Trust, but Verify: Alleviating Pessimistic Errors in Model-Based Exploration
2021 INTERNATIONAL JOINT CONFERENCE ON NEURAL NETWORKS (IJCNN)(2021)
摘要
We propose trust-but-verify (TBV) mechanism, a new method which uses model uncertainty estimates to guide exploration. The mechanism augments graph search planning algorithms with the capacity to deal with learned model's imperfections. We identify certain type of frequent model errors, which we dub false loops, and which are particularly dangerous for graph search algorithms in discrete environments. These errors impose falsely pessimistic expectations and thus hinder exploration. We confirm this experimentally and show that TBV can effectively alleviate them. TBV combined with MCTS or Best First Search forms an effective model-based reinforcement learning solution, which is able to robustly solve sparse reward problems.
更多查看译文
关键词
model-based, exploration, reinforcement learning
AI 理解论文
溯源树
样例
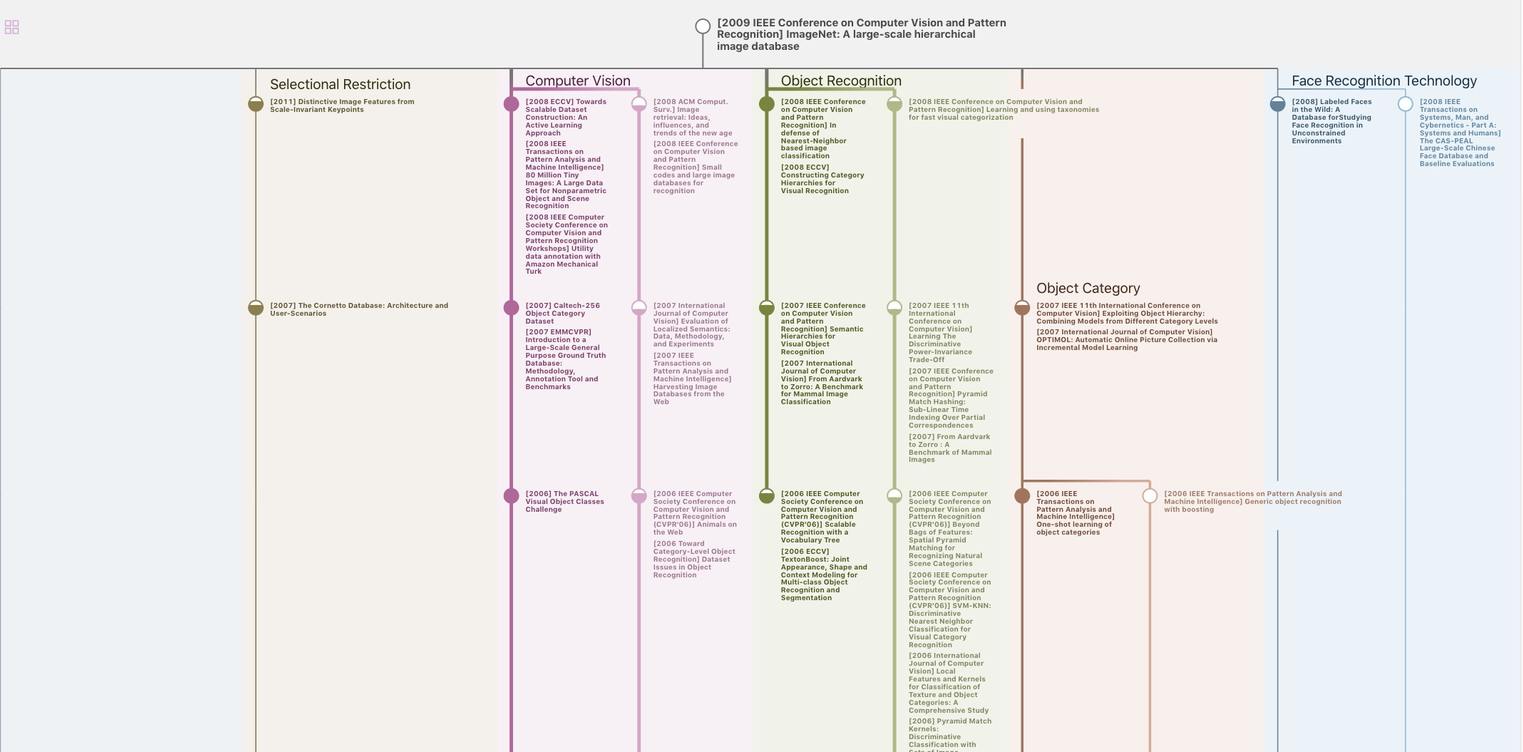
生成溯源树,研究论文发展脉络
Chat Paper
正在生成论文摘要