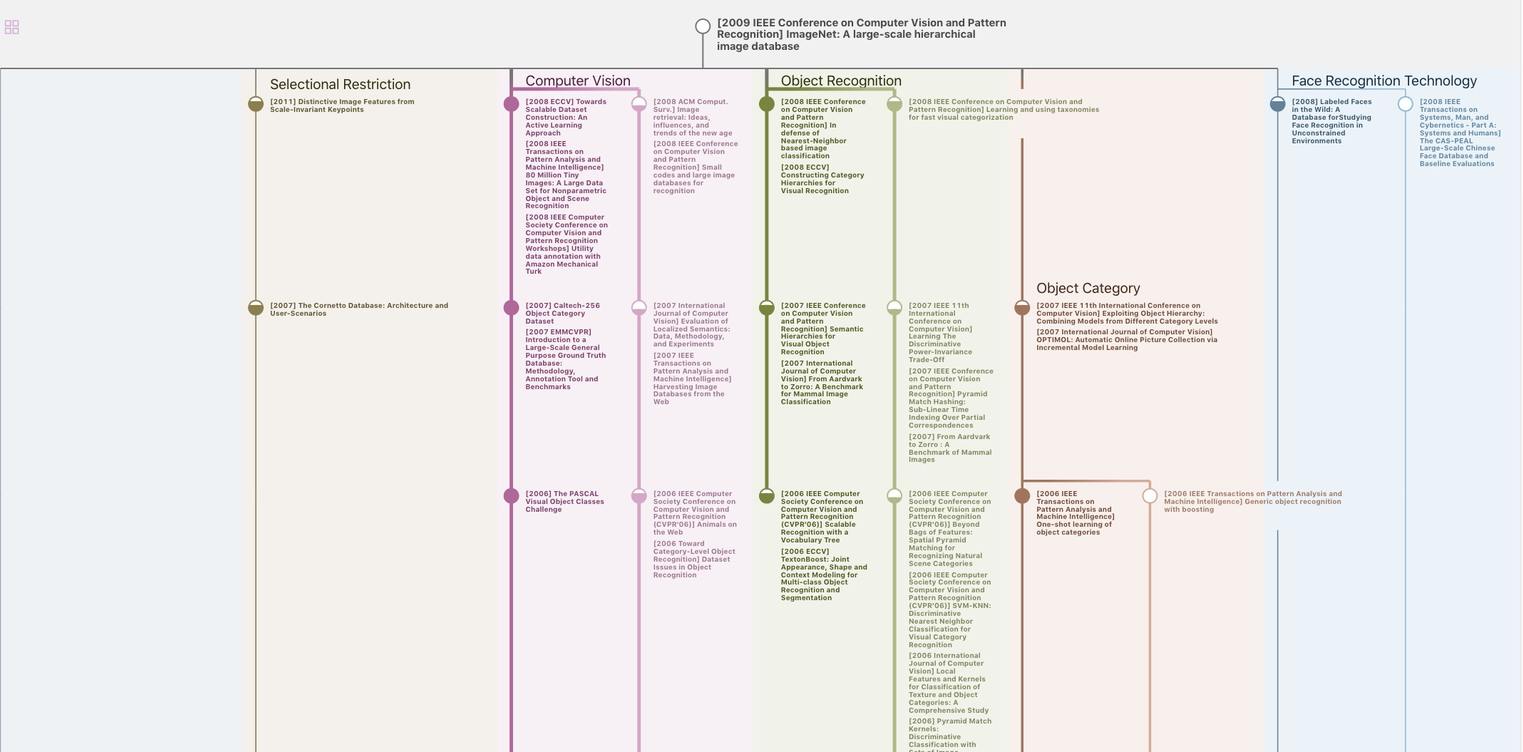
生成溯源树,研究论文发展脉络
Chat Paper
正在生成论文摘要