Private FLI: Anti-Gradient Leakage Recovery Data Privacy Architecture
2021 INTERNATIONAL JOINT CONFERENCE ON NEURAL NETWORKS (IJCNN)(2021)
摘要
While machine learning brings convenience, it also faces the issue of data privacy. For privacy issues, most researches focus on implementing homomorphic encryption or differential privacy to protect data, while ignoring the potential threats caused by the leakage of model parameters. However, a malicious attacker can still recover sensitive data information through model parameters. On the one hand, traditional methods cannot take both high accuracy and low computation time into account. On the other hand, they cannot resist the reconstruction attack from the model's parameter. In order to address this problem, this paper designs a privacy protection framework named FLI, which is inspired by public key infrastructure. In FLI, all participants and the server are trained and aggregated under one framework based on federated learning, which includes key exchange and shares with the idea of homomorphic encryption. Under the algorithm we design, the malicious adversary cannot recover effective information after obtaining the transformed parameters, while the server can still perform effective parameter aggregation. To evaluate the performance of FLI, we conduct extensive experiments. The experimental results show that the computation time is within an acceptable range while ensuring high accuracy.
更多查看译文
关键词
private FLI,anti-gradient leakage recovery data privacy architecture,machine learning,privacy issues,homomorphic encryption,differential privacy,model parameters,malicious attacker,sensitive data information,low computation time,reconstruction attack,privacy protection framework,public key infrastructure,federated learning,key exchange,transformed parameters,effective parameter aggregation
AI 理解论文
溯源树
样例
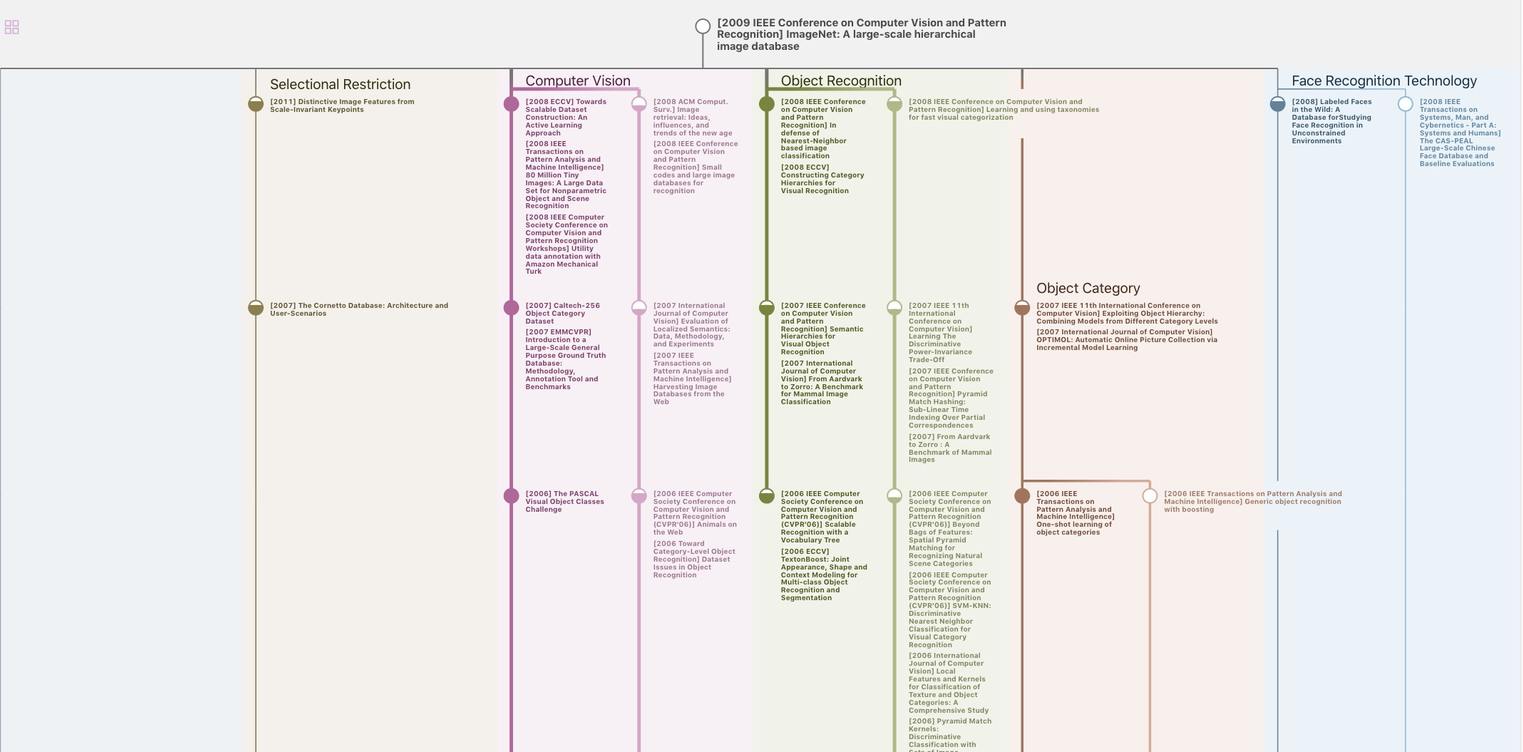
生成溯源树,研究论文发展脉络
Chat Paper
正在生成论文摘要