Learn and Visually Explain Deep Fair Models: an Application to Face Recognition
2021 INTERNATIONAL JOINT CONFERENCE ON NEURAL NETWORKS (IJCNN)(2021)
摘要
Trustworthiness, and in particular Algorithmic Fairness, is emerging as one of the most trending topics in Machine Learning (ML). In fact, ML is now ubiquitous in decision making scenarios, highlighting the necessity of discovering and correcting unfair treatments of (historically discriminated) subgroups in the population (e.g., based on gender, ethnicity, political and sexual orientation). This necessity is even more compelling and challenging when unexplainable black-box Deep Neural Networks (DNN) are exploited. An emblematic example of this necessity is provided by the detected unfair behavior of the ML-based face recognition systems exploited by law enforcement agencies in the United States. To tackle these issues, we first propose different (un)fairness mitigation regularizers in the training process of DNNs. We then study where these regularizers should be applied to make them as effective as possible. We finally measure, by means of different accuracy and fairness metrics and different visual explanation strategies, the ability of the resulting DNNs in learning the desired task while, simultaneously, behaving fairly. Results on the recent FairFace dataset prove the validity of our approach.
更多查看译文
关键词
Algorithmic Fairness, Fair Representation, Demographic Parity, Face Recognition, Deep Neural Network, Explainability, Visual Explanation, Attention Map, Dimensionality Reduction
AI 理解论文
溯源树
样例
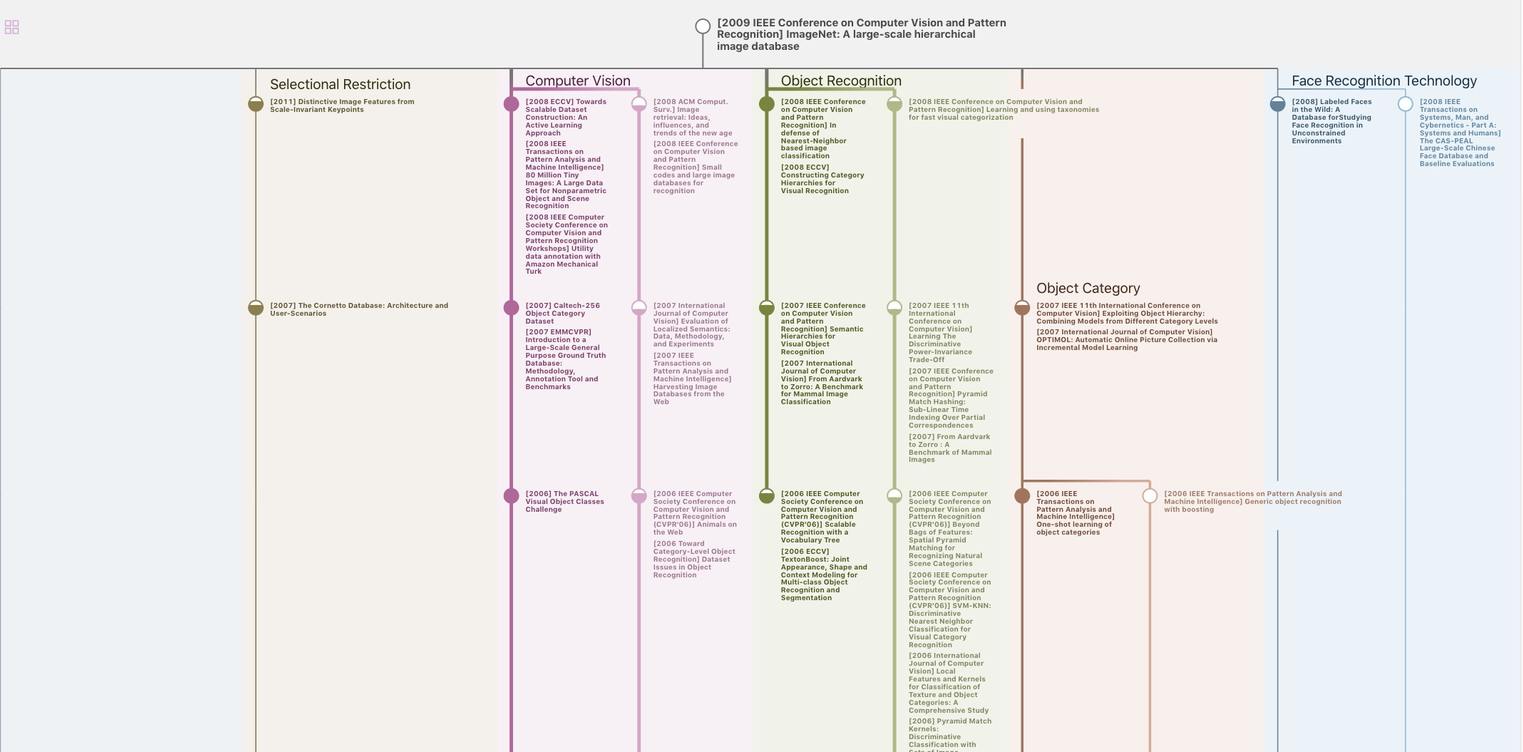
生成溯源树,研究论文发展脉络
Chat Paper
正在生成论文摘要