Improving Meta-Learning Classification with Prototype Enhancement
2021 INTERNATIONAL JOINT CONFERENCE ON NEURAL NETWORKS (IJCNN)(2021)
摘要
Meta-learning classification is about training a classifier by remembering some common knowledge from limited labeled examples in a support set. It has attracted more and more attention in machine learning community. Despite the success, existing Meta-learning algorithms usually ignore the relationship between the samples of the support set and the samples of the query set in a task after feature extraction and thus fail to obtain the most discriminative features, which leads to an unreliable class prototype and a low distinguishability of feature representations. In this paper, we propose a novel metric-based meta-learning algorithm called Prototype Enhancement Network (PEN) to tackle the above problems. The proposed algorithm uses: (1) a Task Context-Related (TCR) Module to obtain a more discriminative and context-related feature representation of samples within a task; and (2) a Prototype Reset (PR) module to expands the support set with partially unlabeled query set samples to mitigate the low-data problem and obtain a more representative class prototype. The cooperation of the TCR module and the PR module effectively enhance the reliability and the accuracy of the class prototype. Experiments are conducted on two classification benchmarks (mini-ImageNet and tiered-ImageNet) and the results confirm the effectiveness of PEN compared with other state-of-the-art methods.
更多查看译文
关键词
Meta-learning classification,support set,Meta-learning algorithms,feature extraction,discriminative features,unreliable class prototype,feature representations,novel metric-based meta-learning algorithm,Prototype Enhancement Network,Task Context-Related Module,discriminative context-related feature representation,Prototype Reset module,representative class prototype,classification benchmarks
AI 理解论文
溯源树
样例
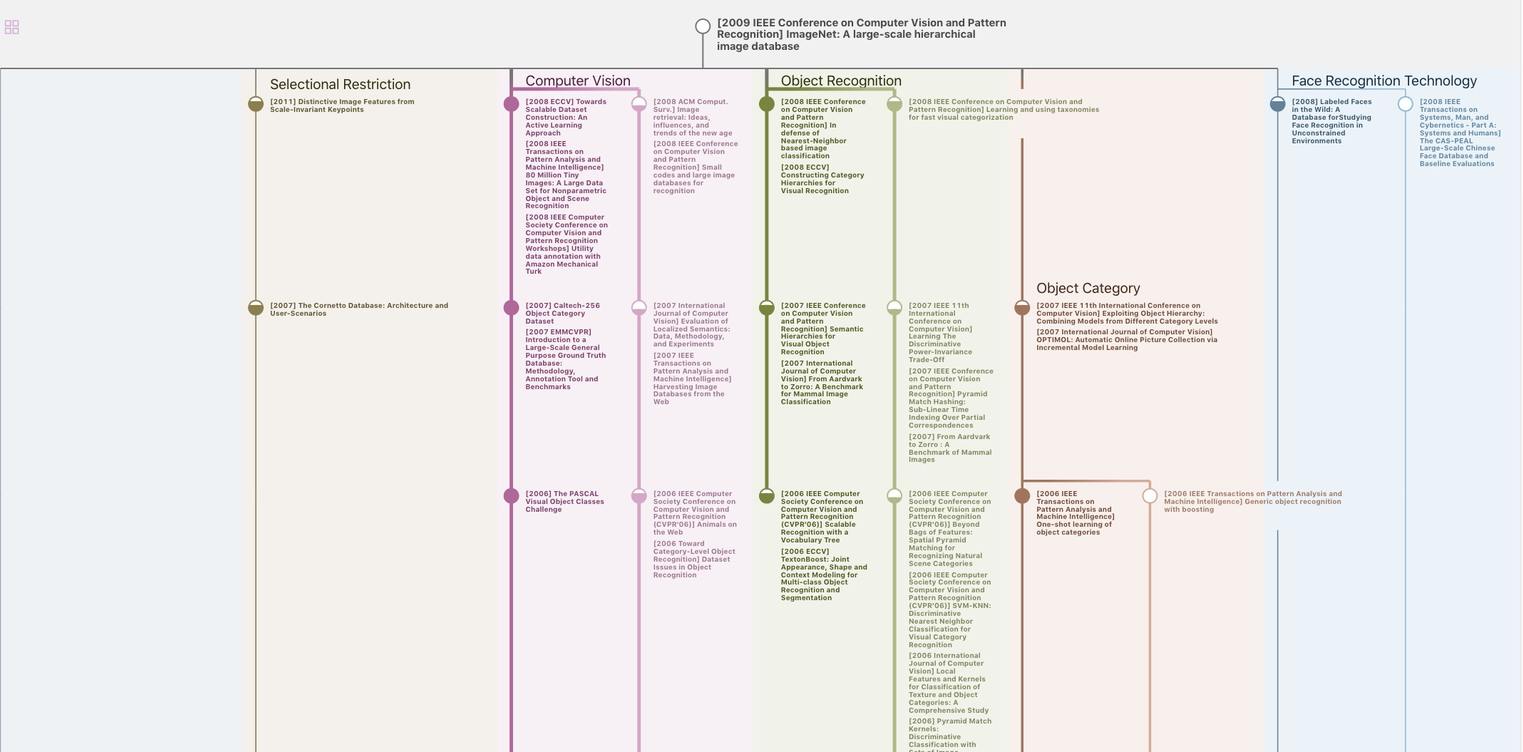
生成溯源树,研究论文发展脉络
Chat Paper
正在生成论文摘要