Predicting Conversation Outcomes Using Multimodal Transformer
2021 INTERNATIONAL JOINT CONFERENCE ON NEURAL NETWORKS (IJCNN)(2021)
摘要
Analysis of communication effectiveness is an important task for understanding business outcomes. Prior research has shown that voice data can be used to predict communication effectiveness. However, to our knowledge, no existing studies have used both vocal and verbal cues to predict conversation outcomes in naturally occurring, dyadic business interactions. We use recorded audio calls collected from a partnering Fortune 500 firm that captures conversations between inside salespeople and business customers. Analysis of communication effectiveness is accomplished by transcribing these audio files and subsequently segmenting each conversation into customer and salesperson speaker turns to enable extraction of audio features and text embeddings for each speaker turn. All the speaker turns from the same conversation can be treated as a time series data, which can be modeled by temporal models, like LSTM or transformers. In this paper we propose that a multimodal transformer network (MTN) can capture the importance of different speaker turns and can be used to effectively predict the outcome of the call using both audio and text features. Results from the proposed model outperform current state-of-the-art results and reveal that text features offer superior outcome prediction compared to audio features.
更多查看译文
关键词
conversation, communication, multimodal, self-attention, transformer, sentiment analysis
AI 理解论文
溯源树
样例
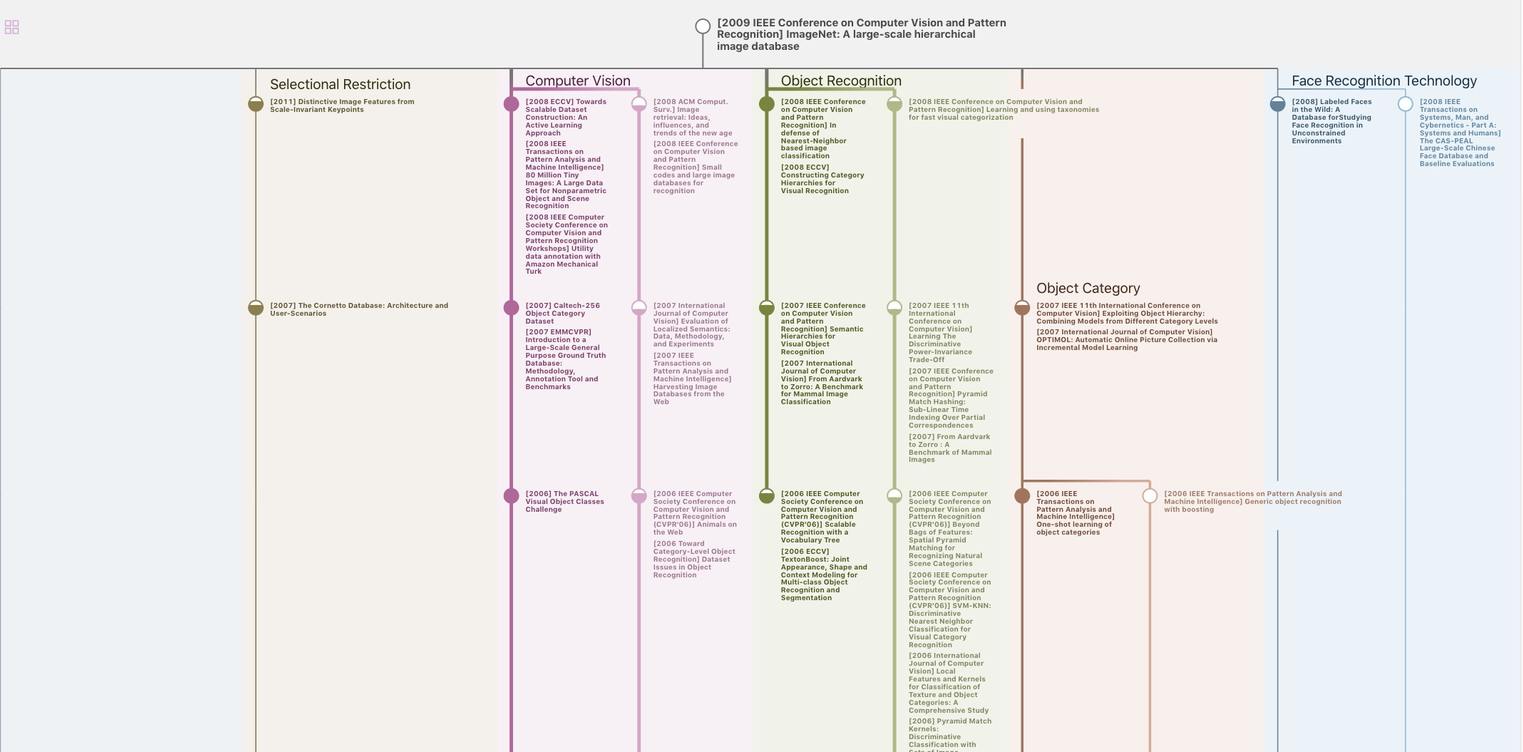
生成溯源树,研究论文发展脉络
Chat Paper
正在生成论文摘要