PTWA: Pre-training with Word Attention for Chinese Named Entity Recognition
2021 INTERNATIONAL JOINT CONFERENCE ON NEURAL NETWORKS (IJCNN)(2021)
摘要
Recently, the character-based model that incorporates potential word information has proven effective for Chinese named entity recognition (NER). However, due to the independence of the pre-trained character model and the lexicon, it will cause the embedding space to be misaligned and cannot be combined well. Chinese pre-trained encoders usually process text as characters. It ignores the information carried by the larger granular information, so the encoder cannot easily adapt to certain character combinations. Because large-grained information is ignored and Chinese does not have clear character boundaries, this will lead to the loss of important semantic information, which is an important problem for Chinese. In this paper, we propose PTWA: pre-training with word attention for Chinese named entity recognition. PTWA uses multi-head word attention to form a word vector from multiple word vectors, and proposes a word length prediction task to better integrate the word vector into pre-training. With the powerful capabilities of the transformer, PTWA can explicitly make full use of potential word information without adding an external lexicon, and can coexist with pre-trained models that implicitly use word information (such as BERT-WWM, and ERNIE). Experiments conducted on four Chinese NER datasets show that the performance of PTWA is better than other word-word models and Chinese pre-training models.
更多查看译文
关键词
Named Entity Recognition, Pre-training, Attention Mechanism
AI 理解论文
溯源树
样例
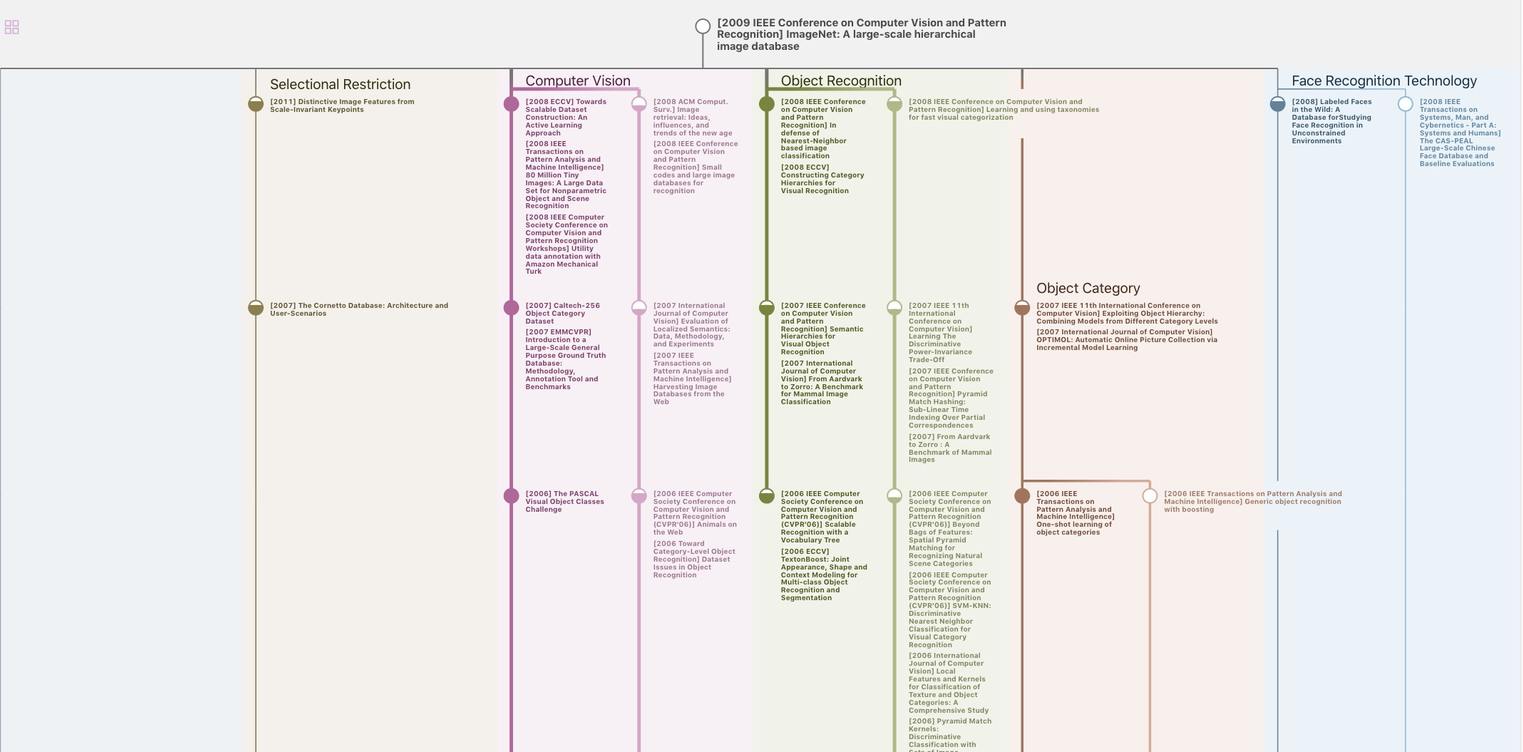
生成溯源树,研究论文发展脉络
Chat Paper
正在生成论文摘要